Utility of Clinical-Radiomic Model to Identify Clinically Significant Prostate Cancer in Biparametric MRI PI-RADS V2.1 Category 3 Lesions
FRONTIERS IN ONCOLOGY(2022)
摘要
PurposeTo determine the predictive performance of the integrated model based on clinical factors and radiomic features for the accurate identification of clinically significant prostate cancer (csPCa) among Prostate Imaging Reporting and Data System (PI-RADS) 3 lesions. Materials and MethodsA retrospective study of 103 patients with PI-RADS 3 lesions who underwent pre-operative 3.0-T MRI was performed. Patients were randomly divided into the training set and the testing set at a ratio of 7:3. Radiomic features were extracted from axial T2WI, diffusion-weighted imaging (DWI), and apparent diffusion coefficient (ADC) images of each patient. The minimum redundancy maximum relevance (mRMR) and least absolute shrinkage and selection operator (LASSO) feature selection methods were used to identify the radiomic features and construct a radiomic model for csPCa identification. Moreover, multivariable logistic regression analysis was used to integrate the clinical factors with radiomic feature model to further improve the accuracy of csPCa identification, and the two are presented in the form of normogram. The performance of the integrated model was compared with radiomic model and clinical model on testing set. ResultsA total of four radiomic features were selected and used for radiomic model construction producing a radiomic score (Radscore). Radscore was significantly different between the csPCa and the non-csPCa patients (training set: p < 0.001; testing set: p = 0.035). Multivariable logistic regression analysis showed that age and PSA could be used as independent predictors for csPCa identification. The clinical-radiomic model produced the receiver operating characteristic (ROC) curve (AUC) in the testing set was 0.88 (95%CI, 0.75-1.00), which was similar to clinical model (AUC = 0.85; 95%CI, 0.52-0.90) (p = 0.048) and higher than the radiomic model (AUC = 0.71; 95%CI, 0.68-1.00) (p < 0.001). The decision curve analysis implies that the clinical-radiomic model could be beneficial in identifying csPCa among PI-RADS 3 lesions. ConclusionThe clinical-radiomic model could effectively identify csPCa among biparametric PI-RADS 3 lesions and thus could help avoid unnecessary biopsy and improve the life quality of patients.
更多查看译文
关键词
radiomics, clinically significant prostate cancer, PI-RADS score 3, nomogram, biparametric MRI (Bp-MRI)
AI 理解论文
溯源树
样例
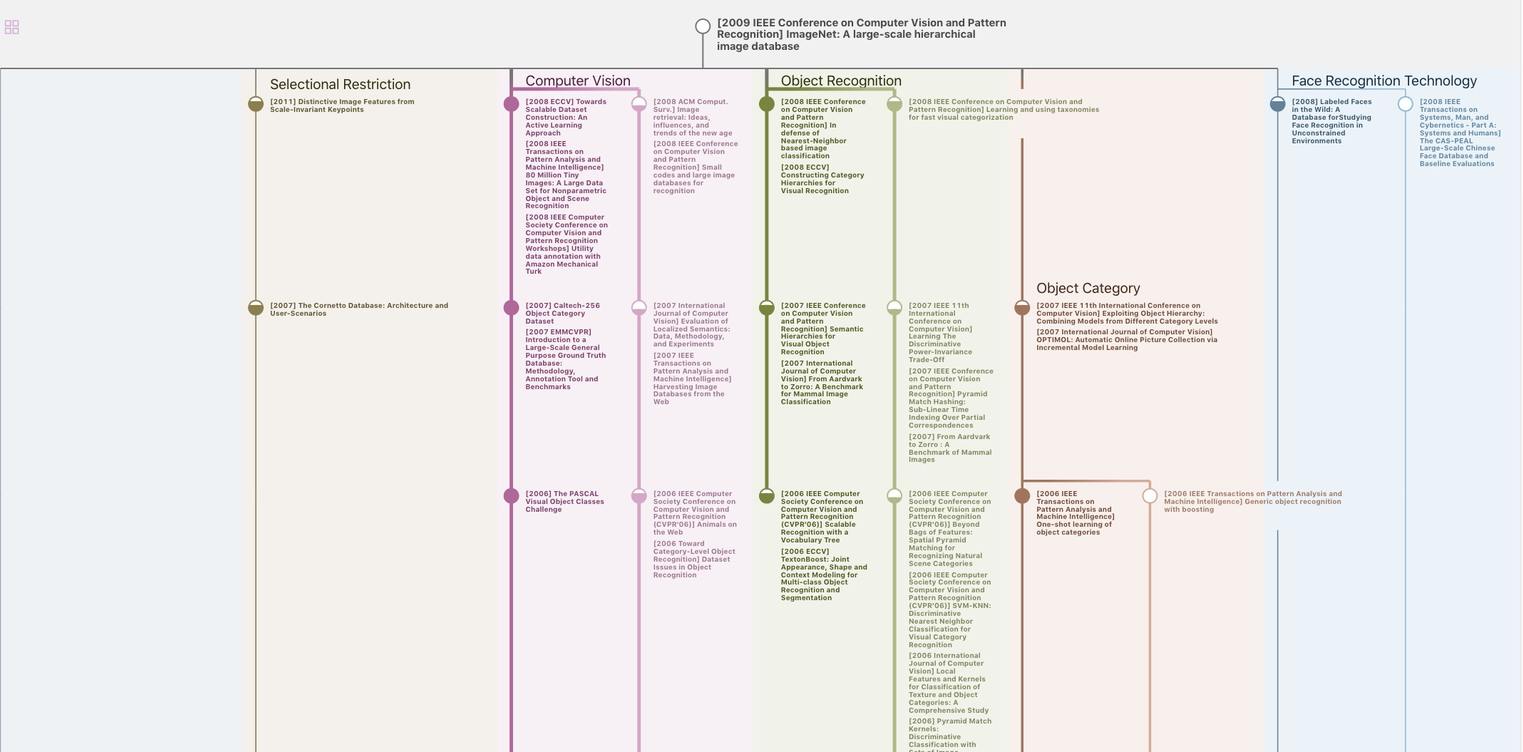
生成溯源树,研究论文发展脉络
Chat Paper
正在生成论文摘要