Optimal denoising of rotationally invariant rectangular matrices
CoRR(2022)
摘要
In this manuscript we consider denoising of large rectangular matrices: given a noisy observation of a signal matrix, what is the best way of recovering the signal matrix itself? For Gaussian noise and rotationally-invariant signal priors, we completely characterize the optimal denoiser and its performance in the high-dimensional limit, in which the size of the signal matrix goes to infinity with fixed aspects ratio, and under the Bayes optimal setting, that is when the statistician knows how the signal and the observations were generated. Our results generalise previous works that considered only symmetric matrices to the more general case of non-symmetric and rectangular ones. We explore analytically and numerically a particular choice of factorized signal prior that models cross-covariance matrices and the matrix factorization problem. As a byproduct of our analysis, we provide an explicit asymptotic evaluation of the rectangular Harish-Chandra-Itzykson-Zuber integral in a special case.
更多查看译文
关键词
optimal denoising,invariant rectangular matrices
AI 理解论文
溯源树
样例
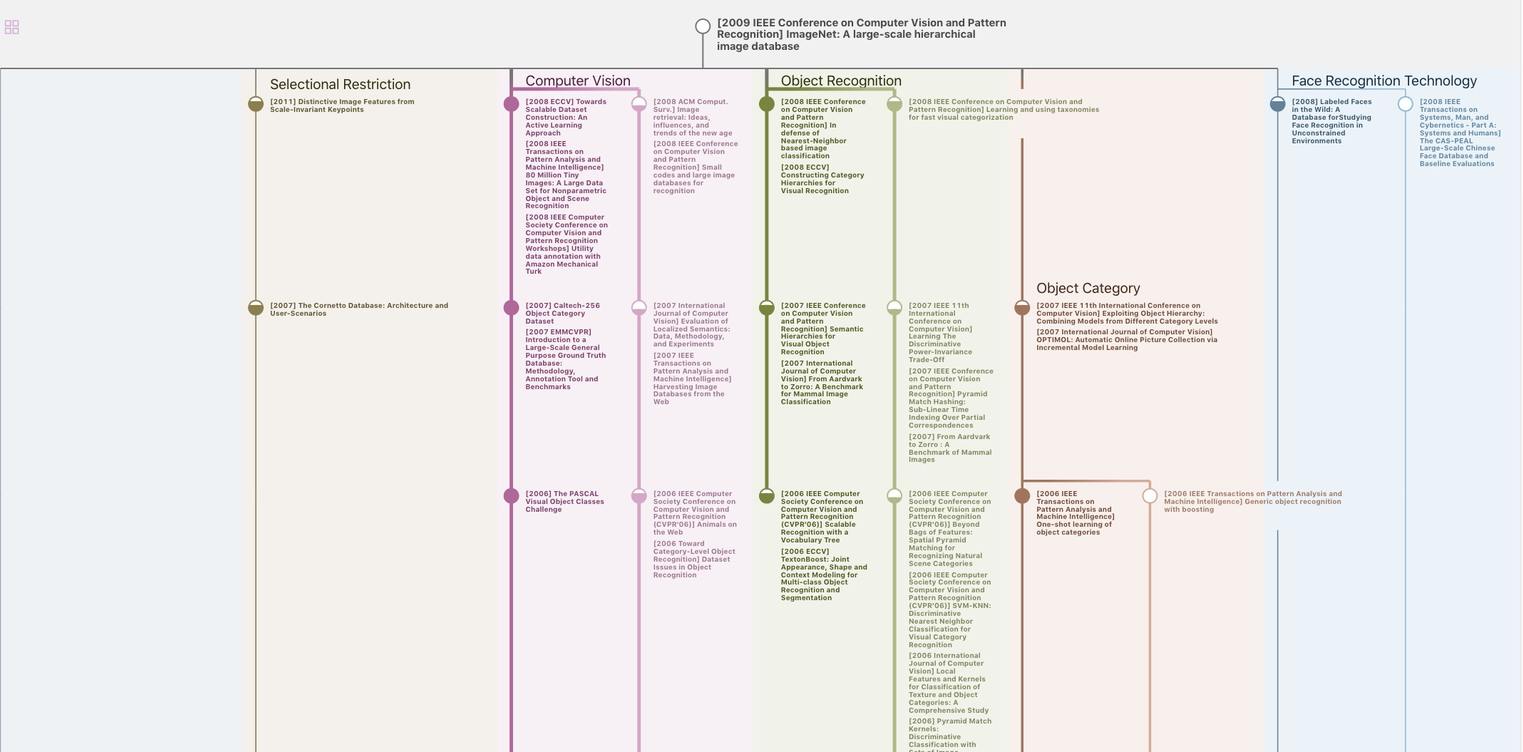
生成溯源树,研究论文发展脉络
Chat Paper
正在生成论文摘要