Unsteady aerodynamic reduced-order modeling based on machine learning across multiple airfoils
Aerospace Science and Technology(2021)
Abstract
Computational-fluid-dynamics-based prediction of unsteady aerodynamics is an essential research topic in the design of aircraft, which usually requires very high computational cost. Therefore, developing efficient and accurate reduced-order models (ROMs) for unsteady aerodynamics is important. With recent progress in modeling unsteady aerodynamics for a fixed configuration, there is a need of developing reduced-order models across multiple geometrical shapes. In this work, the long short-term memory (LSTM) network, a traditional model in machine learning for modeling time-dependent dynamics, is utilized to construct the unsteady aerodynamic reduced-order model, which takes into account the variation of airfoil geometry. The proposed approach is illustrated by some NACA 6-series airfoils under pitching and plunging motions in the transonic flow, where the geometrical parametrization is based on the maximum camber and thickness. Results show that the model can accurately capture the unsteady aerodynamics for varying shape parameters. Finally, as a proof of concept, the constructed ROM is applied to uncertainty and sensitivity analysis with respect to the variation of geometry parameters.
MoreTranslated text
Key words
Reduced-order model,Long short-term memory network,Unsteady aerodynamics,NACA 6-series airfoils
AI Read Science
Must-Reading Tree
Example
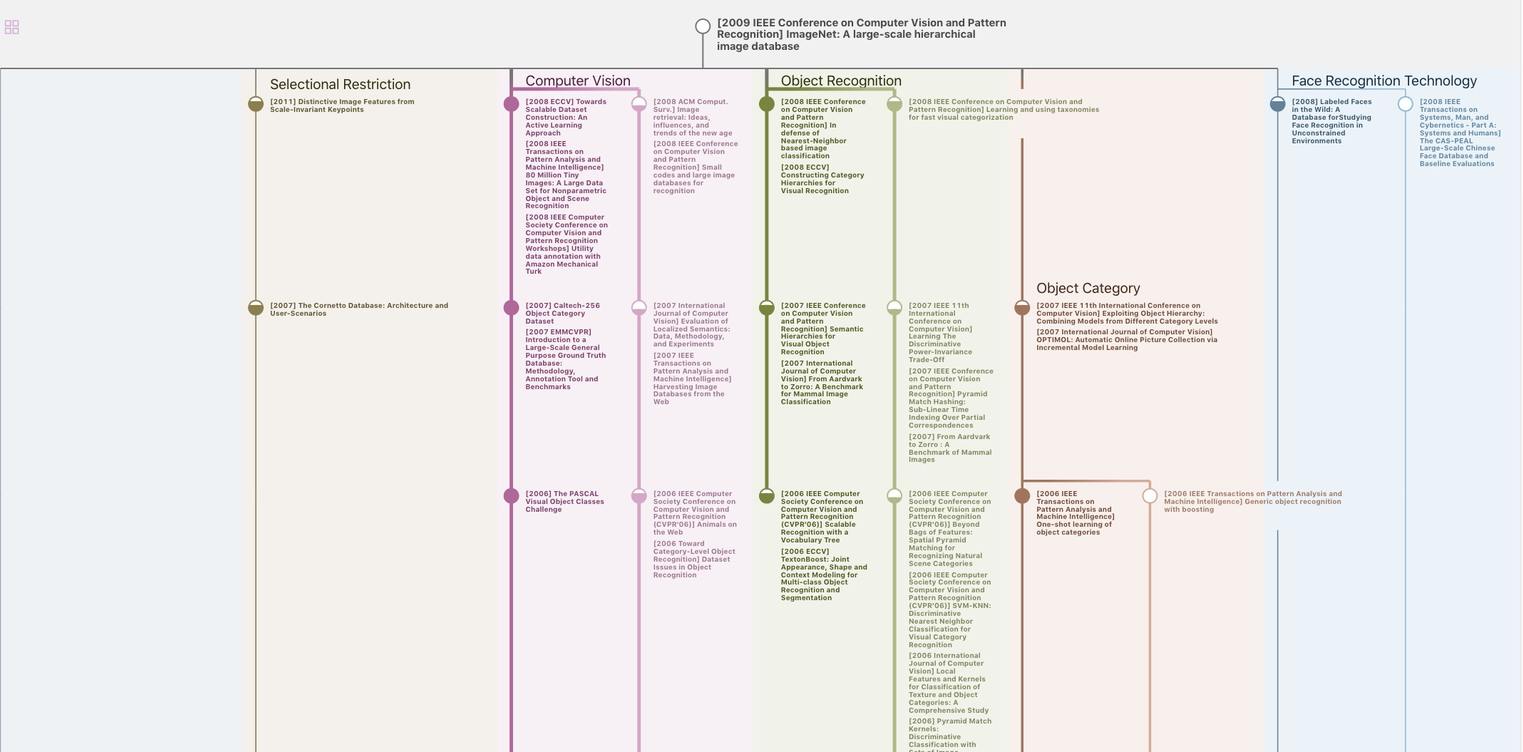
Generate MRT to find the research sequence of this paper
Chat Paper
Summary is being generated by the instructions you defined