Learning dynamic background for weakly supervised moving object detection
Image and Vision Computing(2022)
Abstract
Moving Object Detection (MOD) aims at extracting foreground moving objects in videos from static cameras. While low-rank based approaches have achieved impressive success in the MOD task, their performance remains limited on dynamics background scenes. The main reason is that dynamic clutters, e.g., swaying leaves and rippers, are easy to mix up with moving objects in the decomposition model which simply classify the sparse noise as foregrounds. In order to improve the generalization ability of low-rank based moving object detectors, we suggest adding an explicit dynamic clutter component in the decomposition framework with realistic dynamic background modeling. Then the dynamic clutter can be learned through object-free video data due to their self-similarity across time and space. Thus, the moving objects can be naturally separated by a tensor-based decomposition model which formulates the static background by a unidirectional low-rank tensor, learns the dynamic clutter by a two-stream neural network, and constrains moving objects with spatiotemporal continuity. To further provide a more accurate object detection result, an objectness prior is embedded into our model in an attention manner. Extensive experimental results on the challenging datasets of dynamic background clearly demonstrate the superior performance of our model over the state-of-the-art in terms of quantitative metrics and visual quality.
MoreTranslated text
Key words
Moving object detection,Dynamic background,Data-driven discriminative prior,Low-rank framework
AI Read Science
Must-Reading Tree
Example
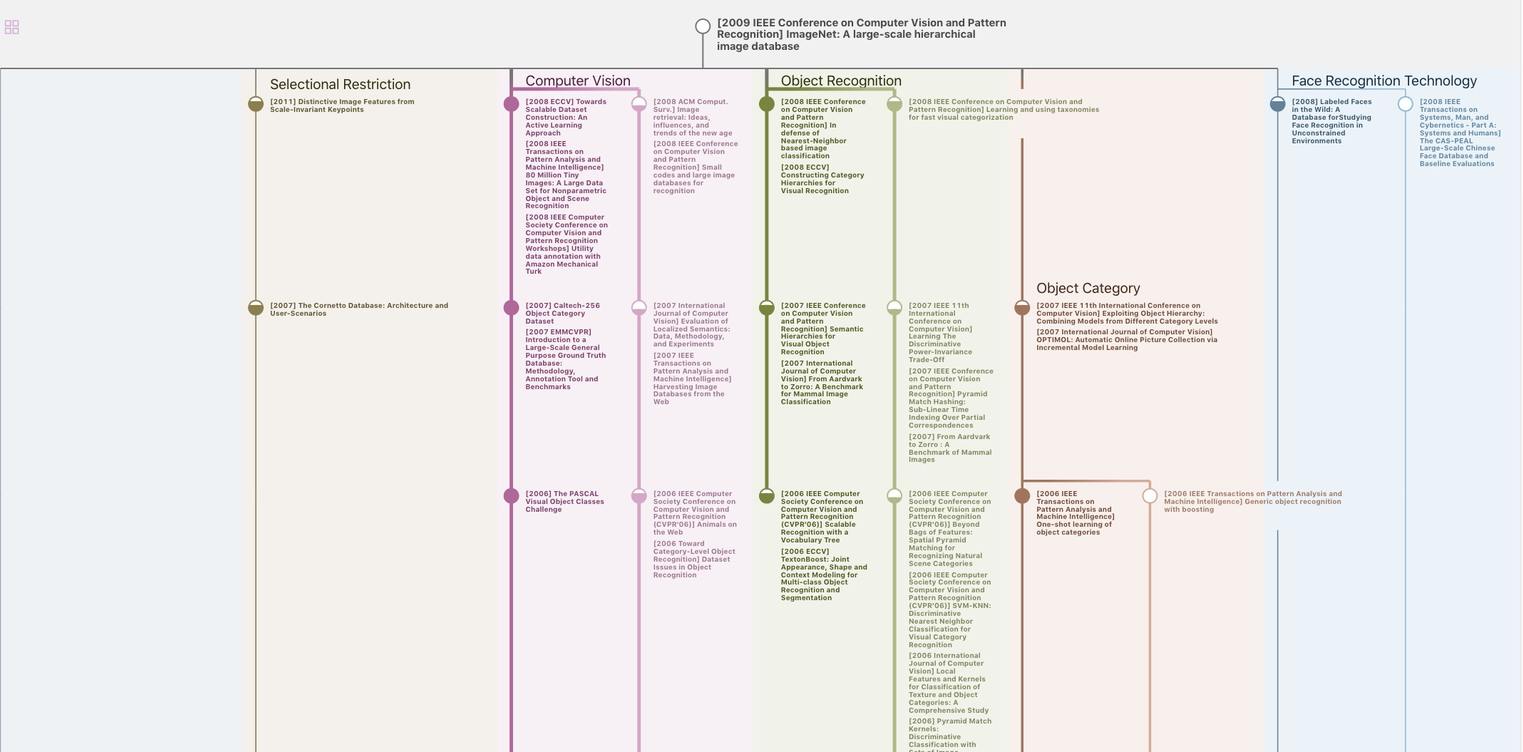
Generate MRT to find the research sequence of this paper
Chat Paper
Summary is being generated by the instructions you defined