Benchmarking Test-Time Unsupervised Deep Neural Network Adaptation on Edge Devices
2022 IEEE International Symposium on Performance Analysis of Systems and Software (ISPASS)(2022)
摘要
The prediction accuracy of deep neural networks (DNNs) after deployment at the edge can suffer with time due to shifts in the distribution of the new data. To improve robustness of DNNs, they must be able to update themselves. However, DNN adaptation at the edge is challenging due to lack of resources. Recently, lightweight prediction-time unsupervised DNN adaptation techniques have been introduced that improve prediction accuracy of the models for noisy data by re-tuning the batch normalization parameters. This paper performs a comprehensive measurement study of such techniques to quantify their performance and energy on various edge devices as well as find bottlenecks and propose optimization opportunities.
更多查看译文
关键词
edge devices,deep neural networks,DNNs,lightweight prediction-time unsupervised DNN adaptation,unsupervised deep neural network adaptation,batch normalization parameters
AI 理解论文
溯源树
样例
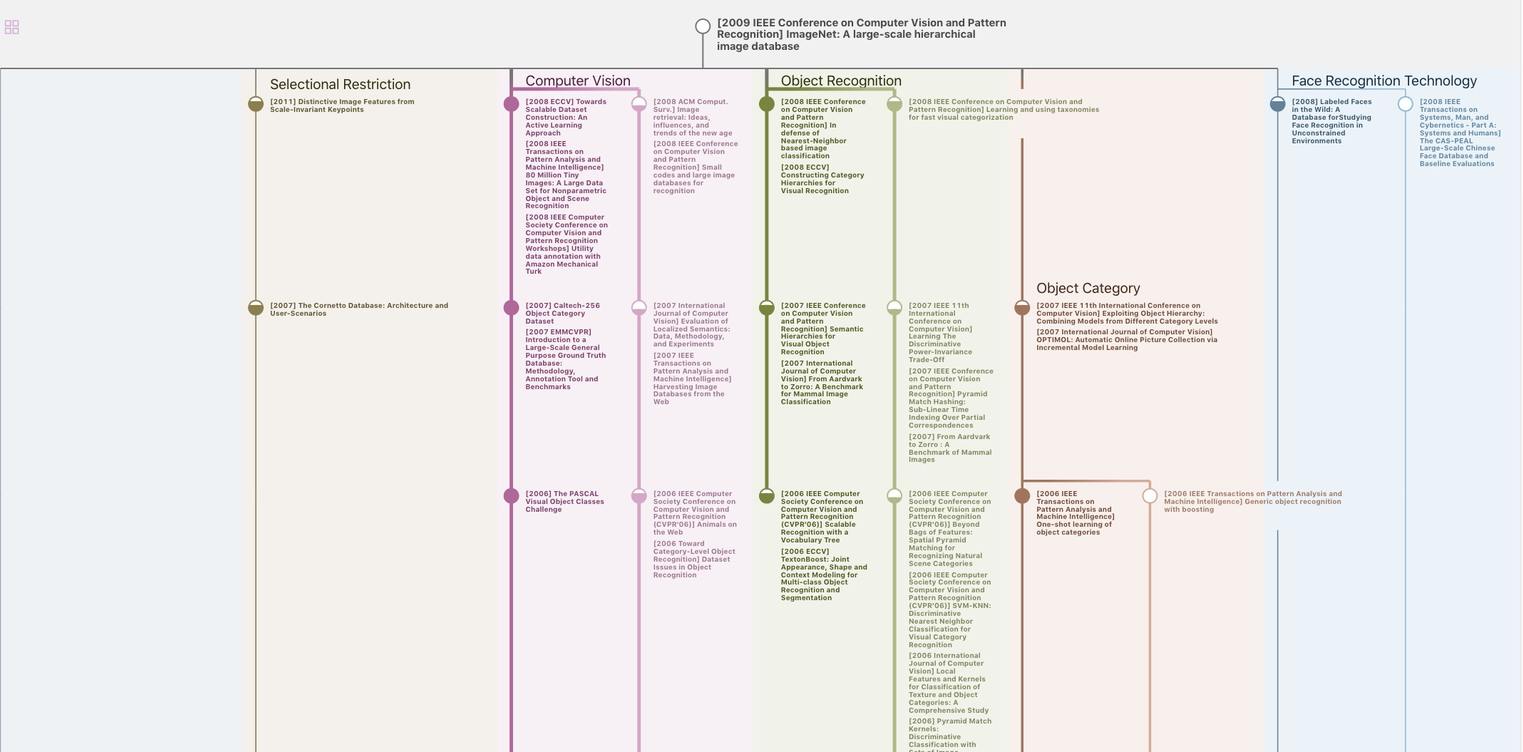
生成溯源树,研究论文发展脉络
Chat Paper
正在生成论文摘要