A Novel Local-Global Graph Convolutional Method for Point Cloud Semantic Segmentation.
IEEE transactions on neural networks and learning systems(2024)
Abstract
Although convolutional neural networks (CNNs) have shown good performance on grid data, they are limited in the semantic segmentation of irregular point clouds. This article proposes a novel and effective graph CNN framework, referred to as the local-global graph convolutional method (LGGCM), which can achieve short- and long-range dependencies on point clouds. The key to this framework is the design of local spatial attention convolution (LSA-Conv). The design includes two parts: generating a weighted adjacency matrix of the local graph composed of neighborhood points, and updating and aggregating the features of nodes to obtain the spatial geometric features of the local point cloud. In addition, a smooth module for central points is incorporated into the process of LSA-Conv to enhance the robustness of the convolution against noise interference by adjusting the position coordinates of the points adaptively. The learned robust LSA-Conv features are then fed into a global spatial attention module with the gated unit to extract long-range contextual information and dynamically adjust the weights of features from different stages. The proposed framework, consisting of both encoding and decoding branches, is an end-to-end trainable network for semantic segmentation of 3-D point clouds. The theoretical analysis of the approximation capabilities of LSA-Conv is discussed to determine whether the features of the point cloud can be accurately represented. Experimental results on challenging benchmarks of the 3-D point cloud demonstrate that the proposed framework achieves excellent performance.
MoreTranslated text
Key words
Point cloud compression,Feature extraction,Convolution,Semantics,Correlation,Deep learning,Logic gates,Attention mechanism,deep learning,graph convolutional neural network (CNN),point cloud,semantic segmentation
AI Read Science
Must-Reading Tree
Example
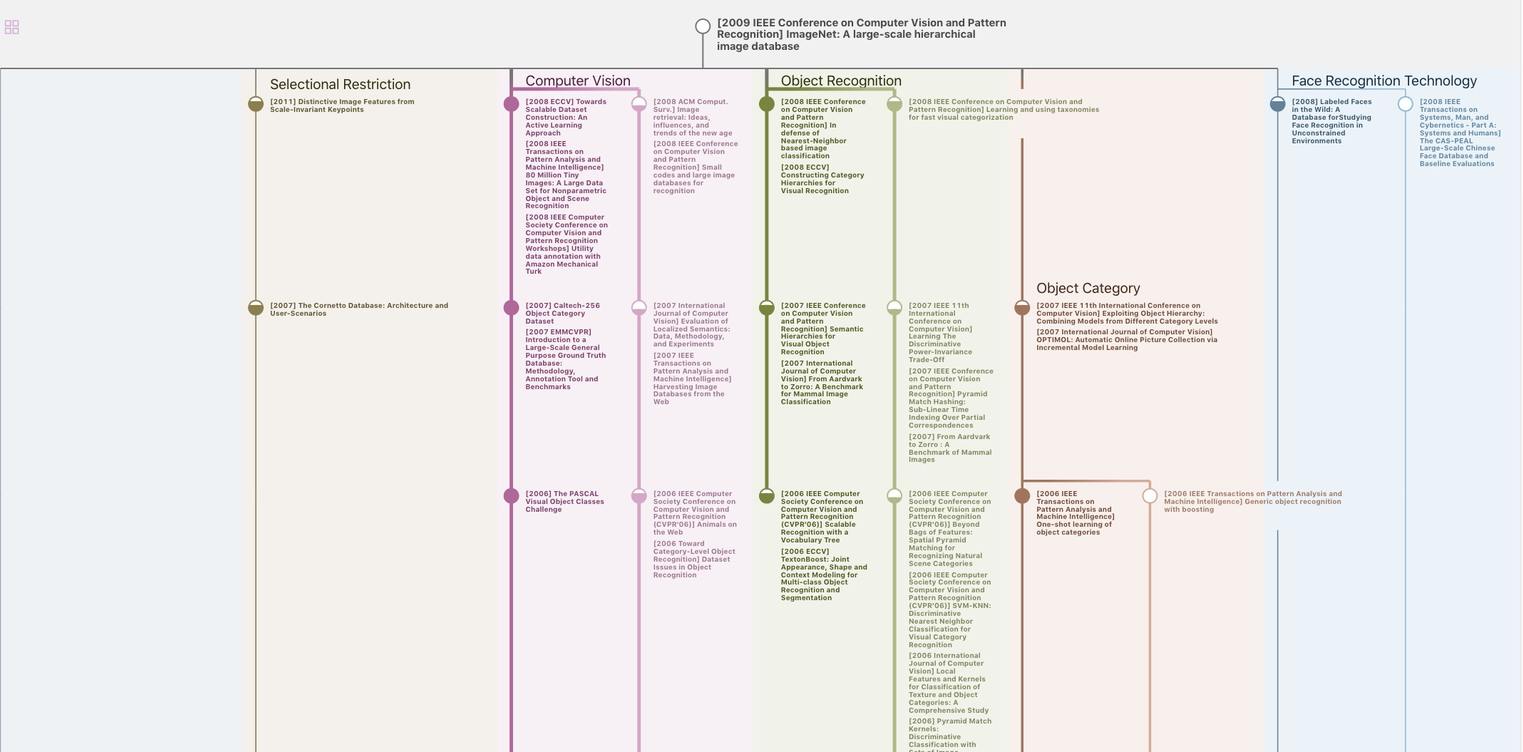
Generate MRT to find the research sequence of this paper
Chat Paper
Summary is being generated by the instructions you defined