Motion-Guided Physics-Based Learning for Cardiac MRI Reconstruction.
ACSCC(2021)
摘要
In this work, we propose a robust learning-based cardiac motion estimation framework, to estimate non-rigid cardiac motion fields from undersampled cardiac data. Our proposed frameworks leverages the advantages of a lightweight motion estimation network and a combination of photometric and smoothness losses. This framework enables the prediction of cardiac motion fields to further improve on the downstream task of motion-compensated image reconstruction. We evaluate our motion estimation framework qualitatively and quantitatively on 41 in-house acquired 2D cardiac CINE MRIs. Our proposed method provides quantitatively competitive results to state-of-the art methods in motion estimation, and superior results in image reconstruction in terms of structural similarity metric and peak-signal-to-noise ratio. Furthermore, our frameworks allows for ~3500x faster motion estimation compared to state-of-the-art approaches, opening up the practical application potential for motion-guided physics-based image reconstruction.
更多查看译文
关键词
magnetic resonance imaging,motion estimation,image reconstruction,deep learning,neural networks,cardiac
AI 理解论文
溯源树
样例
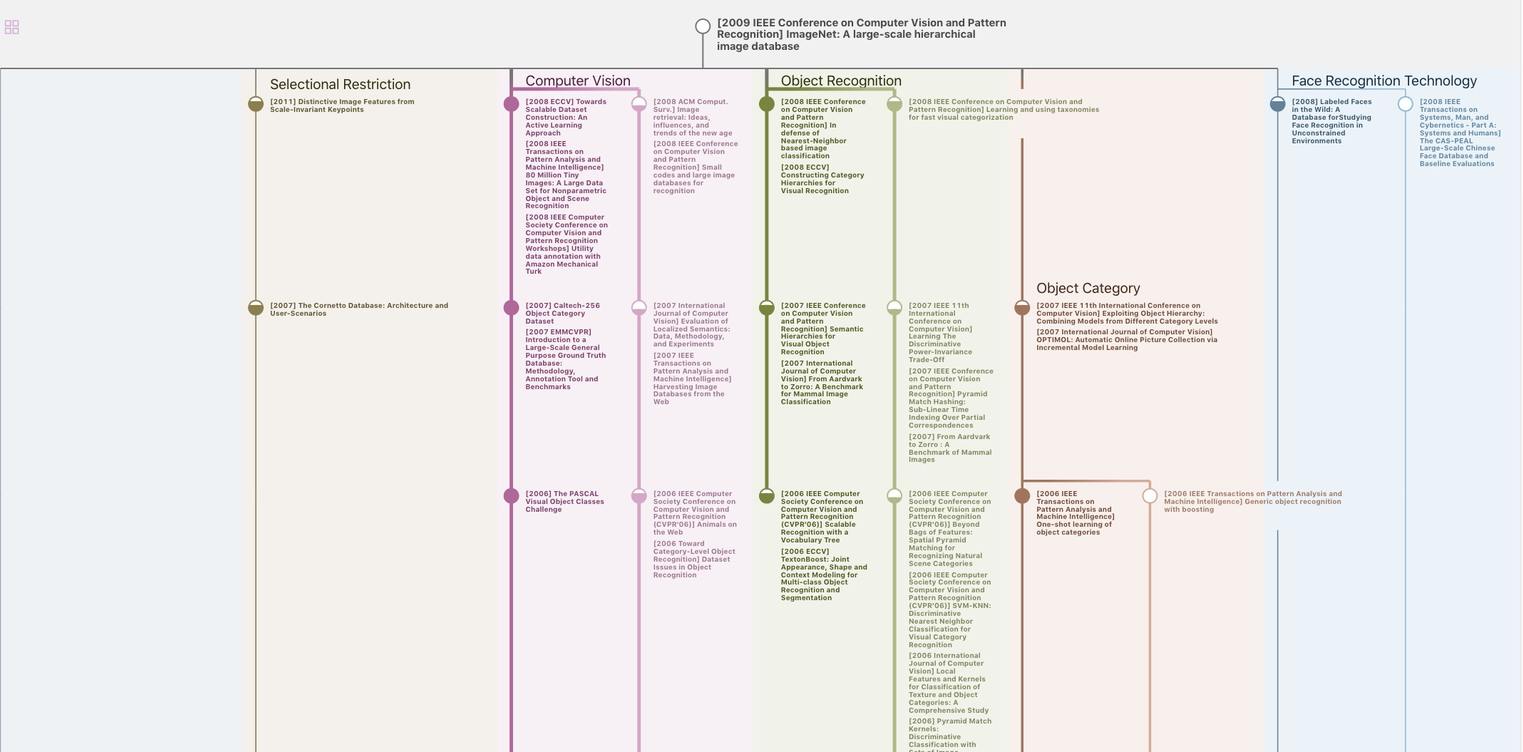
生成溯源树,研究论文发展脉络
Chat Paper
正在生成论文摘要