Optimal maintenance scheduling under uncertainties using Linear Programming-enhanced Reinforcement Learning
Engineering Applications of Artificial Intelligence(2022)
摘要
Maintenance is of great importance for the safety and integrity of infrastructures. The expected optimal maintenance policy in this study should be able to minimize system maintenance cost while satisfying the system reliability requirements. Stochastic maintenance scheduling with an infinite horizon has not been investigated thoroughly in the literature. In this work, we formulate the maintenance optimization under uncertainties as a Markov Decision Process (MDP) problem and solve it using a modified Reinforcement Learning method. A Linear Programming-enhanced RollouT (LPRT) is proposed, which considers both constrained deterministic and stochastic maintenance scheduling with an infinite horizon. The novelty of the proposed approach is that it is suitable for online maintenance scheduling, which can include random unexpected maintenance performance and system degradation. The proposed method is demonstrated with numerical examples and compared with several existing methods. Results show that LPRT is able to determine the suitable optimal maintenance policy efficiently compared with existing methods with similar accuracy. Parametric studies are used to investigate the effect of uncertainty, subproblem size, and the number of stochastic stages on the final maintenance cost. Limitations and future work are given based on the proposed study.
更多查看译文
关键词
Maintenance scheduling,Rollout,Linear programming,Infinite horizon,Stochastic maintenance
AI 理解论文
溯源树
样例
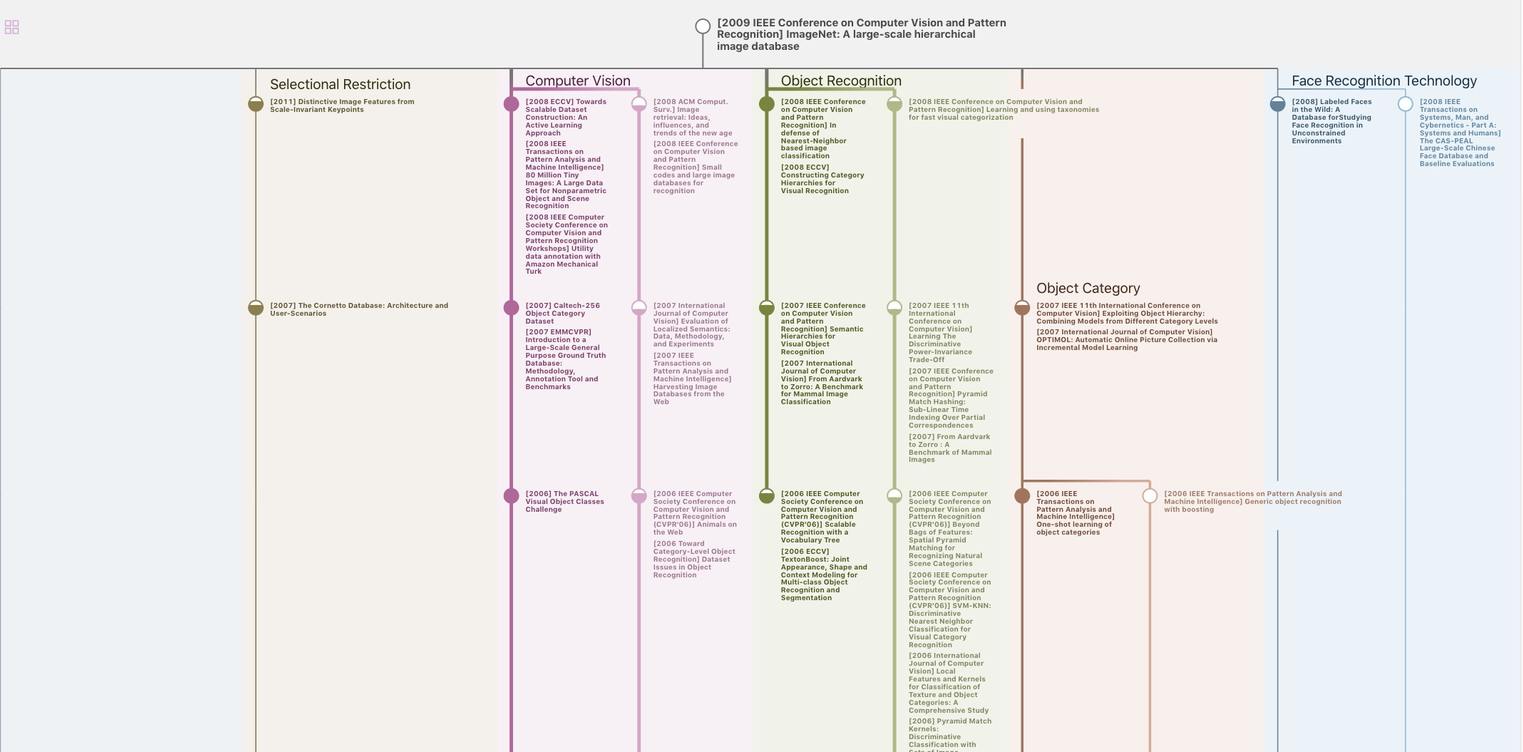
生成溯源树,研究论文发展脉络
Chat Paper
正在生成论文摘要