Not optimal, just noisy: the geometry of correlated variability leads to highly suboptimal sensory coding
biorxiv(2022)
摘要
The brain represents the world through the activity of neural populations. Correlated variability across simultaneously recorded neurons (noise correlations) has been observed across cortical areas and experimental paradigms. Many studies have shown that correlated variability improves stimulus coding compared to a null model with no correlations. However, such results do not shed light on whether neural populations’ correlated variability achieves optimal coding. Here, we assess optimality of noise correlations in diverse datasets by developing two novel null models each with a unique biological interpretation: a uniform correlations null model and a factor analysis null model. We show that across datasets, the correlated variability in neural populations leads to highly suboptimal coding performance according to these null models. We demonstrate that biological constraints prevent many subsets of the neural populations from achieving optimality according to these null models, and that subselecting based on biological criteria leaves coding performance suboptimal. Finally, we show that the optimal subpopulation is exponentially small as a function of neural dimensionality. Together, these results show that the geometry of correlated variability leads to highly suboptimal sensory coding.
### Competing Interest Statement
The authors have declared no competing interest.
更多查看译文
关键词
variability
AI 理解论文
溯源树
样例
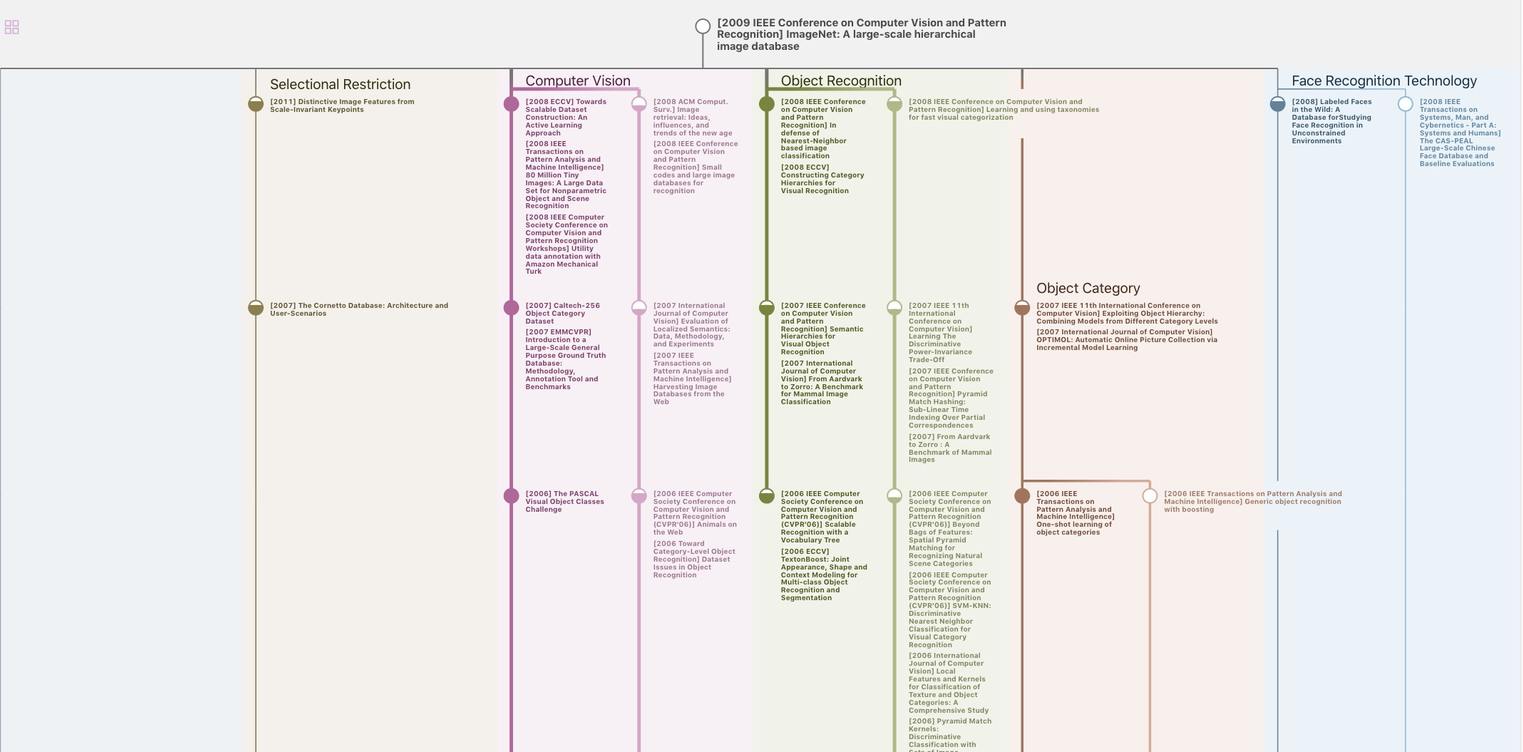
生成溯源树,研究论文发展脉络
Chat Paper
正在生成论文摘要