Digital soil mapping of soil total nitrogen based on Landsat 8, Sentinel 2, and WorldView-2 images in smallholder farms in Yellow River Basin, China
Environmental Monitoring and Assessment(2022)
摘要
Predicting spatial explicit information of soil nutrients is critical for sustainable soil management and food security under climate change and human disturbance in agricultural land. Digital soil mapping (DSM) techniques can utilize soil-landscape information from remote sensing data to predict the spatial pattern of soil nutrients, and it is important to explore the effects of remote sensing data types on DSM. This research utilized Landsat 8 (LT), Sentinel 2 (ST), and WorldView-2 (WV) remote sensing data and employed partial least squares regression (PLSR), random forest (RF), and support vector machine (SVM) algorithms to characterize the spatial pattern of soil total nitrogen (TN) in smallholder farm settings in Yellow River Basin, China. The overall relationships between TN and spectral indices from LT and ST were stronger than those from WV. Multiple red edge band-based spectral indices from ST and WV were relevant variables for TN, while there were no red band-based spectral indices from ST and WV identified as relevant variables for TN. Soil moisture and vegetation were major driving forces of soil TN spatial distribution in this area. The research also concluded that farmlands of crop rotation had relatively higher TN concentration compared with farmlands of monoculture. The soil prediction models based on WV achieved relatively lower model performance compared with those based on ST and LT. The effects of remote sensing data spectral resolution and spectral range on enhancing soil prediction model performance are higher than the effects of remote sensing data spatial resolution. Soil prediction models based on ST can provide location-specific soil maps, achieve fair model performance, and have low cost. This research suggests DSM research utilizing ST has relatively high prediction accuracy, and can produce soil maps that are fit for the spatial explicit soil management for smallholder farms.
更多查看译文
关键词
Digital soil mapping, Soil nitrogen, Spatial prediction, Soil nutrients, Food security, Soil management
AI 理解论文
溯源树
样例
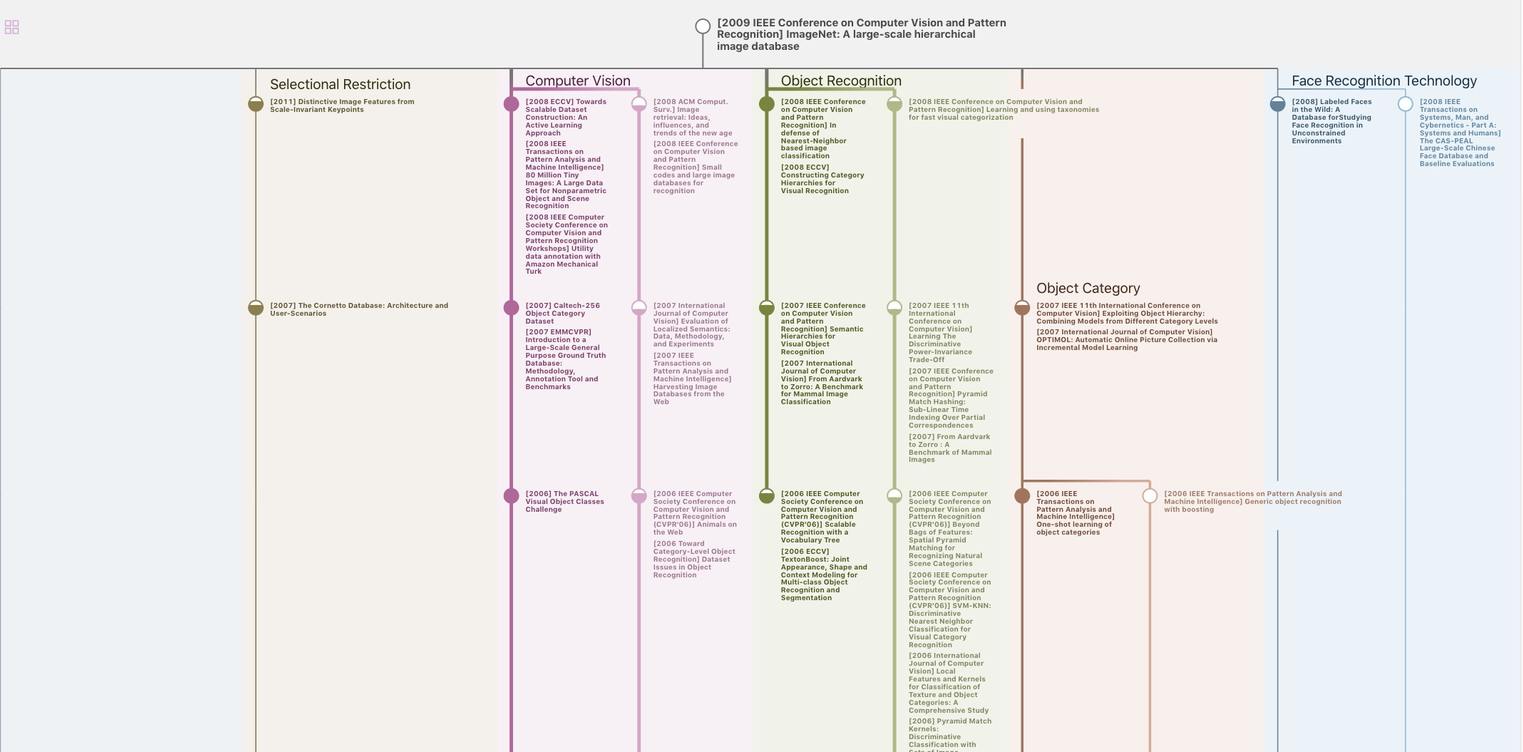
生成溯源树,研究论文发展脉络
Chat Paper
正在生成论文摘要