Partitioning and Gaussian Processes for Accelerating Sampling in Monte Carlo Tree Search for Continuous Decisions.
WSC(2021)
Abstract
We propose Part-MCTS for sampling continuous decisions at each stage of a Monte Carlo Tree Search algorithm. At each MCTS stage, Part-MCTS sequentially partitions the decision space and keeps a collection of Gaussian processes to describe the landscape of the objective function. A classification criteria based on the estimation of the minimum allows us to focus the attention on regions with better predicted behavior, reducing the evaluation effort elsewhere. Within each subregion, we can use any sampling distribution, and we propose to sample using Bayesian optimization. We compare our approach to KR-UCT (Yee et al. 2016) as state of the art competitor. Part-MCTS achieves better accuracy over a set of nonlinear test functions, and it has the ability to identify multiple promising solutions in a single run. This can be important when multiple solutions from a stage can be preserved and expanded at subsequent stages.
MoreTranslated text
Key words
sampling distribution,Bayesian optimization,nonlinear test functions,sampling continuous decisions,Monte Carlo tree search algorithm,Gaussian processes,MCTS sequentially partitions,KR-UCT
AI Read Science
Must-Reading Tree
Example
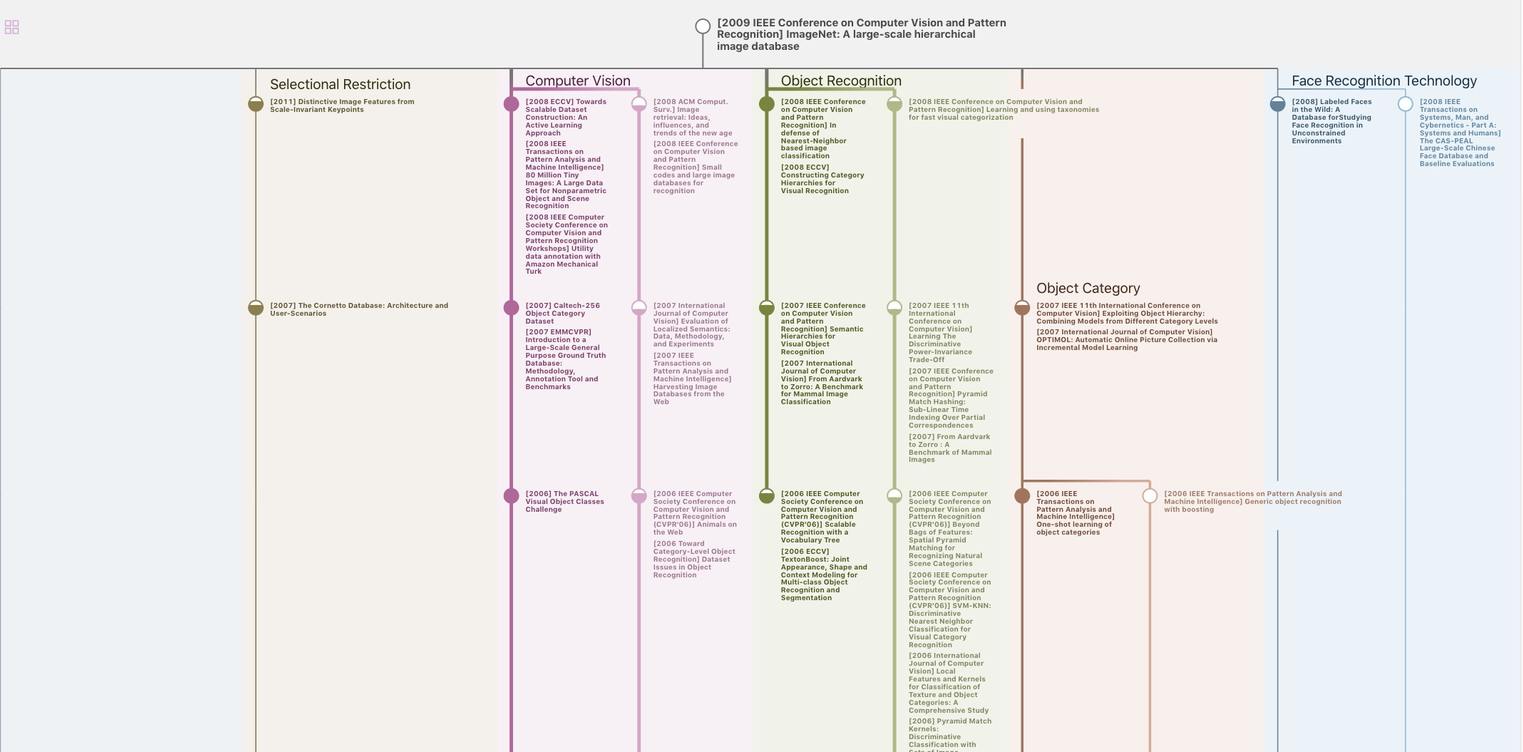
Generate MRT to find the research sequence of this paper
Chat Paper
Summary is being generated by the instructions you defined