A computational model for individual differences in non-reinforced learning for individual items
bioRxiv (Cold Spring Harbor Laboratory)(2024)
摘要
Cue-Approach Training (CAT) is a paradigm that enhances preferences without external reinforcmeents, suggesting a potential role for internal learning processes. Here, we developed a novel Bayesian computational model to quantify anticipatory response patterns during the training phase of CAT. This phase includes individual items and thus this marker is potentially of internal learning signals at the item level. Our model, fitted to meta-analysis data from 29 prior CAT experiments, was able to predict individual differences in non-reinforced preference changes using a key computational marker. Crucially, two new experiments manipulated the training procedure to influence the model’s predicted learning marker. As predicted and preregistered, the manipulation successfully induced differential preference changes, supporting a causal role of our model. These findings demonstrate powerful potential of our computational framework for investigating intrinsic learning processes. This framework could be used to predict preference changes and opens new avenues for understanding intrinsic motivation and decision-making.
Teaser Bayesian modeling of response time predicts individual differences in non reinforced preference change.
### Competing Interest Statement
The authors have declared no competing interest.
更多查看译文
关键词
passive computational marker,individual differences,learning,non-reinforced
AI 理解论文
溯源树
样例
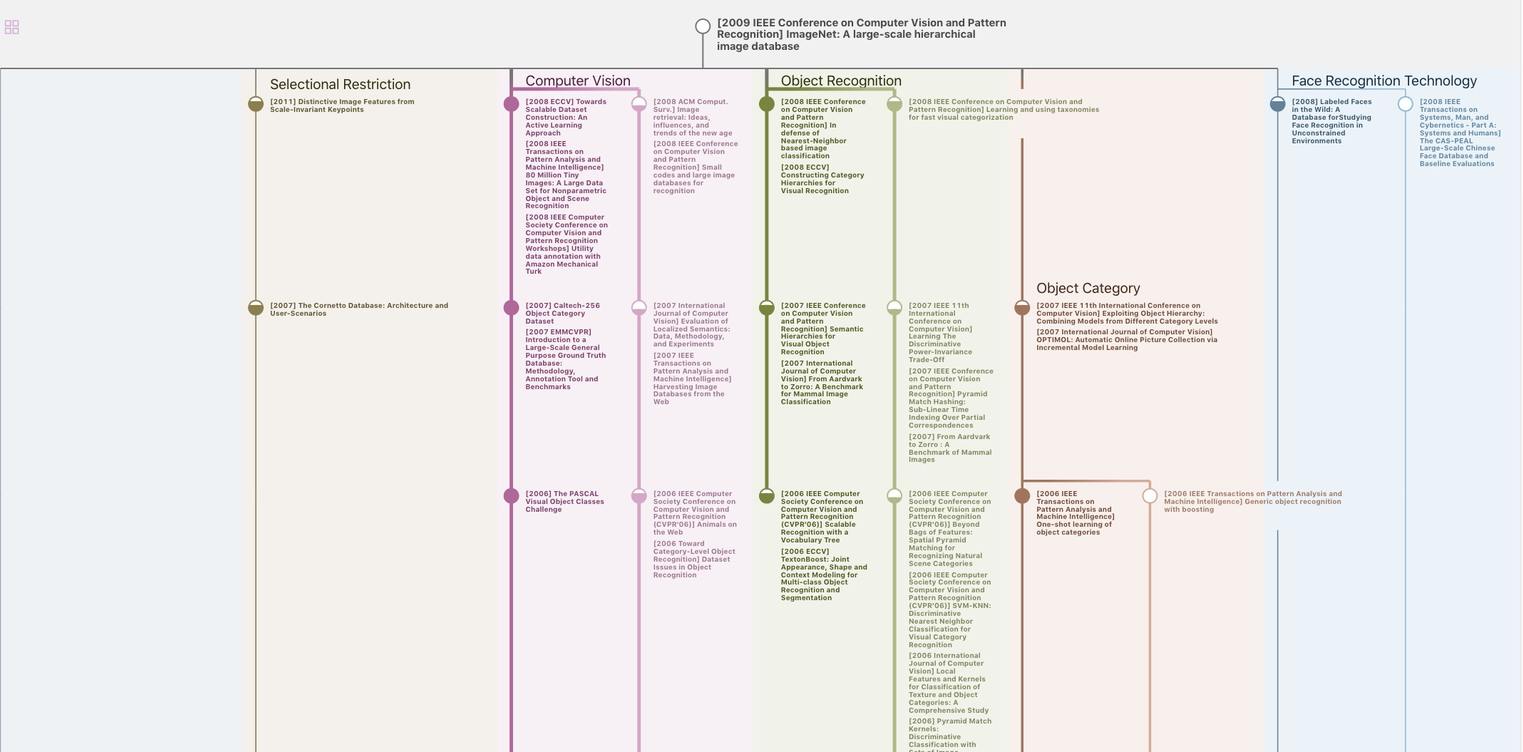
生成溯源树,研究论文发展脉络
Chat Paper
正在生成论文摘要