High-Resolution Iterative Feedback Network for Camouflaged Object Detection
AAAI 2023(2023)
Abstract
Spotting camouflaged objects that are visually assimilated into the background is tricky for both object detection algorithms and humans who are usually confused or cheated by the perfectly intrinsic similarities between the foreground objects and the background surroundings. To tackle this challenge, we aim to extract the high-resolution texture details to avoid the detail degradation that causes blurred vision in edges and boundaries. We introduce a novel HitNet to refine the low-resolution representations by high-resolution features in an iterative feedback manner, essentially a global loop-based connection among the multi-scale resolutions. To design better feedback feature flow and avoid the feature corruption caused by recurrent path, an iterative feedback strategy is proposed to impose more constraints on each feedback connection. Extensive experiments on four challenging datasets demonstrate that our HitNet breaks the performance bottleneck and achieves significant improvements compared with 29 state-of-the-art methods. In addition, to address the data scarcity in camouflaged scenarios, we provide an application example to convert the salient objects to camouflaged objects, thereby generating more camouflaged training samples from the diverse salient object datasets. Code will be made publicly available.
MoreTranslated text
Key words
CV: Scene Analysis & Understanding,CV: Object Detection & Categorization,CV: Segmentation
AI Read Science
Must-Reading Tree
Example
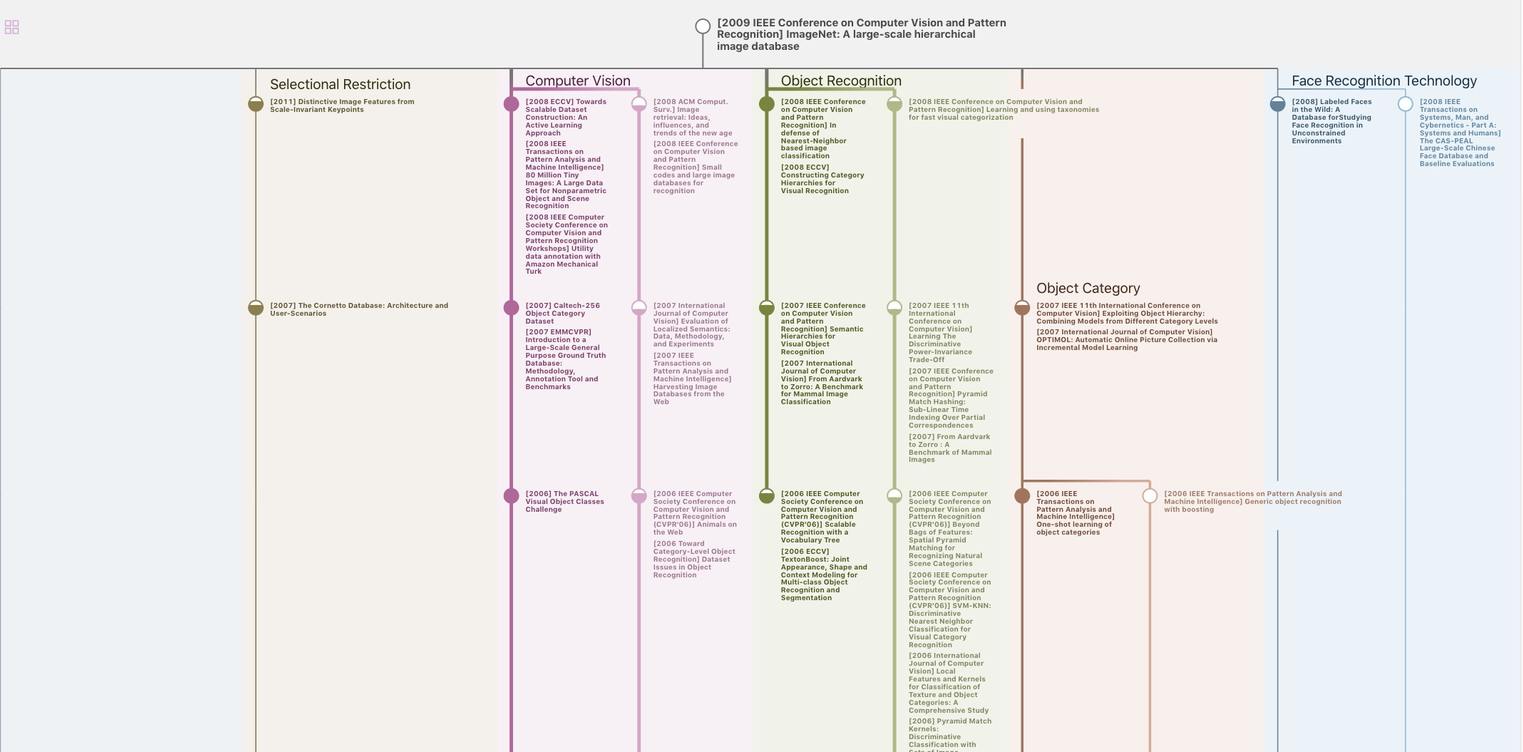
Generate MRT to find the research sequence of this paper
Chat Paper
Summary is being generated by the instructions you defined