Self-Supervised Point Cloud Completion on Real Traffic Scenes Via Scene-Concerned Bottom-Up Mechanism
IEEE International Conference on Multimedia and Expo (ICME)(2022)
摘要
Real scans always miss partial geometries of objects due to the self-occlusions, external-occlusions, and limited sensor resolutions. Point cloud completion aims to refer the complete shapes for incomplete 3D scans of objects. Current deep learning-based approaches rely on large-scale complete shapes in the training process, which are usually obtained from synthetic datasets. It is not applicable for real-world scans due to the domain gap. In this paper, we propose a self-supervised point cloud completion method (TraPCC) for vehicles in real traffic scenes without any complete data. Based on the symmetry and similarity of vehicles, we make use of consecutive point cloud frames to construct vehicle memory bank as reference. We design a bottom-up mechanism to focus on both local geometry details and global shape features of inputs. In addition, we design a scene-graph in the network to pay attention to the missing parts by the aid of neighboring vehicles. Experiments show that TraPCC achieve good performance for real-scan completion on KITTI and nuScenes traffic datasets even without any complete data in training. We also show a downstream application of 3D detection, which benefits from our completion approach.
更多查看译文
关键词
point cloud completion,self-supervised,scene-concerned
AI 理解论文
溯源树
样例
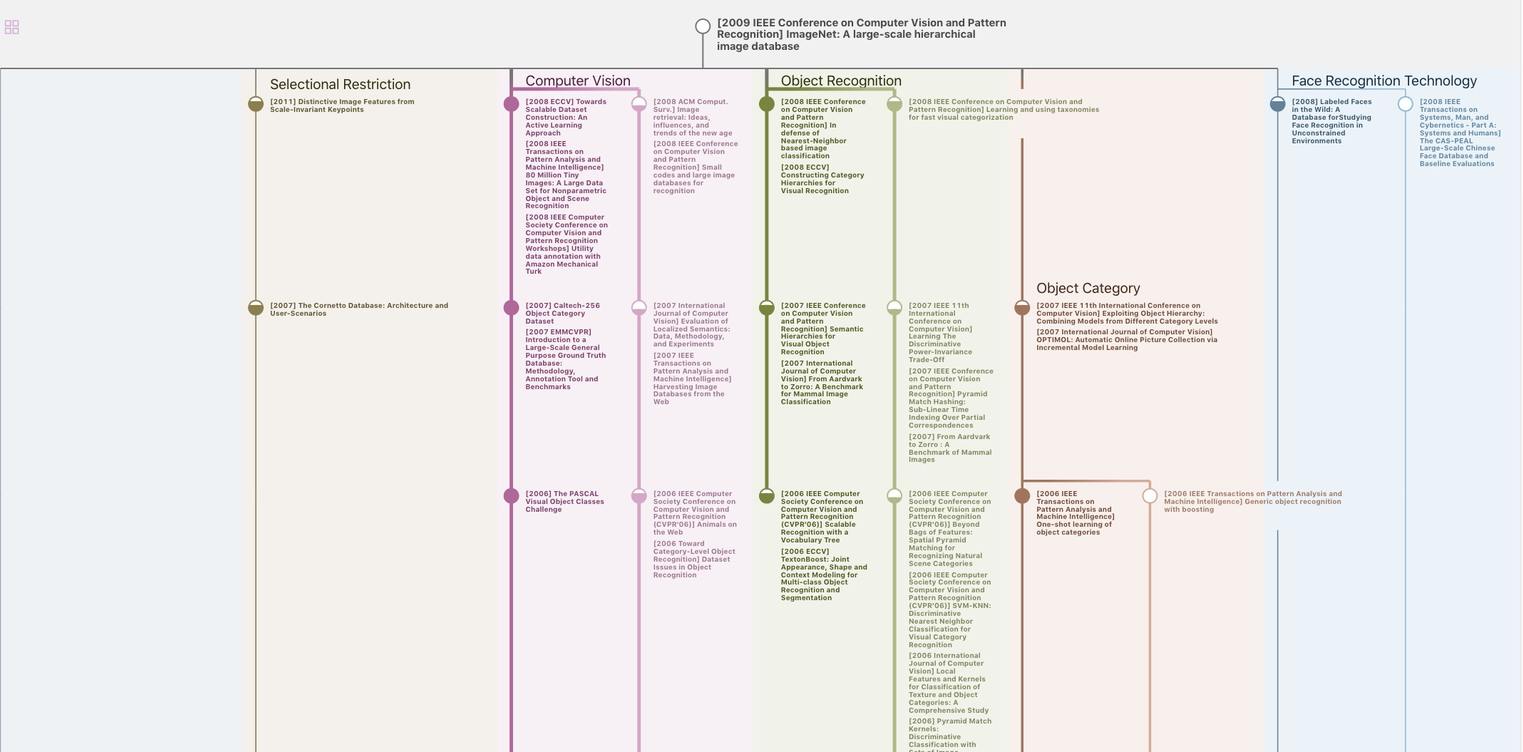
生成溯源树,研究论文发展脉络
Chat Paper
正在生成论文摘要