Robust Low-Rank Tensor Recovery From Quantized and Corrupted Measurements.
ACSCC(2021)
摘要
Real-world datasets are commonly represented by higher-order tensors, and easily suffer from quantized and corrupted errors. This paper, for the first time, studies the tensor recovery from quantized and corrupted measurements. A maximum likelihood approach under the exact low-Tucker- rank constraint is proposed to estimate the actual tensor. We provide both an upper bound and lower bound of the recovery error, and the theorems indicate that our method is order-wise optimal when the rank of the tensor is small. We also show that the error decays in the same order as the state-of-the-art method when there is no corruption. An efficient proximal gradient-based solver is proposed to recover the tensor. Experiments on both synthetic data and a public video dataset validate the effectiveness of our method.
更多查看译文
关键词
robust tensor recovery,higher-order tensor,quantization,low-rank,Tucker rank
AI 理解论文
溯源树
样例
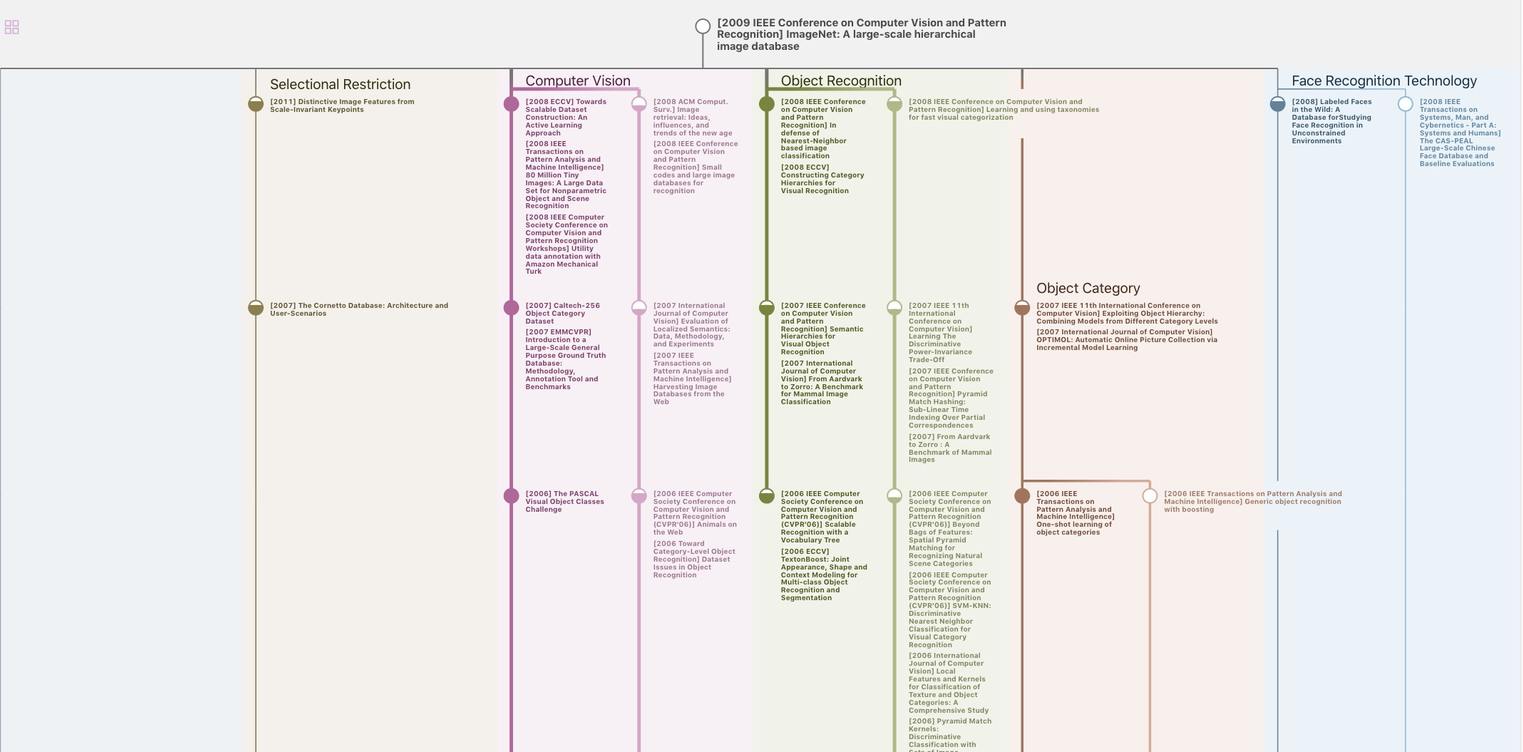
生成溯源树,研究论文发展脉络
Chat Paper
正在生成论文摘要