Computationally Efficient Maximum Likelihood Channel Estimation for Coarsely Quantized Massive MIMO Systems
IEEE Communications Letters(2022)
摘要
We consider computationally efficient maximum likelihood (ML) channel estimation for massive multiple-input multiple-output (MIMO) systems using coarsely quantized measurements obtained from low-resolution analog-to-digital converters (ADCs) at the receivers. We first devise a computationally efficient ML estimator (referred to as CQML) by using the cyclic optimization and majorization-minimization (MM) techniques. Then, we show the connections between CQML and the conventional unquantized ML estimator. Numerical examples are provided to demonstrate the effectiveness and computational efficiency of the proposed channel estimation algorithm.
更多查看译文
关键词
Low-resolution analog-to-digital converters (ADCs),massive multiple-input multiple-output (MIMO) communications,maximum likelihood (ML) channel estimation,majorization-minimization (MM)
AI 理解论文
溯源树
样例
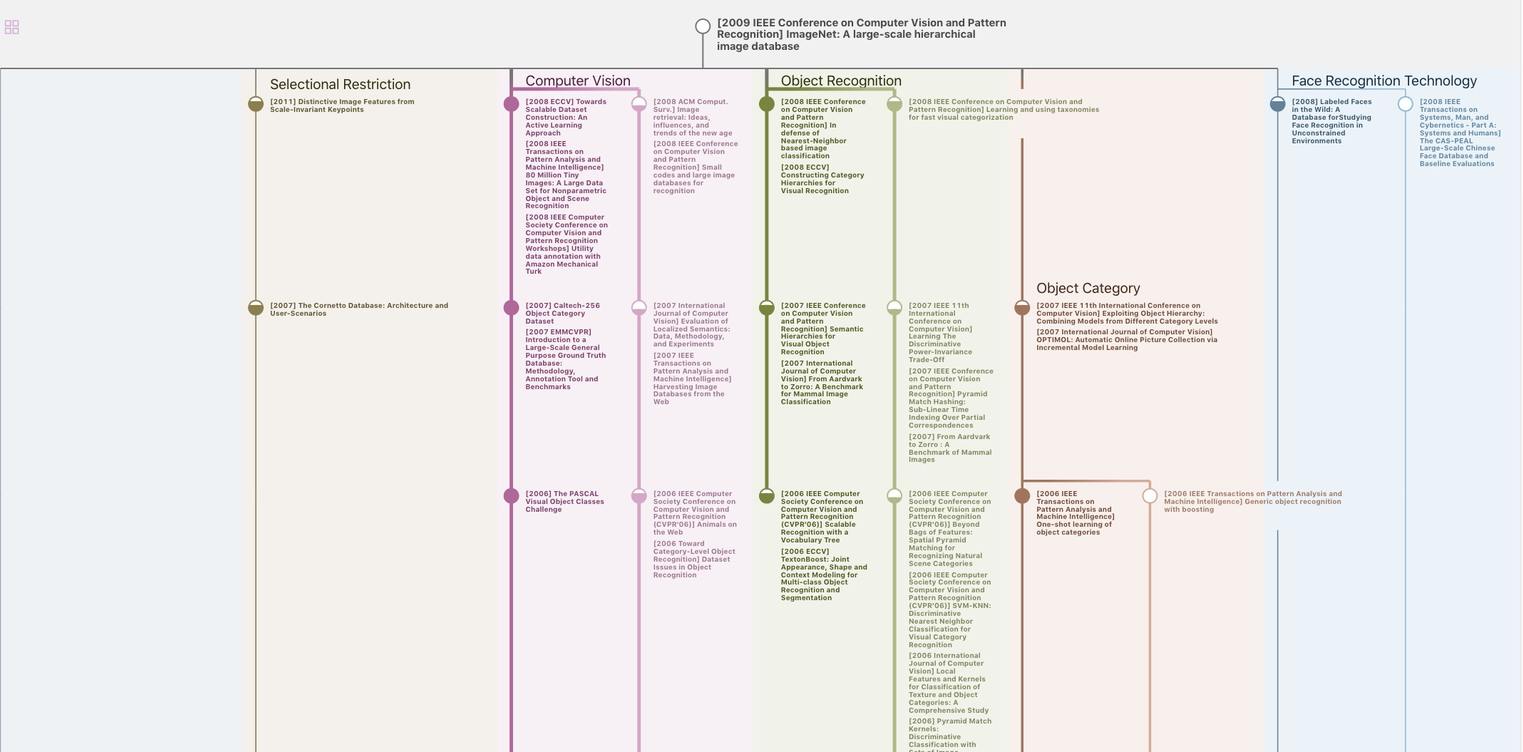
生成溯源树,研究论文发展脉络
Chat Paper
正在生成论文摘要