Subspace-based self-weighted multiview fusion for instance retrieval
Information Sciences(2022)
摘要
Most existing subspace-based multiview learning algorithms treat multiview features equally in the process of subspace reconstruction without distinguishing the discrepant contributions from different views. This limitation adversely affects subspace embedding, particularly when significant variances in view-specific feature characteristics are present. In this paper, a unified subspace-based self-weighted multiview learning framework (SSMVL) is proposed for instance retrieval. In contrast to the previous methods in which the variances in multiview features are ignored, the self-weighted learning mechanism is integrated into the multiview subspace learning framework such that the weights of different views are adaptively learned instead of empirically assigned. In addition, the proposed SSMVL approach falls into the unsupervised learning category and is thus independent of massive amounts of labeled data resulting from labor-intensive annotation. In this study, the extension of SSMVL to the Hamming subspace learning paradigm is also explored for efficient retrieval. Experiments on five public benchmarks reveal that the self-weighted learning strategy plays a beneficial role in multiview fusion, and our method achieves superior performance in comparison to state-of-the-art methods.
更多查看译文
关键词
Self-weighted learning,Multiview subspace learning,Multiview fusion,Instance retrieval
AI 理解论文
溯源树
样例
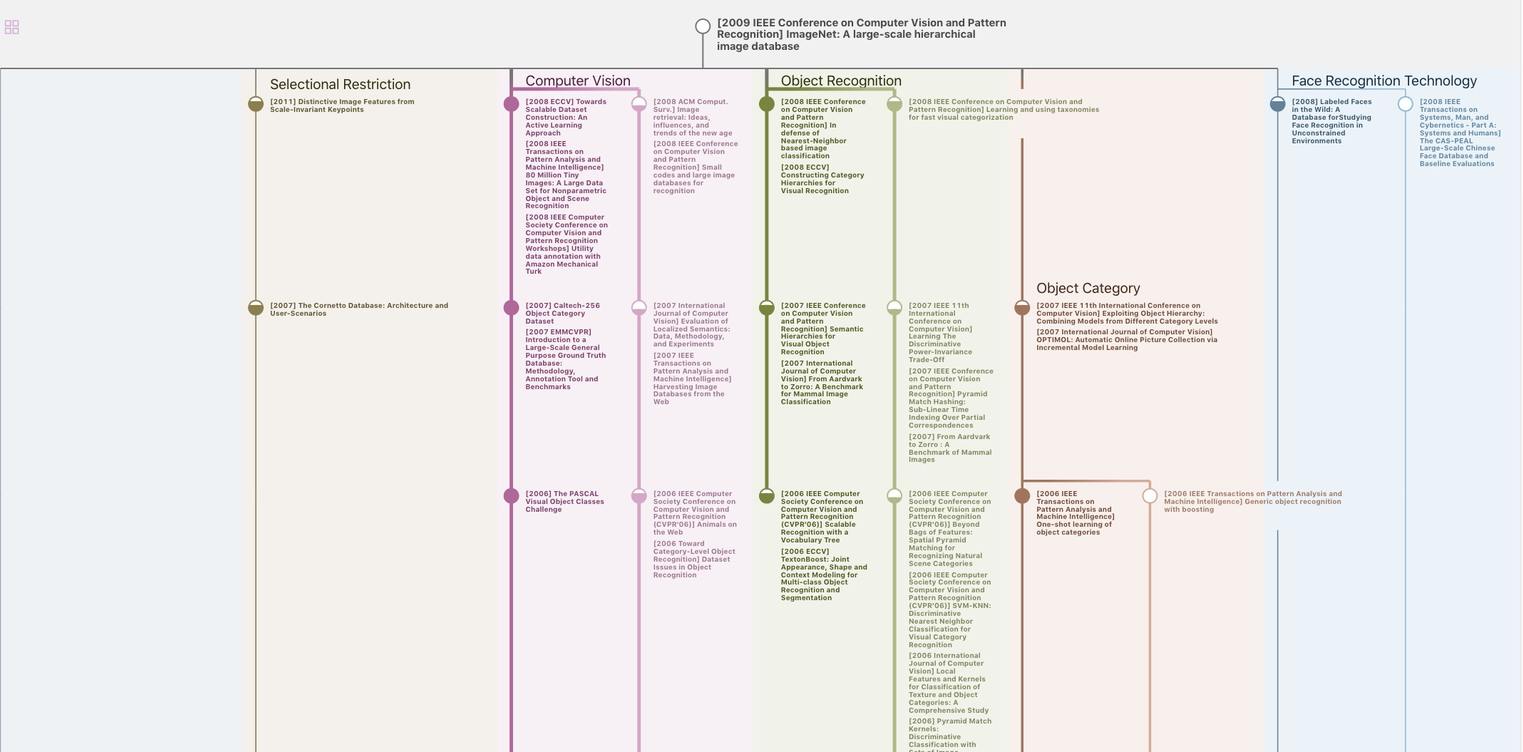
生成溯源树,研究论文发展脉络
Chat Paper
正在生成论文摘要