A comprehensive comparison on cell type composition inference for spatial transcriptomics data
biorxiv(2022)
摘要
Spatial transcriptomic (ST) technologies allow researchers to examine high-quality RNA-sequencing data along with maintained two-dimensional positional information as well as a co-registered histology image. A popular use of ST omics data is to provide insights about tissue structure and spatially unique features. However, due to the technical nature unique to most ST data, the resolution varies from a diameter of 2-10 μm to 50-100 μm instead of single-cell resolution, which brings uncertainty into cell number and cell mixture within each ST spot. Motivated by the important role for spatial arrangement of cell types within a tissue in physiology and disease pathogenesis, several ST deconvolution methods have been developed and are being used to explore gene expression variation and identification of spatial domains. The aim of this work is to review state-of-the-art methods for ST deconvolution, while comparing their strengths and weaknesses. Specifically, we use four real datasets to examine the performance of eight methods across different tissues and technological platforms.
Key Points
### Competing Interest Statement
The authors have declared no competing interest.
更多查看译文
关键词
spatial transcriptomics, single-cell, cell-type deconvolution, deep learning, probabilistic modeling
AI 理解论文
溯源树
样例
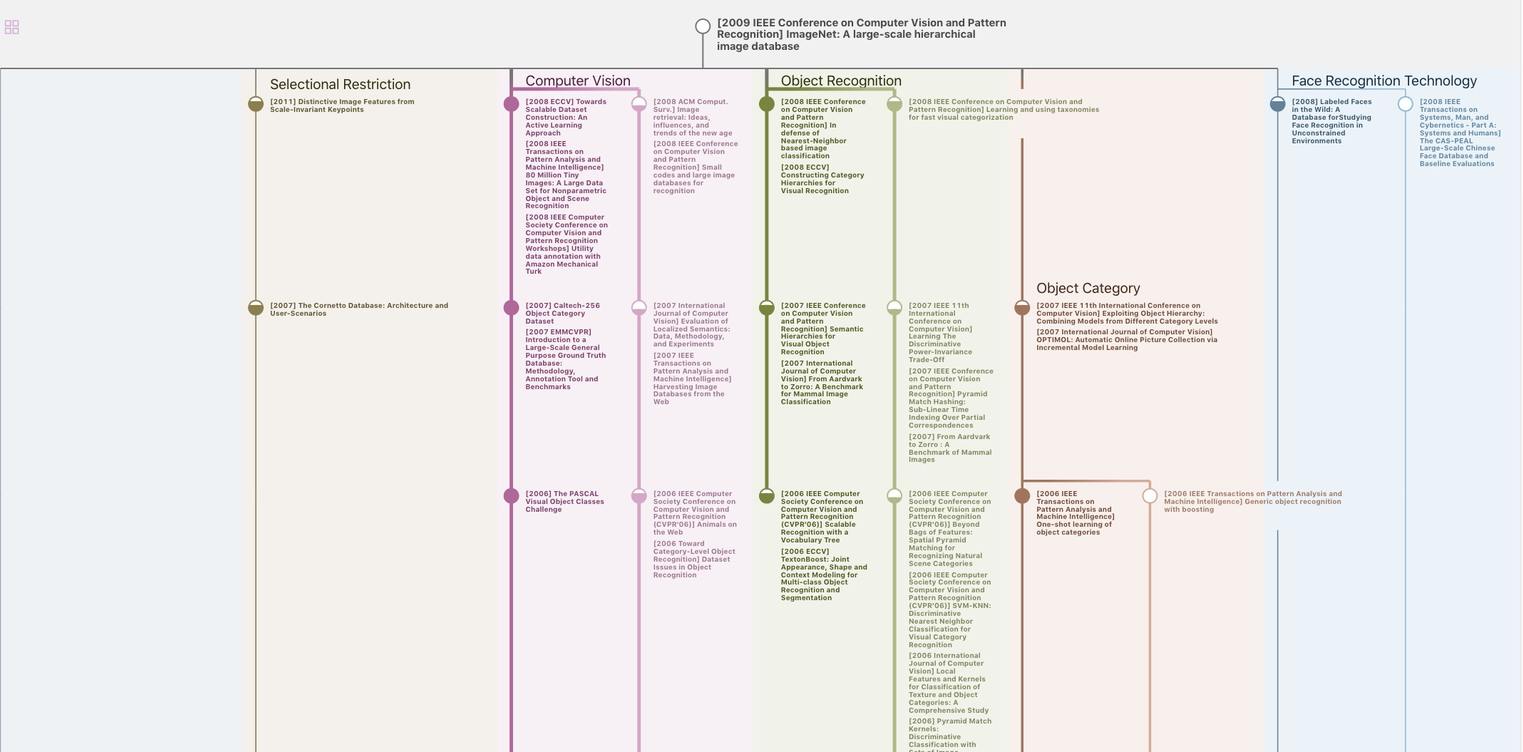
生成溯源树,研究论文发展脉络
Chat Paper
正在生成论文摘要