Depth Completion Using Geometry-Aware Embedding
IEEE International Conference on Robotics and Automation(2022)
摘要
Exploiting internal spatial geometric constraints of sparse LiDARs is beneficial to depth completion, however, has been not explored well. This paper proposes an efficient method to learn geometry-aware embedding, which encodes the local and global geometric structure information from 3D points, e.g., scene layout, object's sizes and shapes, to guide dense depth estimation. Specifically, we utilize the dynamic graph representation to model generalized geometric relationship from irregular point clouds in a flexible and efficient manner. Further, we joint this embedding and corresponded RGB appearance information to infer missing depths of the scene with well structure-preserved details. The key to our method is to integrate implicit 3D geometric representation into a 2D learning architecture, which leads to a better trade-off between the performance and efficiency. Extensive experiments demonstrate that the proposed method outperforms previous works and could reconstruct fine depths with crisp boundaries in regions that are over-smoothed by them. The ablation study gives more insights into our method that could achieve significant gains with a simple design, while having better generalization capability and stability. The code is available at https://github.com/Wenchao-Du/GAENet.
更多查看译文
关键词
depth completion,geometry-aware
AI 理解论文
溯源树
样例
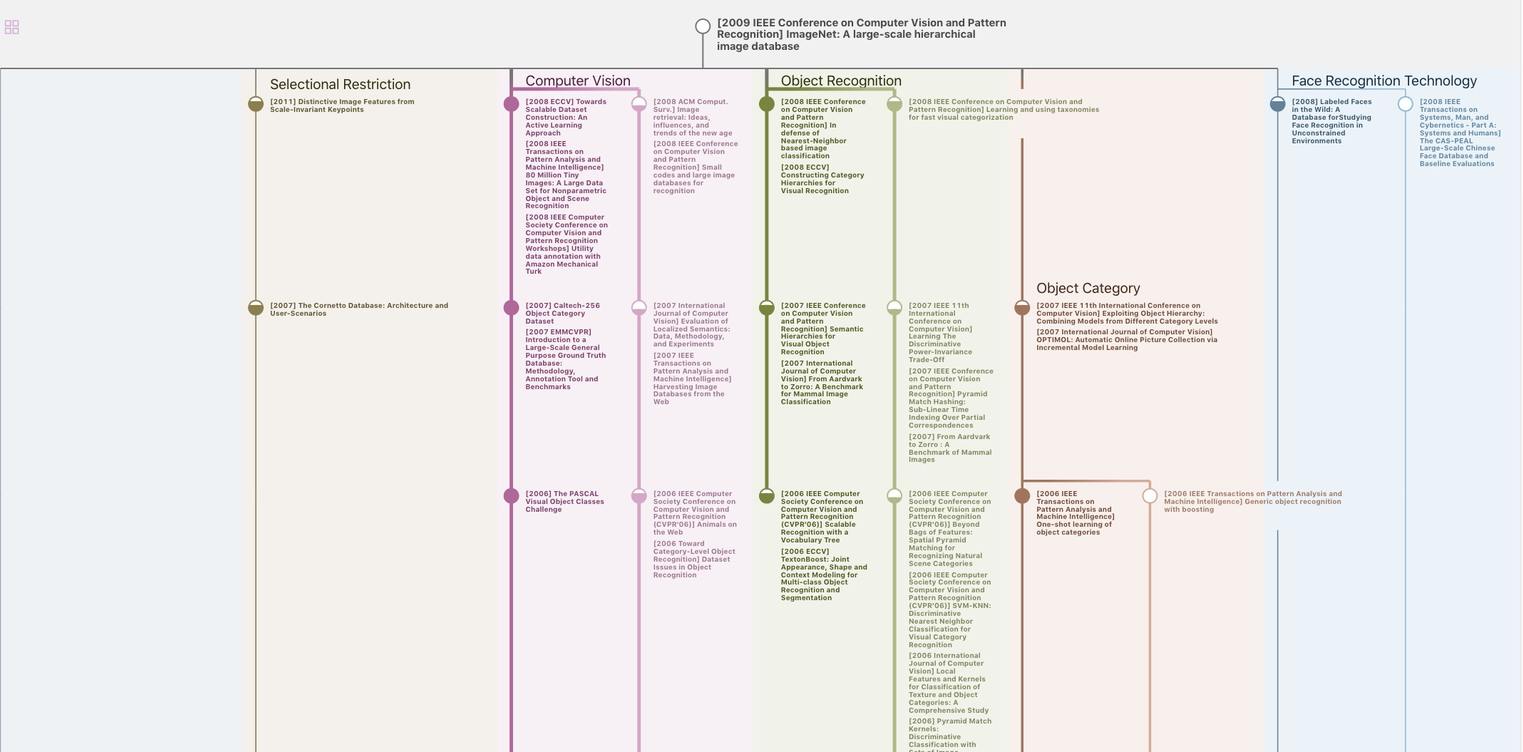
生成溯源树,研究论文发展脉络
Chat Paper
正在生成论文摘要