Progress and Challenges for the Machine Learning-Based Design of Fit-for-purpose Monoclonal Antibodies
mAbs(2022)
Key words
Machine learning,artificial intelligence,antibody,antigen,developability,drug design
AI Read Science
Must-Reading Tree
Example
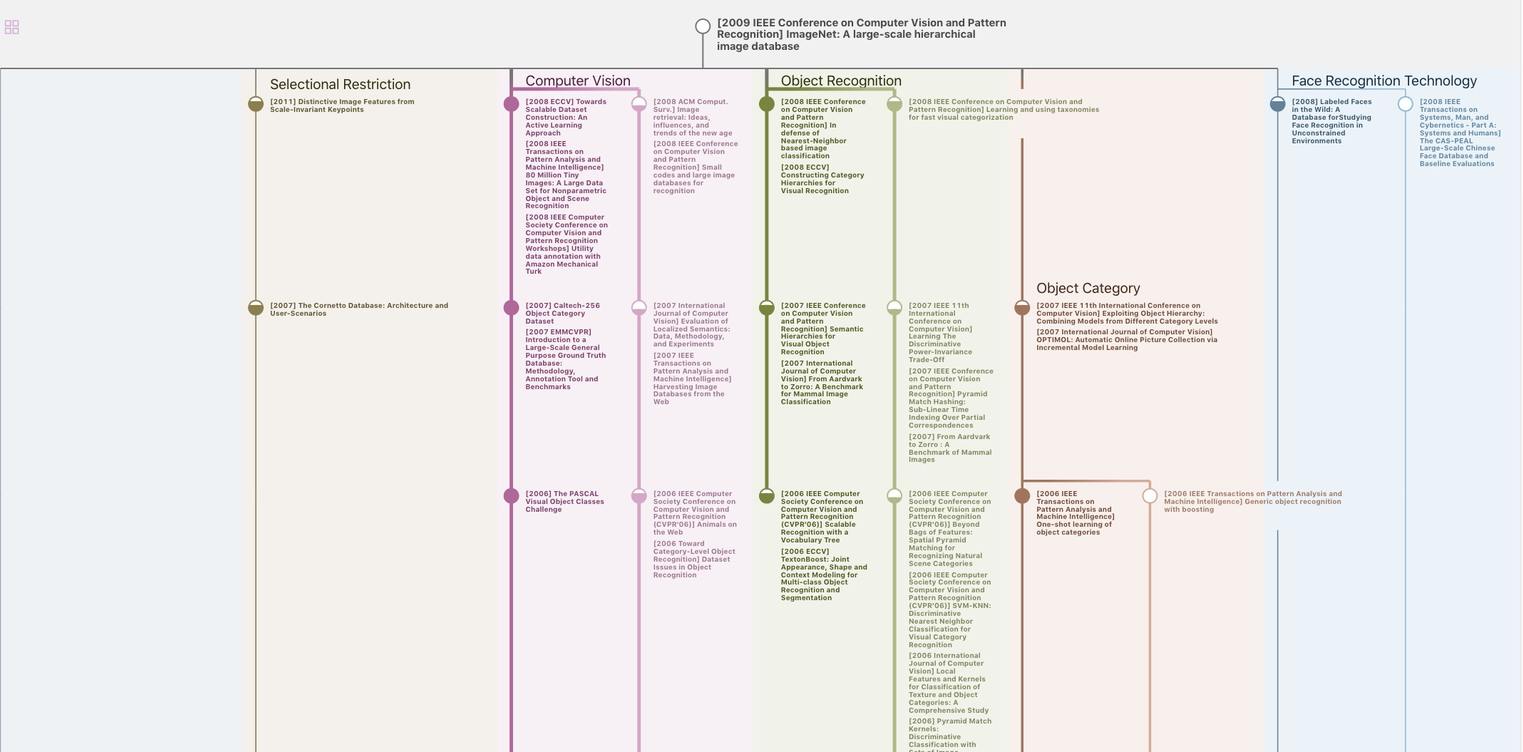
Generate MRT to find the research sequence of this paper
Chat Paper
Summary is being generated by the instructions you defined