Image-based tool condition monitoring based on convolution neural network in turning process
The International Journal of Advanced Manufacturing Technology(2022)
摘要
Tool wear has a significant impact on machining quality, efficiency, and cost, so it is vitally important for manufacturing systems. The current work of Tool Condition Monitoring (TCM) mainly processes the time series signals from multisensory using intelligent algorithms. However, the limits of these methods are as follows: (1) the image information is not integrated into the time series signals, and (2) the traditional methods face the problems of poor generalization and fast convergence. Thus, a novel integrated model based on the multisensory feature fusion and neural network is presented. The sensor data is first pre-processed using Piecewise Aggregate Approximation (PAA) and then recoded into images using Gramian Angular Field (GAF). The images, together with the tool infrared images, are inputs to the Convolutional Neural Network (CNN) model, which realizes the output of flank wear value. Both time series signals and tool infrared images are used to achieve the classification, and the final classification accuracy in the test set is 91%. The results show the high computation efficiency and the good generalization performance of the presented methodology.
更多查看译文
关键词
Tool condition monitoring,Deep learning,Turning
AI 理解论文
溯源树
样例
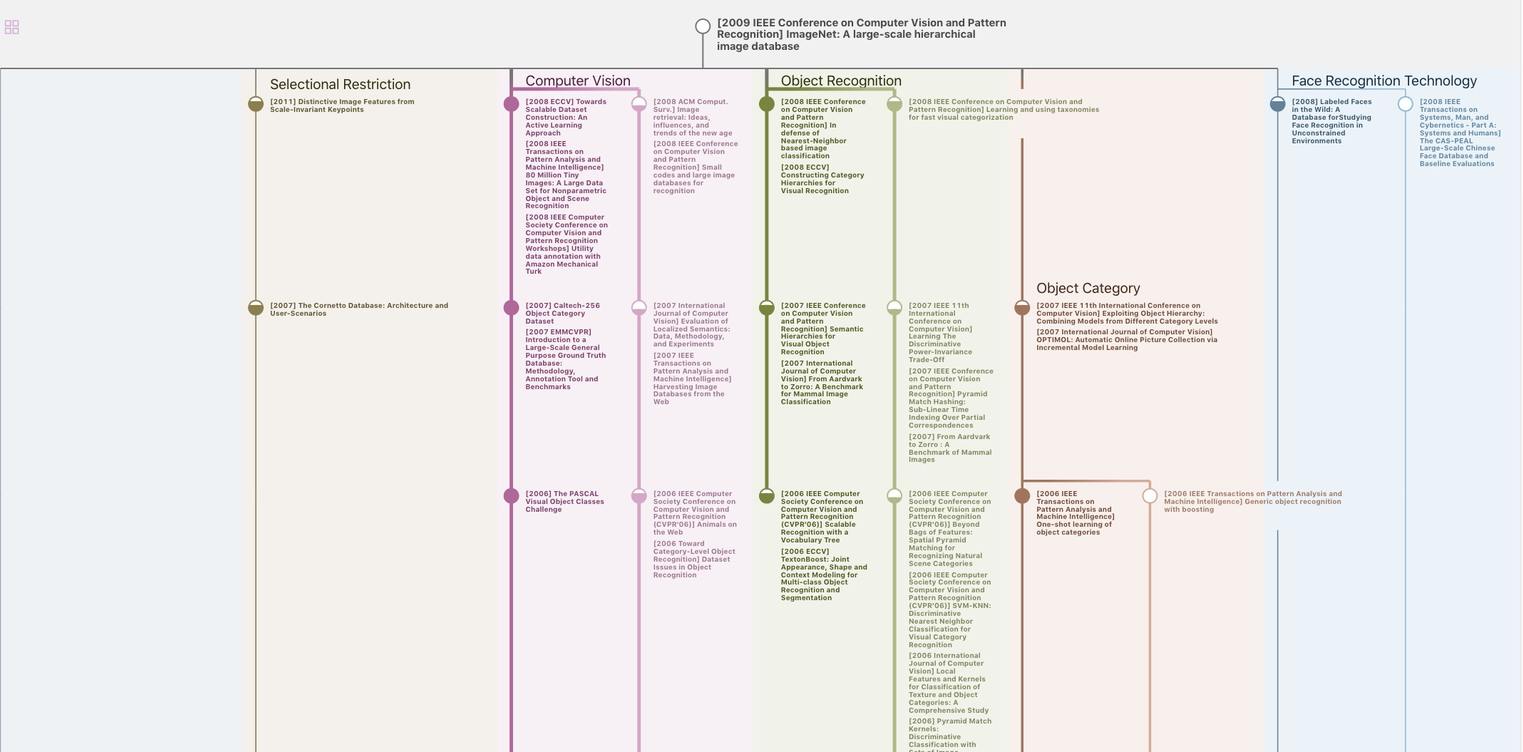
生成溯源树,研究论文发展脉络
Chat Paper
正在生成论文摘要