Reversible watermarking based on extreme prediction using modified differential evolution
Applied Intelligence(2022)
Abstract
Prediction-error expansion (PEE) is an important technique for reversible watermarking (RW). Traditional PEE methods exploit pixel correlations by modifying prediction-error histogram (PEH) with Laplacian-like distributions, but they rarely consider the influence of needless shifting on image distortion. Asymmetric PEE approaches utilize skewed PEHs to select fewer shifted pixels for superior performance in capacity-distortion control tasks. In this way, the construction of a suitable extreme predictor is especially useful for increasing embedding capacity and reducing image distortion to satisfy user requirements. However, in recent works, the parameters of extreme predictors were not fully optimized. This paper presents a novel extreme predictor, in which the prediction parameters are optimized by using a differential evolution (DE) algorithm to increase the performance of asymmetric PEE by building a sharp and skewed PEH. Specifically, we develop a new DE algorithm based on hybrid mutation and grouping crossover operations to further improve the optimization accuracy of the algorithm. Then, an RW scheme based on the proposed predictor and image separation is built to fully exploit the embedding potential of different bit-planes in images. To reduce image distortion, a strategy that is complementary to double-embedding is presented for lower bit-plane (LBP) embedding. Experimental results demonstrate that the proposed scheme can achieve better image quality and a larger embedding capacity than similar state-of-the-art methods.
MoreTranslated text
Key words
Reversible watermarking,Differential evolution,Prediction-error expansion,Extreme prediction,Bit-plane
AI Read Science
Must-Reading Tree
Example
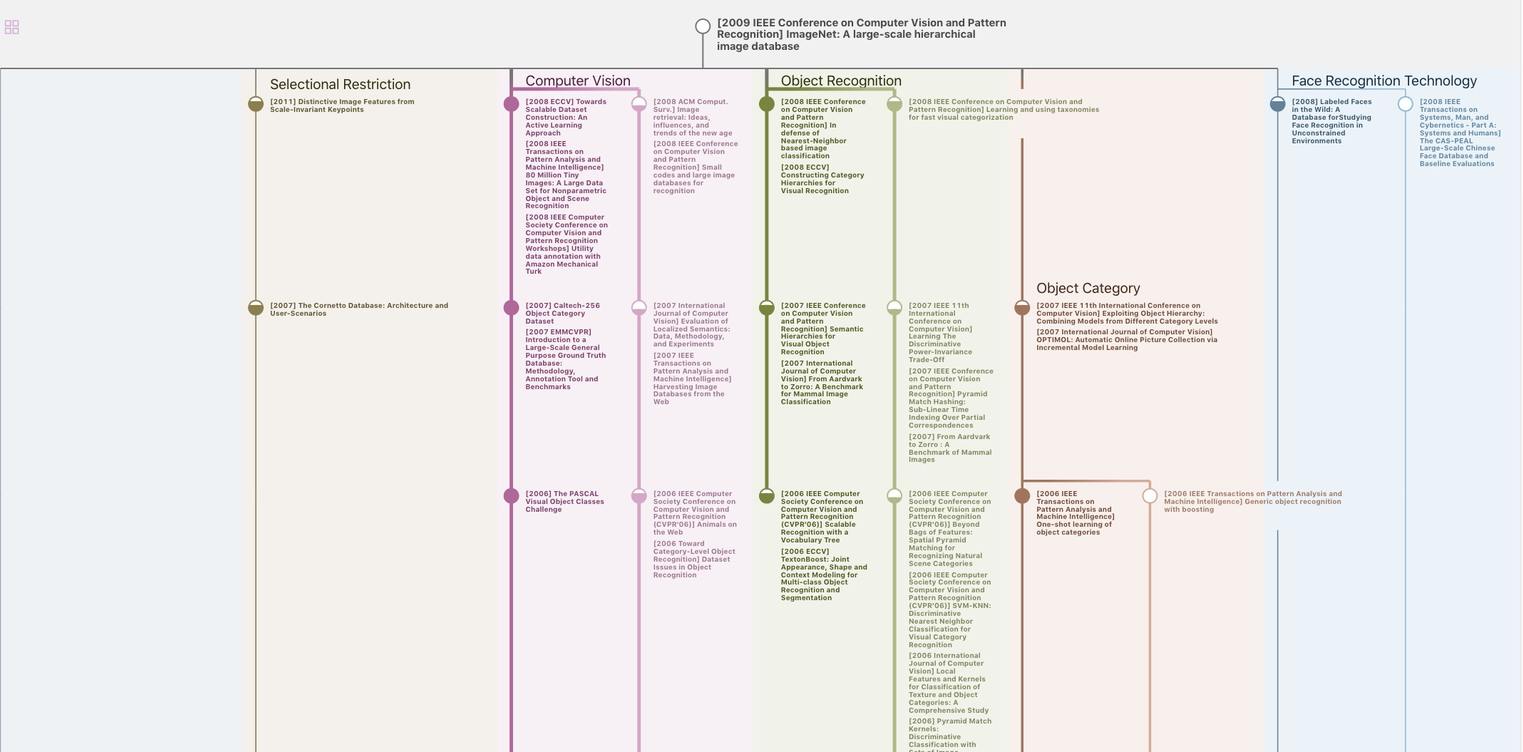
Generate MRT to find the research sequence of this paper
Chat Paper
Summary is being generated by the instructions you defined