HIBRIDS: Attention with Hierarchical Biases for Structure-aware Long Document Summarization
PROCEEDINGS OF THE 60TH ANNUAL MEETING OF THE ASSOCIATION FOR COMPUTATIONAL LINGUISTICS (ACL 2022), VOL 1: (LONG PAPERS)(2022)
摘要
Document structure is critical for efficient information consumption. However, it is challenging to encode it efficiently into the modern Transformer architecture. In this work, we present HIBRIDS, which injects Hierarchical Biases foR Incorporating Document Structure into the calculation of attention scores. We further present a new task, hierarchical question-summary generation, for summarizing salient content in the source document into a hierarchy of questions and summaries, where each follow-up question inquires about the content of its parent question-summary pair. We also annotate a new dataset with 6, 153 question-summary hierarchies labeled on long government reports. Experiment results show that our model produces better question-summary hierarchies than comparisons on both hierarchy quality and content coverage, a finding also echoed by human judges. Additionally, our model improves the generation of long-form summaries from lengthy government reports and Wikipedia articles, as measured by ROUGE scores.
更多查看译文
关键词
hierarchical biases,attention,structure-aware
AI 理解论文
溯源树
样例
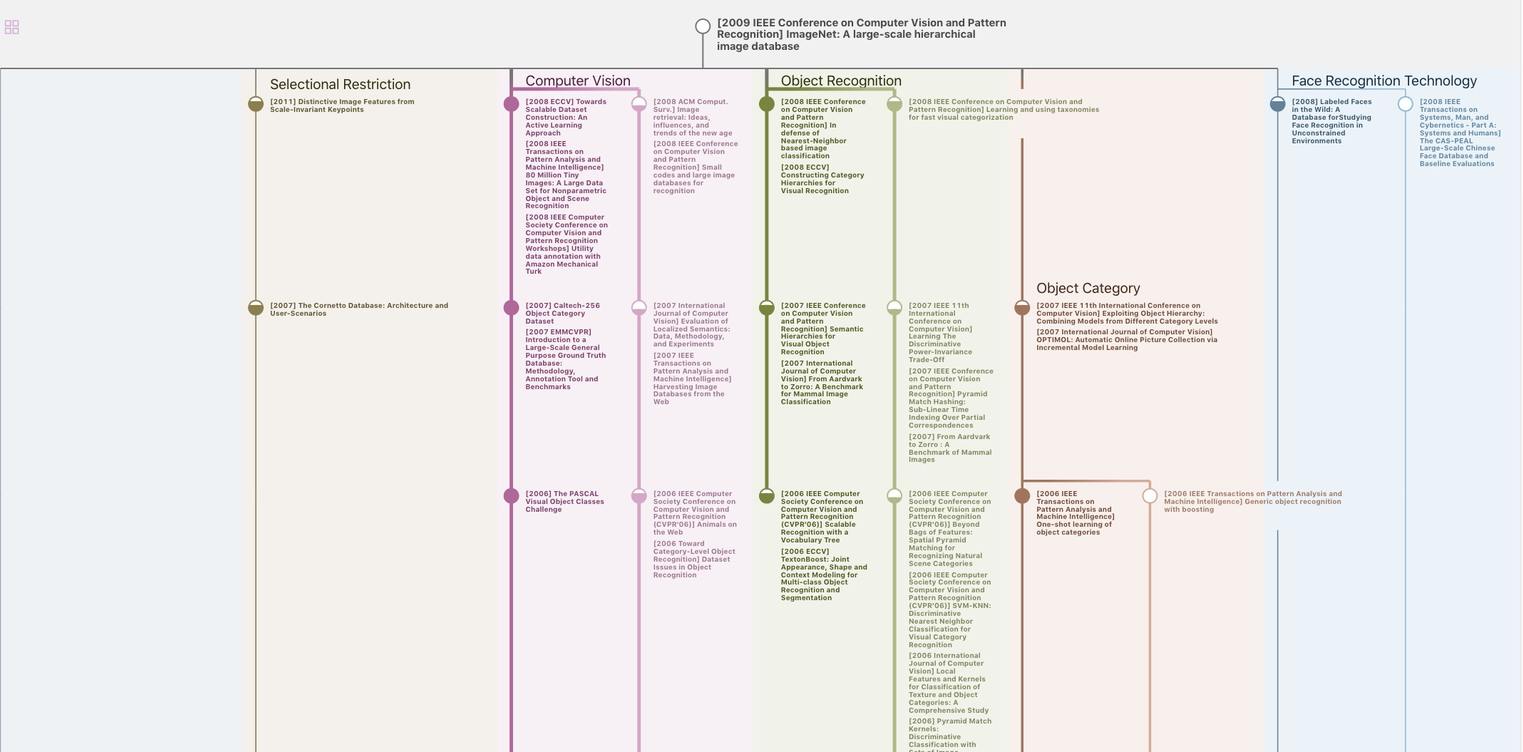
生成溯源树,研究论文发展脉络
Chat Paper
正在生成论文摘要