Single-image Human-body Reshaping with Deep Neural Networks
arxiv(2022)
摘要
In this paper, we present NeuralReshaper, a novel method for semantic reshaping of human bodies in single images using deep generative networks. To achieve globally coherent reshaping effects, our approach follows a fit-then-reshape pipeline, which first fits a parametric 3D human model to a source human image and then reshapes the fitted 3D model with respect to user-specified semantic attributes. Previous methods rely on image warping to transfer 3D reshaping effects to the entire image domain and thus often cause distortions in both foreground and background. Instead, to achieve more realistic reshaping results, we resort to generative adversarial nets conditioned on the source image and a 2D warping field induced by the reshaped 3D model. Specifically, we separately encode the foreground and background information in the source image using a two-headed U-net-like generator and guide the information flow from the foreground branch to the background branch via feature space warping. Furthermore, to deal with the lack-of-data problem that no paired data exist (i.e., the same human bodies in varying shapes), we introduce a novel weakly-supervised strategy to train our network. Besides, unlike previous methods that often require manual efforts to correct undesirable artifacts caused by incorrect body-to-image fitting, our method is fully automatic. Extensive experiments on both indoor and outdoor datasets demonstrate the superiority of our method over previous approaches.
更多查看译文
AI 理解论文
溯源树
样例
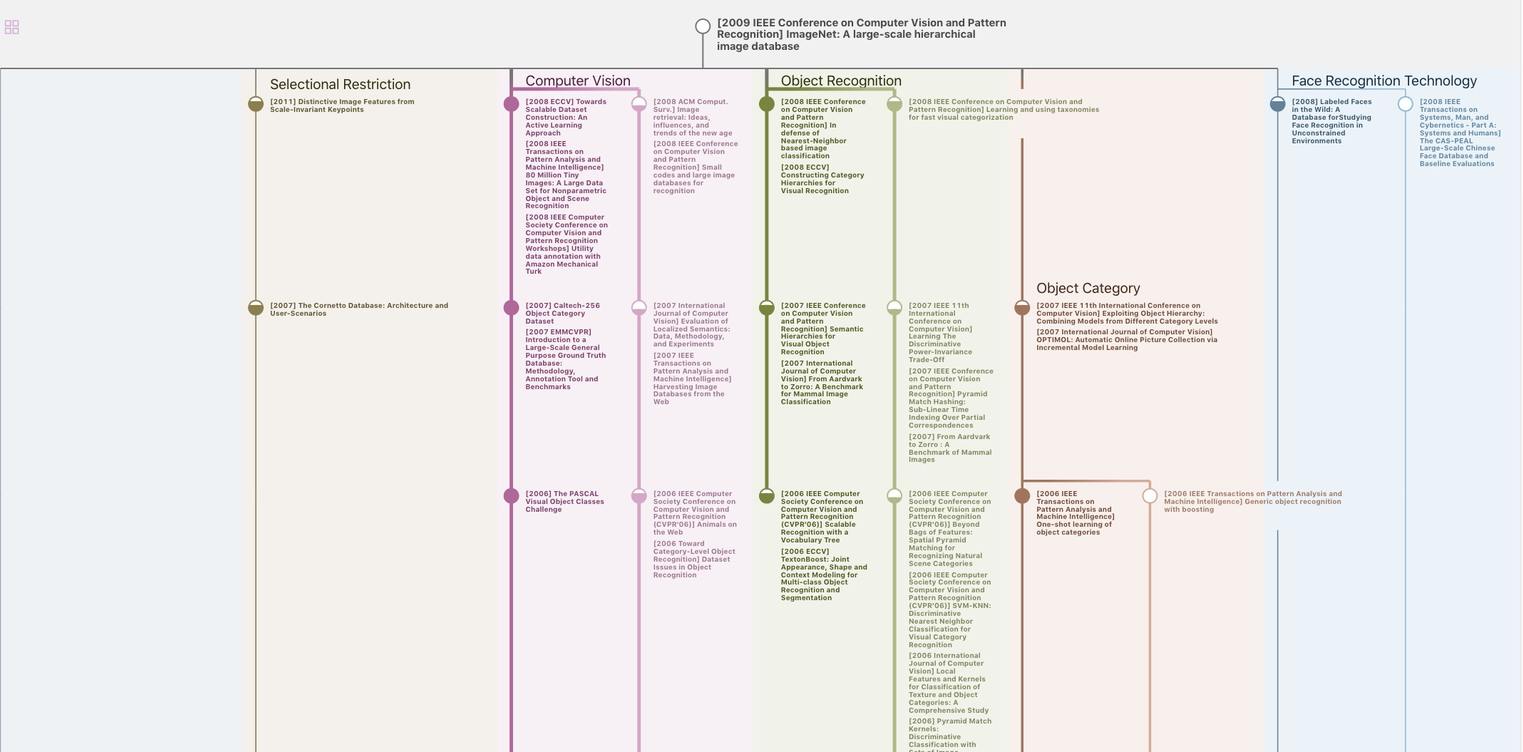
生成溯源树,研究论文发展脉络
Chat Paper
正在生成论文摘要