Optimizing a superconducting radio-frequency gun using deep reinforcement learning
PHYSICAL REVIEW ACCELERATORS AND BEAMS(2022)
摘要
Superconducting photoelectron injectors are promising for generating highly brilliant pulsed electron beams with high repetition rates and low emittances. Experiments such as ultrafast electron diffraction, experiments at the Terahertz scale, and energy recovery linac applications require such properties. However, optimizing the beam properties is challenging due to the high number of possible machine parameter combinations. This article shows the successful automated optimization of beam properties utilizing an already existing simulation model. To reduce the required computation time, we replace the costly simulation with a faster approximation with a neural network. For optimization, we propose a reinforcement learning approach leveraging the simple computation of the derivative of the approximation. We prove that our approach outperforms standard optimization methods for the required function evaluations given a defined minimum accuracy.
更多查看译文
关键词
learning,radio-frequency
AI 理解论文
溯源树
样例
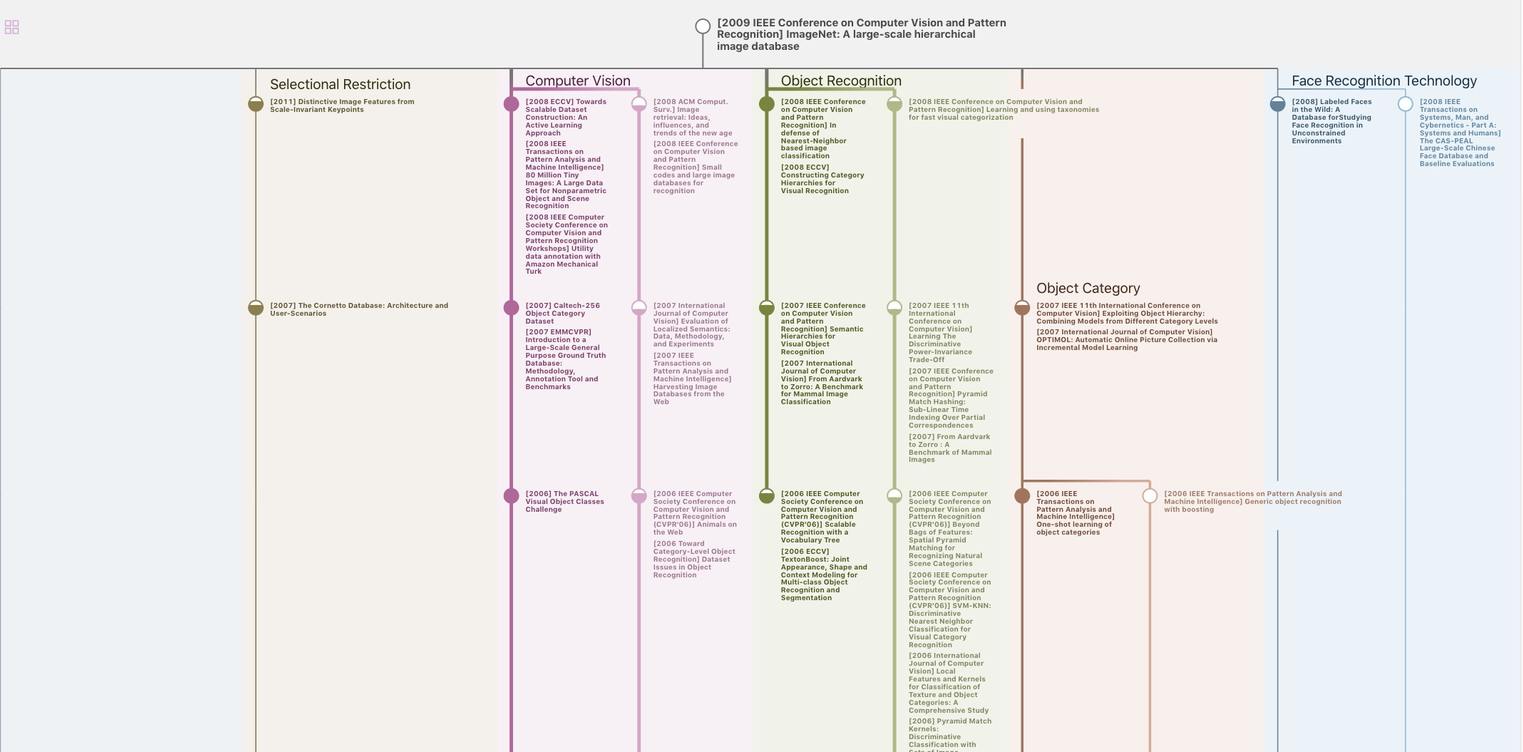
生成溯源树,研究论文发展脉络
Chat Paper
正在生成论文摘要