Self-supervised Correlation Learning for Cross-Modal Retrieval
user-5f163cde4c775ed682f575fe(2022)
摘要
Cross-modal retrieval aims to retrieve relevant data from another modality when given a query of one modality. Although most existing methods that rely on the label information of multimedia data have achieved promising results, the performance benefiting from labeled data comes at a high cost since labeling data often requires enormous labor resources, especially on large-scale multimedia datasets. Therefore, unsupervised cross-modal learning is of crucial importance in real-world applications. In this paper, we propose a novel unsupervised cross-modal retrieval method, named Self-supervised Correlation Learning (SCL), which takes full advantage of large amounts of unlabeled data to learn discriminative and modality-invariant representations. Since unsupervised learning lacks the supervision of category labels, we incorporate the knowledge from the input as a supervisory signal by maximizing the mutual information between the input and the output of different modality-specific projectors. Besides, for the purpose of learning discriminative representations, we exploit unsupervised contrastive learning to model the relationship among intra- and inter-modality instances, which makes similar samples closer and pushes dissimilar samples apart. Moreover, to further eliminate the modality gap, we use a weight-sharing scheme and minimize the modality-invariant loss in the joint representation space. Beyond that, we also extend the proposed method to the semi-supervised setting. Extensive experiments conducted on three widely-used benchmark datasets demonstrate that our method achieves competitive results compared with current state-of-the-art cross-modal retrieval approaches.
更多查看译文
关键词
Cross-modal retrieval,self-supervised contrastive learning,mutual information estimation
AI 理解论文
溯源树
样例
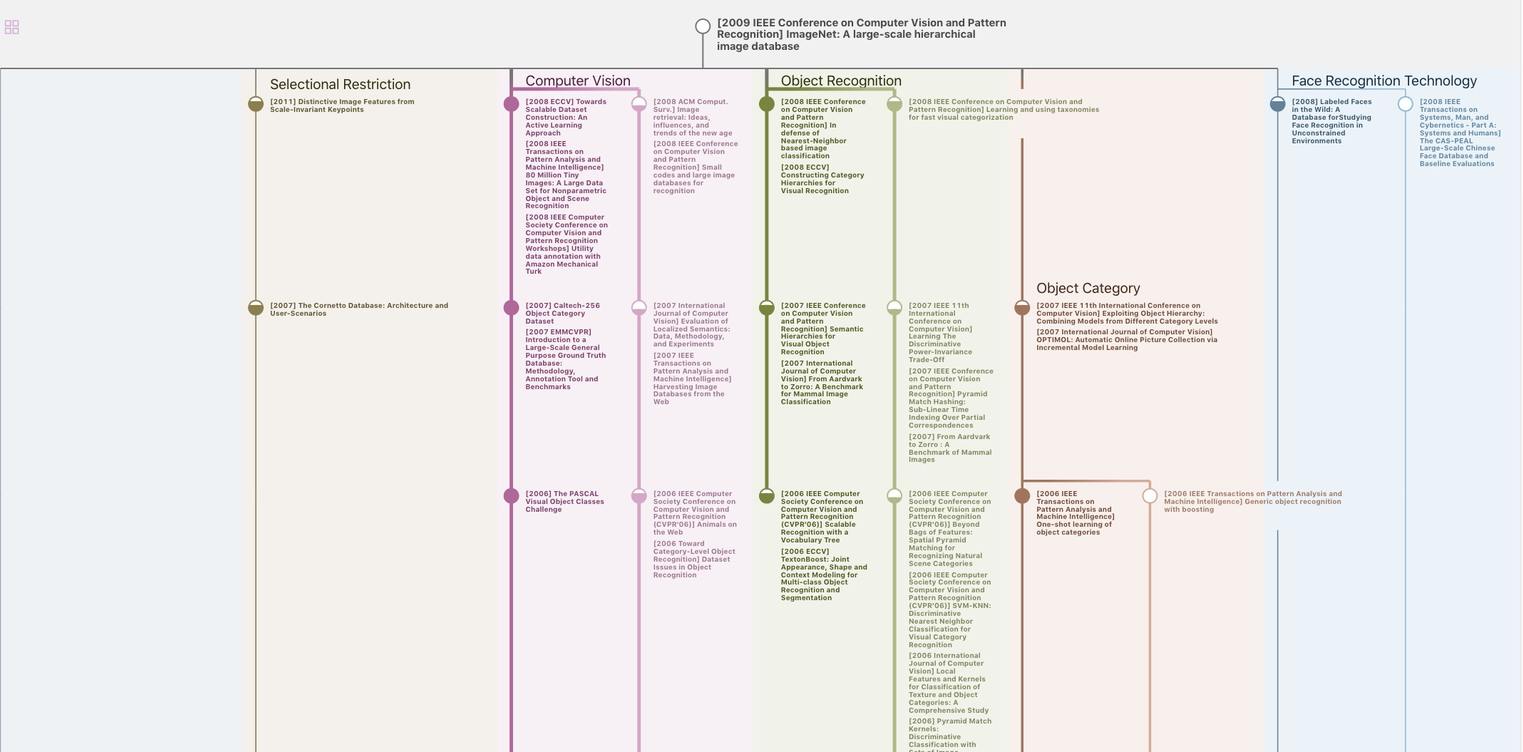
生成溯源树,研究论文发展脉络
Chat Paper
正在生成论文摘要