Patch2Self denoising of Diffusion MRI with Self-Supervision and Matrix Sketching
bioRxiv(2022)
摘要
Diffusion-weighted magnetic resonance imaging (DWI) is the only noninvasive method for quantifying microstructure and reconstructing white-matter pathways in the living human brain. Fluctuations from multiple sources create significant additive noise in DWI data which must be suppressed before subsequent microstructure analysis. We introduce a self-supervised learning method for denoising DWI data, Patch2Self (P2S), which uses the entire volume to learn a full-rank locally linear denoiser for that volume. By taking advantage of the oversampled q -space of DWI data, P2S can separate structure from noise without requiring an explicit model for either. The setup of P2S however can be resource intensive, both in terms of running time and memory usage, as it uses all voxels ( n ) from all-but-one held-in volumes ( d − 1) to learn a linear mapping Φ : ℝ n ×( d −1) ↦ ℝ n for denoising the held-out volume. We exploit the redundancy imposed by P2S to alleviate its performance issues and inspect regions that influence the noise disproportionately. Specifically we introduce P2S-sketch, which makes a two-fold contribution: (1) P2S-sketch uses matrix sketching to perform self-supervised denoising. By solving a sub-problem on a smaller sub-space, so called, coreset , we show how P2S can yield a significant speedup in training time while using less memory. (2) We show how the so-called statistical leverage scores can be used to interpret the denoising of dMRI data, a process that was traditionally treated as a black-box. Our experiments conducted on simulated and real data clearly demonstrate that P2S via matrix sketching (P2S-sketch) does not lead to any loss in denoising quality, while yielding significant speedup and improved memory usage by training on a smaller fraction of the data. With thorough comparisons on real and simulated data, we show that Patch2Self outperforms the current state-of-the-art methods for DWI denoising both in terms of visual conspicuity and downstream modeling tasks. We demonstrate the effectiveness of our approach via multiple quantitative metrics such as fiber bundle coherence, R 2 via cross-validation on model fitting, mean absolute error of DTI residuals across a cohort of sixty subjects.
### Competing Interest Statement
The authors have declared no competing interest.
更多查看译文
关键词
diffusion mri,self-supervision
AI 理解论文
溯源树
样例
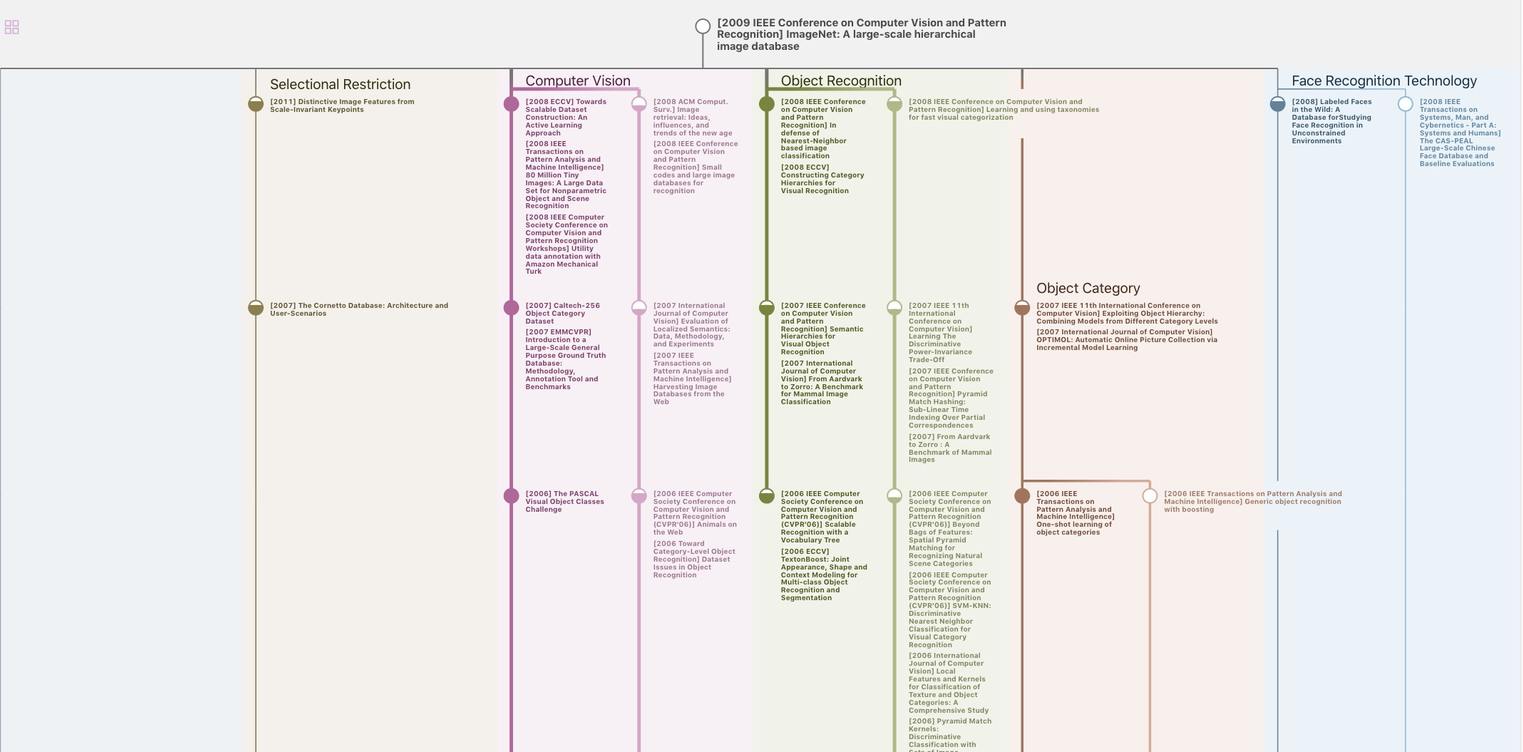
生成溯源树,研究论文发展脉络
Chat Paper
正在生成论文摘要