A Framework for Verifiable and Auditable Collaborative Anomaly Detection
IEEE ACCESS(2022)
Abstract
Collaborative and Federated Leaning are emerging approaches to manage cooperation between a group of agents for the solution of Machine Learning tasks, with the goal of improving each agent's performance without disclosing any data. In this paper we present a novel algorithmic architecture that tackle this problem in the particular case of Anomaly Detection (or classification of rare events), a setting where typical applications often comprise data with sensible information, but where the scarcity of anomalous examples encourages collaboration. We show how Random Forests can be used as a tool for the development of accurate classifiers with an effective insight-sharing mechanism that does not break the data integrity. Moreover, we explain how the new architecture can be readily integrated in a blockchain infrastructure to ensure the verifiable and auditable execution of the algorithm. Furthermore, we discuss how this work may set the basis for a more general approach for the design of collaborative ensemble-learning methods beyond the specific task and architecture discussed in this paper.
MoreTranslated text
Key words
Collaboration, Collaborative work, Anomaly detection, Radio frequency, Training, Computer architecture, Task analysis, Algorithm auditing, anomaly detection, blockchain, collaborative learning
AI Read Science
Must-Reading Tree
Example
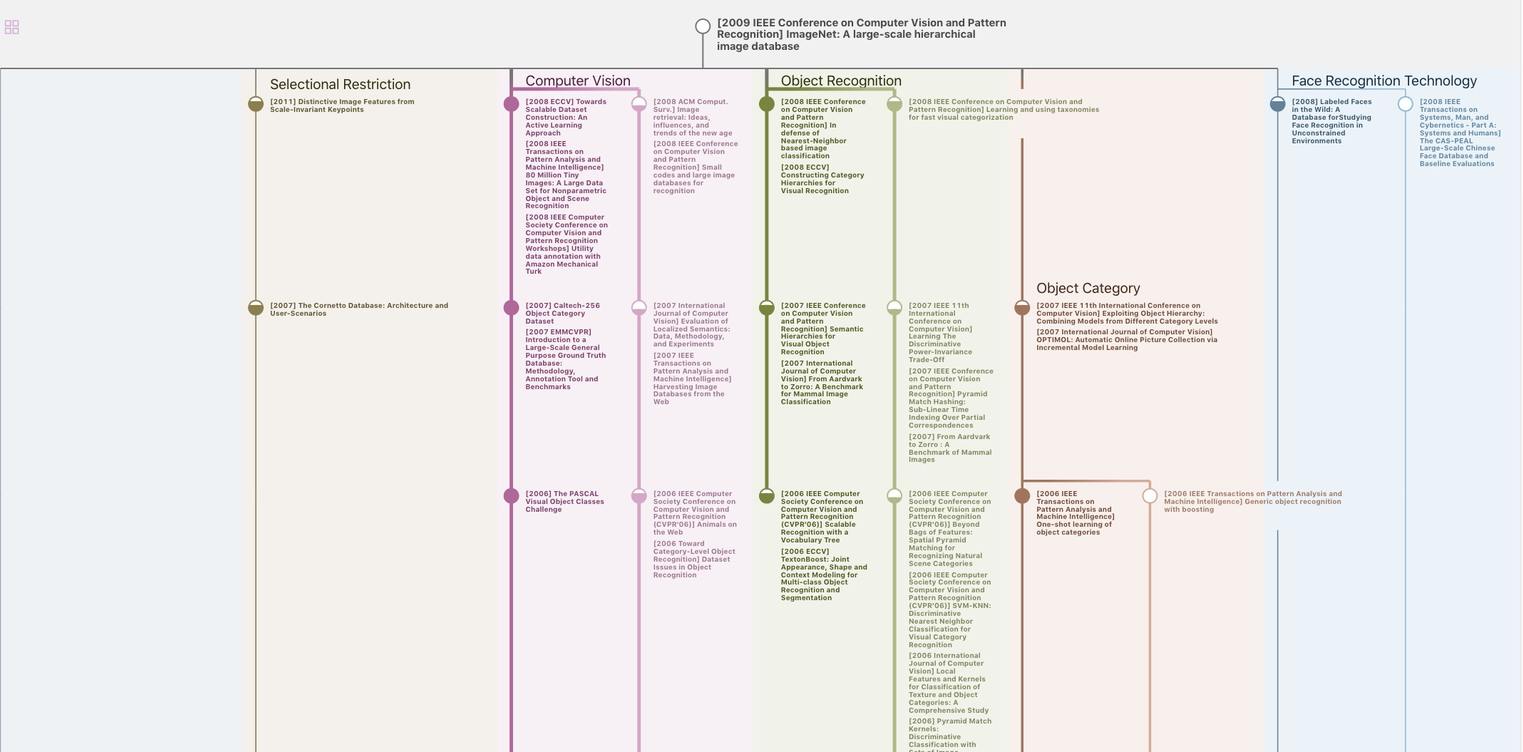
Generate MRT to find the research sequence of this paper
Chat Paper
Summary is being generated by the instructions you defined