Differentiable Multi-Agent Actor-Critic for Multi-Step Radiology Report Summarization
PROCEEDINGS OF THE 60TH ANNUAL MEETING OF THE ASSOCIATION FOR COMPUTATIONAL LINGUISTICS (ACL 2022), VOL 1: (LONG PAPERS)(2022)
摘要
The IMPRESSIONS section of a radiology report about an imaging study is a summary of the radiologist's reasoning and conclusions, and it also aids the referring physician in confirming or excluding certain diagnoses. A cascade of tasks are required to automatically generate an abstractive summary of the typical information-rich radiology report. These tasks include acquisition of salient content from the report and generation of a concise, easily consumable IMPRESSIONS section. Prior research on radiology report summarization has focused on single-step end-to-end models - which subsume the task of salient content acquisition. To fully explore the cascade structure and explainability of radiology report summarization, we introduce two innovations. First, we design a two-step approach: extractive summarization followed by abstractive summarization. Second, we additionally break down the extractive part into two independent tasks: extraction of salient (1) sentences and (2) keywords. Experiments on English radiology reports from two clinical sites show our novel approach leads to a more precise summary compared to single-step and to two-step-with-single-extractive-process baselines with an overall improvement in F1 score of 3-4%.
更多查看译文
关键词
multi-agent,actor-critic,multi-step
AI 理解论文
溯源树
样例
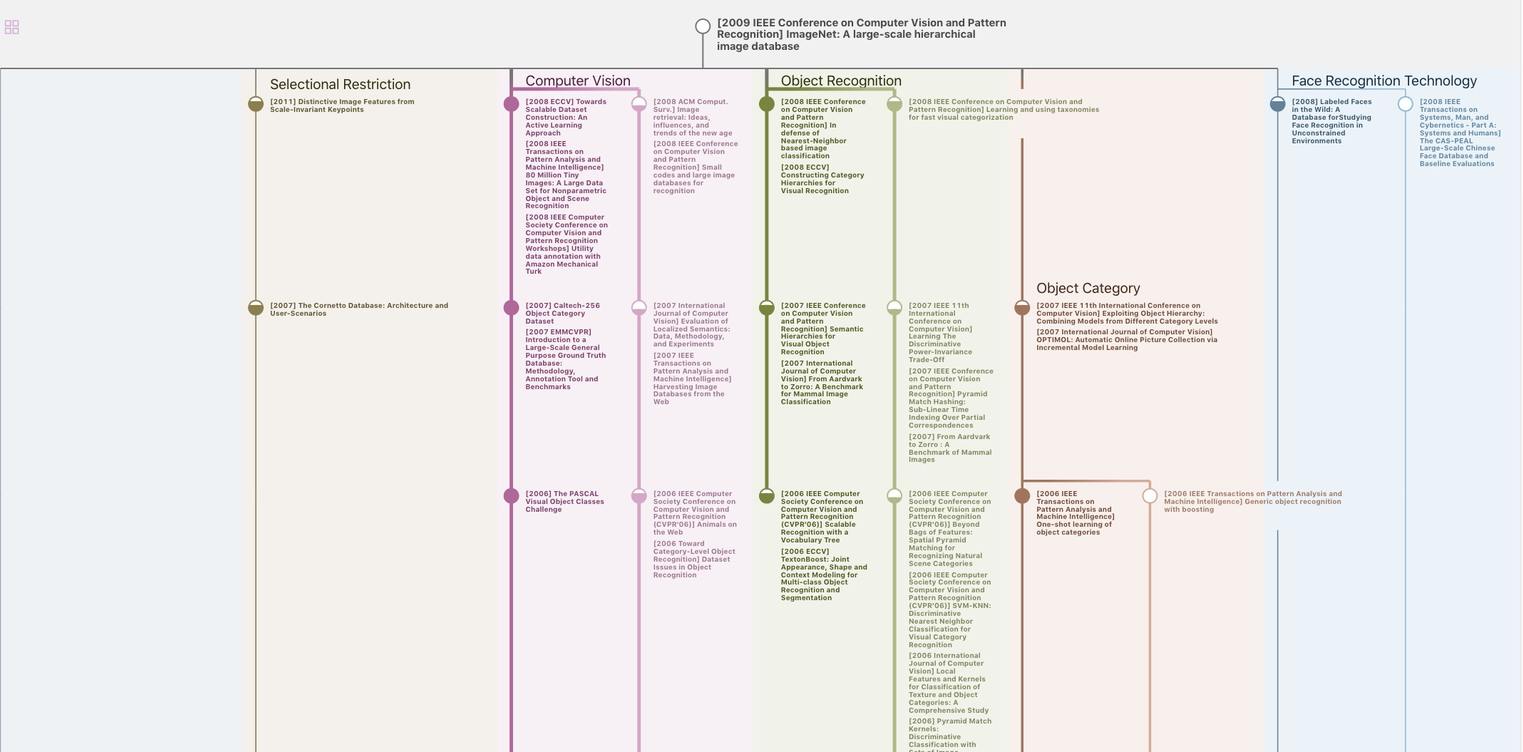
生成溯源树,研究论文发展脉络
Chat Paper
正在生成论文摘要