Tiny FCOS: a Lightweight Anchor-Free Object Detection Algorithm for Mobile Scenarios
MOBILE NETWORKS & APPLICATIONS(2021)
摘要
Many mobile vision application scenarios require the real-time detection of objects, such as real-world road condition detection. The real-time object detection demands a lightweight of the model, which is the ability to real-time process the detection of object efficiently. Recent studies have shown the potential of FCOS with anchor-free to increase the detection capacity. However, there are several severe issues with FCOS that prevent it from being directly employed to real-time object detection, such as algorithm model’s complexity, larger computation of parameter, and high memory usage. To address these issues, we design a lightweight FCOS-based model for real-time object detection, named Tiny FCOS, with three distinction characteristics: (1) a lightweight backbone network, which achieves the abatement of model’s weight efficiently. (2) a standardized dilated convolution group to construct the structure of FPN and efficiently reduces the impact of the gridding effect. (3) the prediction branch of FCN, while conceptually simple, detect object at only one convolution block rather than stacking convolutions with kernel size of 3 × 3 by 4 times. Experiments on two datasets demonstrate that the mAP of Tiny FCOS reaches 62.4% improving 4% compared to Tiny YOLOv3 on dataset PASCAL VOC, while the amount of parameters and calculations fall down to only 27.8% and 20.3% of FCOS respectively and the speed is 6 times that of FCOS on dataset KITTI. Furthermore, Tiny FCOS significantly outperforms existing methods and provides a new solution to the real-time object detection problems.
更多查看译文
关键词
Mobile vision, Deep learning, Object detection, Lightweight, Anchor-free
AI 理解论文
溯源树
样例
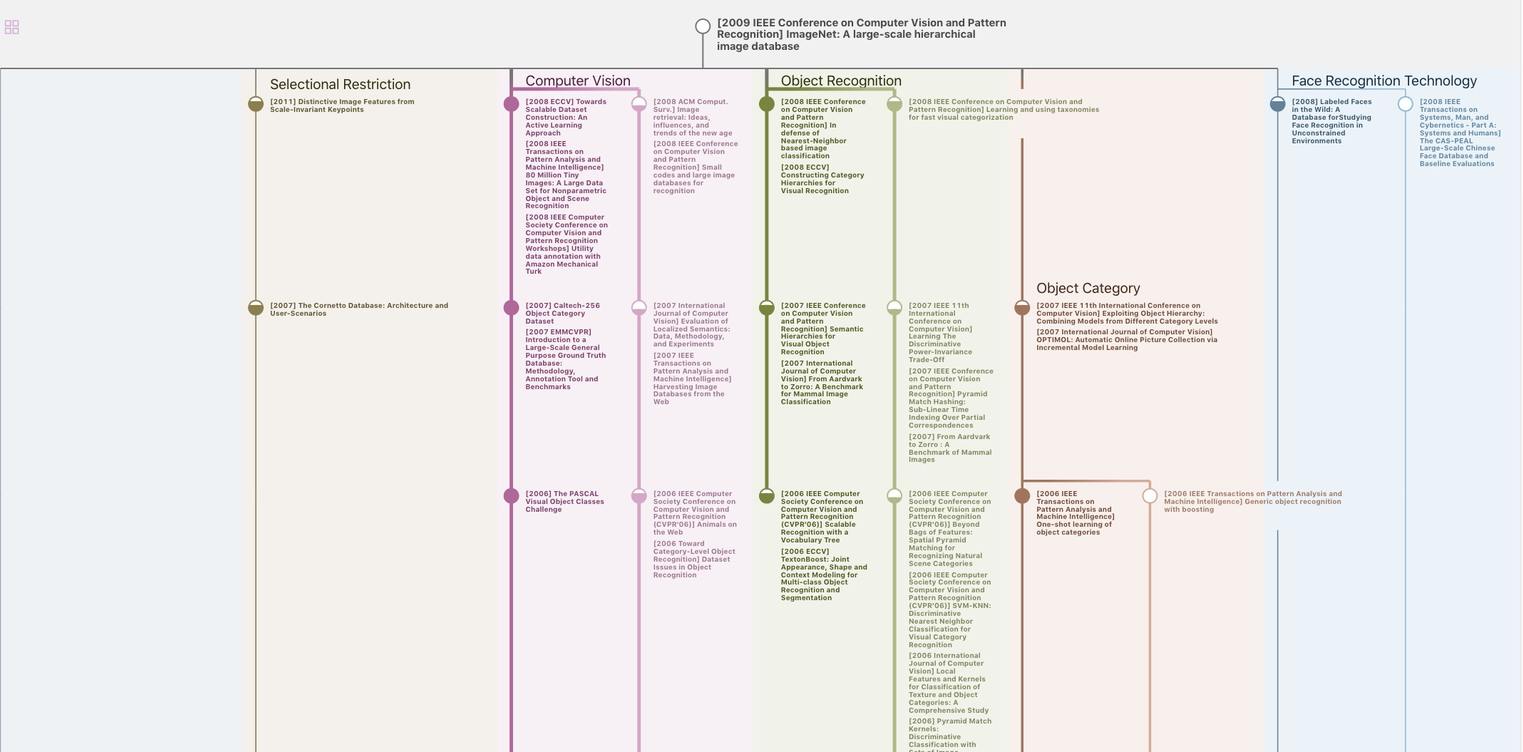
生成溯源树,研究论文发展脉络
Chat Paper
正在生成论文摘要