Neural Machine Translation with Phrase-Level Universal Visual Representations
PROCEEDINGS OF THE 60TH ANNUAL MEETING OF THE ASSOCIATION FOR COMPUTATIONAL LINGUISTICS (ACL 2022), VOL 1: (LONG PAPERS)(2022)
摘要
Multimodal machine translation (MMT) aims to improve neural machine translation (NMT) with additional visual information, but most existing MMT methods require paired input of source sentence and image, which makes them suffer from shortage of sentence-image pairs. In this paper, we propose a phrase-level retrieval-based method for MMT to get visual information for the source input from existing sentence-image data sets so that MMT can break the limitation of paired sentence-image input. Our method performs retrieval at the phrase level and hence learns visual information from pairs of source phrase and grounded region, which can mitigate data sparsity. Furthermore, our method employs the conditional variational auto-encoder to learn visual representations which can filter redundant visual information and only retain visual information related to the phrase. Experiments show that the proposed method significantly outperforms strong baselines on multipleMMTdatasets, especially when the textual context is limited.
更多查看译文
关键词
representations,translation,phrase-level
AI 理解论文
溯源树
样例
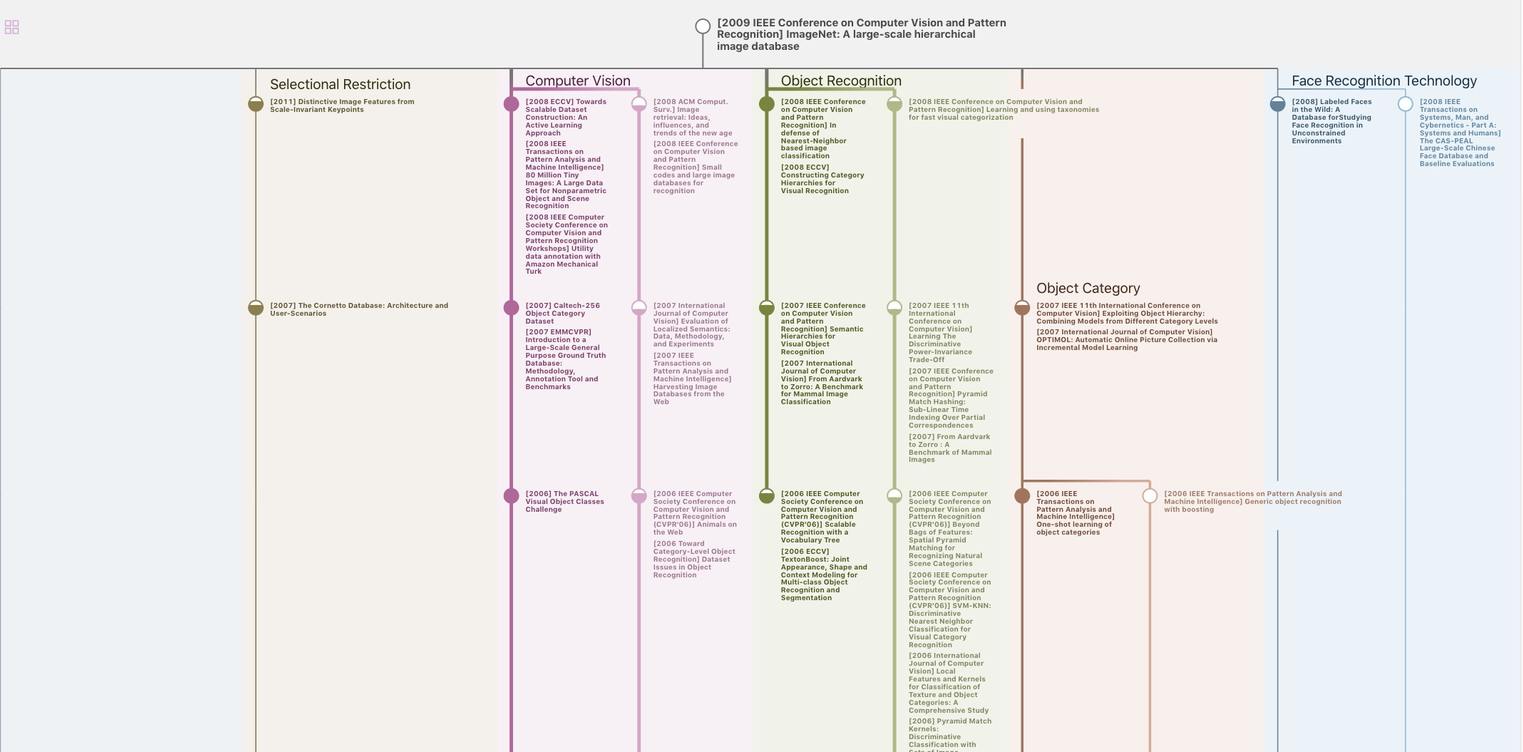
生成溯源树,研究论文发展脉络
Chat Paper
正在生成论文摘要