Cost Function Learning in Memorized Social Networks With Cognitive Behavioral Asymmetry
IEEE TRANSACTIONS ON COMPUTATIONAL SOCIAL SYSTEMS(2024)
Abstract
article investigates the cost function learning in social information networks, wherein human memory and cognitive bias are explicitly taken into account. We first propose a model for social information-diffusion dynamics, with a focus on the systematic modeling of asymmetric cognitive bias represented by confirmation bias and novelty bias. Building on the dynamics model, we then propose the (MIRL)-I-3-a memorized model and maximum-entropy-based inverse reinforcement learning- for learning cost functions. Compared with the existing model-free IRLs, the characteristics of (MIRL)-I-3 are significantly different here: no dependence on the Markov decision process principle, the need for only a single finite-time trajectory sample, and bounded decision variables. Finally, the effectiveness of the proposed social information-diffusion model and the (MIRL)-I-3 algorithm is validated by the online social media data.
MoreTranslated text
Key words
Asymmetric confirmation bias,asymmetric novelty bias,cost function learning,human memory,inverse reinforcement learning (IRL),social information-diffusion dynamics
AI Read Science
Must-Reading Tree
Example
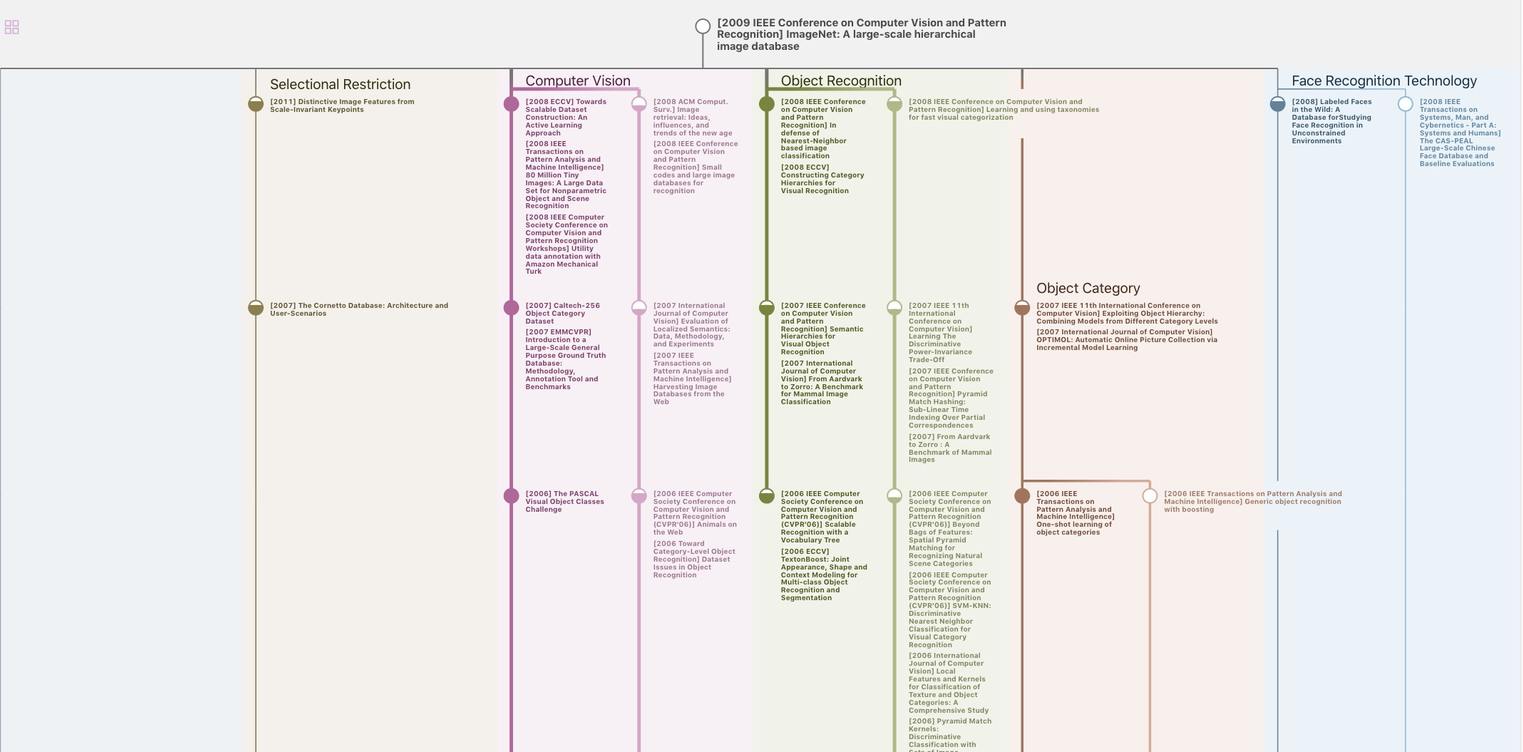
Generate MRT to find the research sequence of this paper
Chat Paper
Summary is being generated by the instructions you defined