PulseNet: A multitask learning network for remote heart rate estimation
Knowledge-Based Systems(2022)
摘要
Photoplethysmography imaging (PPGI) is a heart rate (HR) estimation technology based on facial videos that is widely used in scenes where traditional equipment is inconvenient to use. However, the energy of the PPGI signal is weak and easily interferes with by external conditions, such as changes in illumination and head movement, resulting in the lack of robustness of traditional PPGI extraction methods in natural scenes. To address these challenges, we construct an end-to-end multitask model called PulseNet based on spatiotemporal convolution. PulseNet combines skin segmentation and attention mechanisms to suppress background noise and estimates heart rate through mutual constraints between PPGI signals and average heart rate values. We first detect faces from the video and adjust the facial sequence to the same size. Then, the facial sequence is taken as input, and the skin-based attention mechanism is used to train the model. The skin confidence and PPGI feature maps are used to assign different weights to the face regions to extract physiological features. The final physiological features are used for average HR prediction and PPGI signal regression. The results of intradatabase testing and cross-database testing on four public datasets show that the experimental results of PulseNet are better than the comparison methods.
更多查看译文
关键词
End-to-end learning,Heart rate estimation,Photoplethysmography imaging,Remote photoplethysmography,Spatiotemporal convolution
AI 理解论文
溯源树
样例
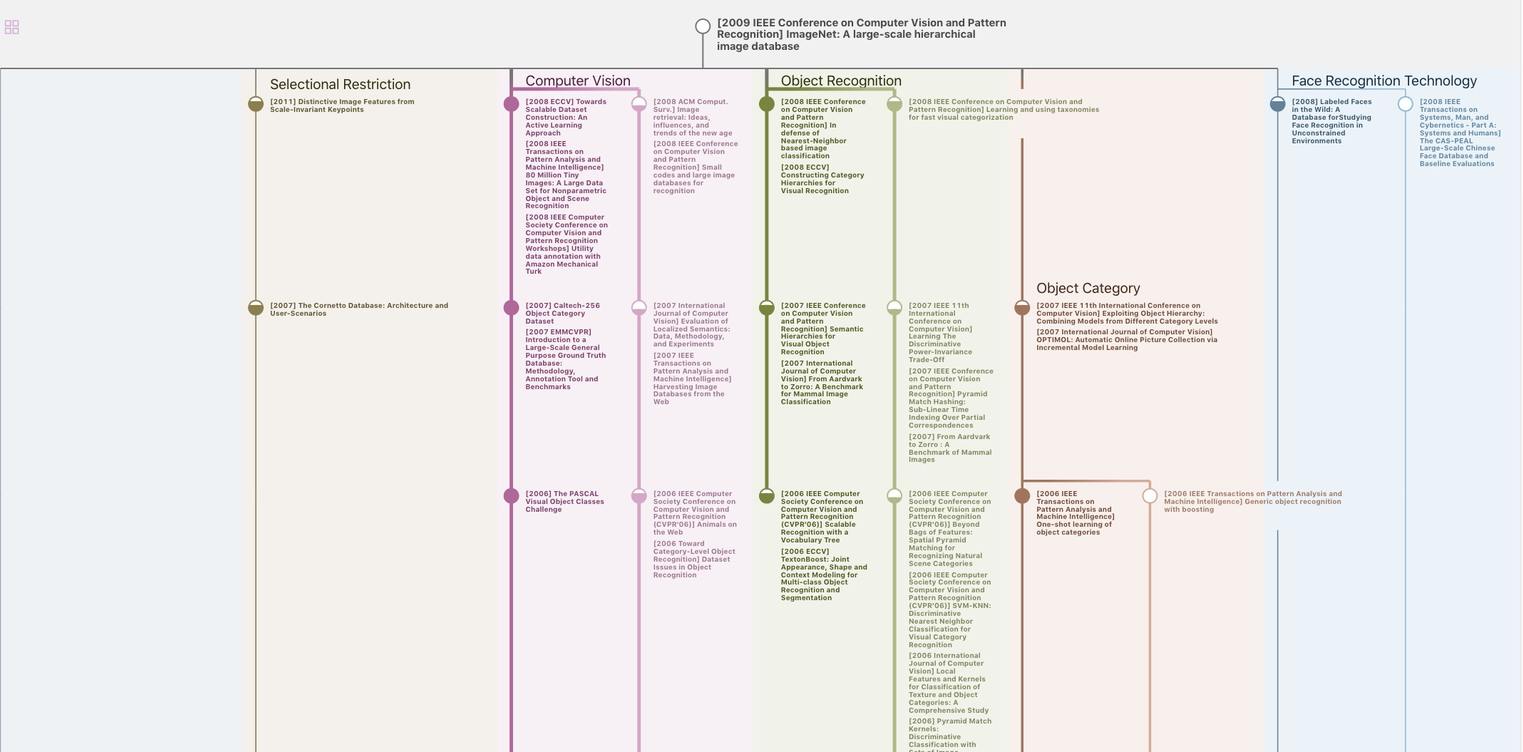
生成溯源树,研究论文发展脉络
Chat Paper
正在生成论文摘要