Bayesian Attribute Bagging-Based Extreme Learning Machine for High-Dimensional Classification and Regression
ACM TRANSACTIONS ON INTELLIGENT SYSTEMS AND TECHNOLOGY(2022)
摘要
This article presents a Bayesian attribute bagging-based extreme learning machine (BAB-ELM) to handle high-dimensional classification and regression problems. First, the decision-making degree (DMD) of a condition attribute is calculated based on the Bayesian decision theory, i.e., the conditional probability of the condition attribute given the decision attribute. Second, the condition attribute with the highest DMD is put into the condition attribute group (CAG) corresponding to the specific decision attribute. Third, the bagging attribute groups (BAGs) are used to train an ensemble learning model of extreme learning machines (ELMs). Each base ELM is trained on a BAG which is composed of condition attributes that are randomly selected from the CAGs. Fourth, the information amount ratios of bagging condition attributes to all condition attributes is used as the weights to fuse the predictions of base ELMs in BAB-ELM. Exhaustive experiments have been conducted to compare the feasibility and effectiveness of BAB-ELM with seven other ELM models, i.e., ELM, ensemble-based ELM (EN-ELM), voting-based ELM (V-ELM), ensemble ELM (E-ELM), ensemble ELM based on multi-activation functions (MAF-EELM), bagging ELM, and simple ensemble ELM. Experimental results show that BAB-ELM is convergent with the increase of base ELMs and also can yield higher classification accuracy and lower regression error for high-dimensional classification and regression problems.
更多查看译文
关键词
Ensemble learning,extreme learning machine,attribute bagging,Bayesian decision,re-substitution entropy
AI 理解论文
溯源树
样例
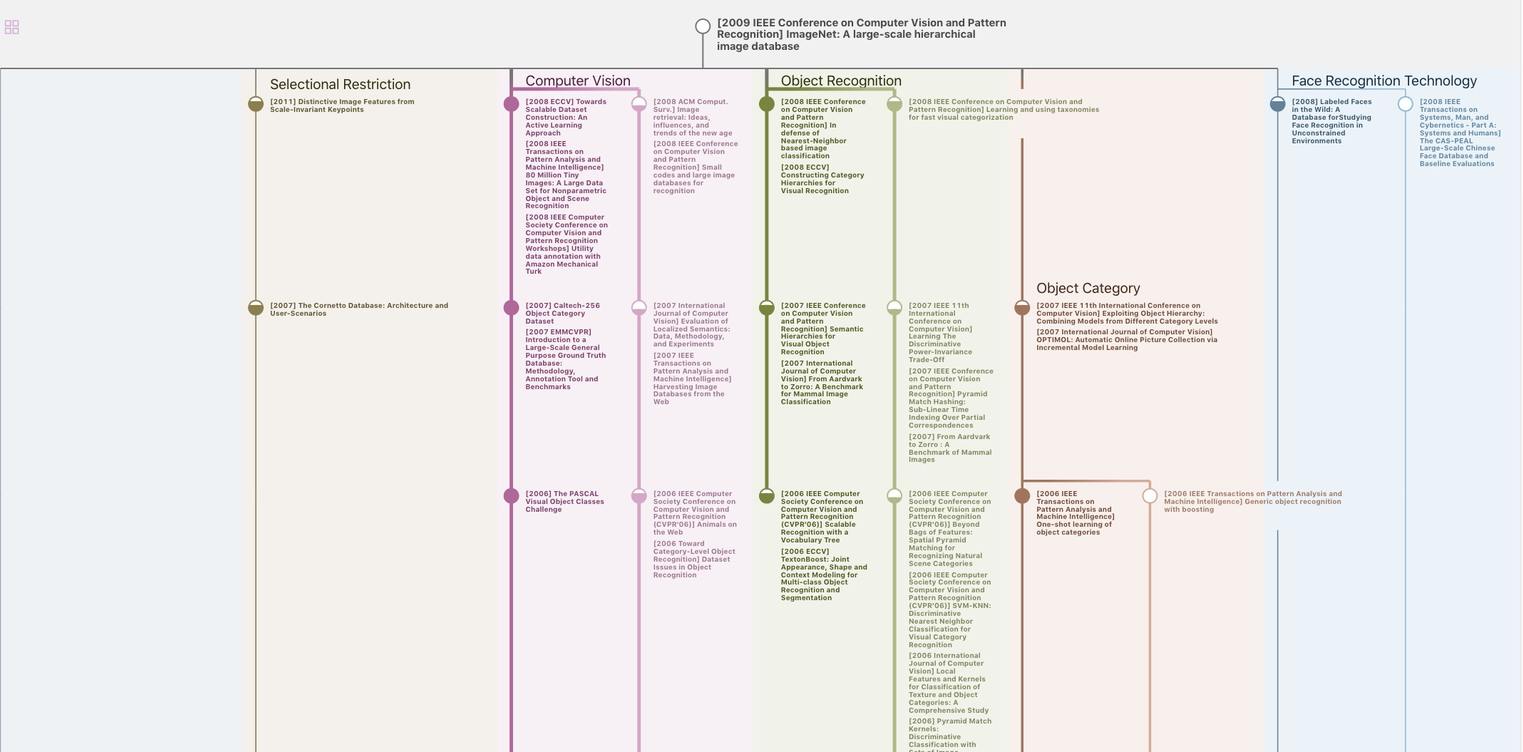
生成溯源树,研究论文发展脉络
Chat Paper
正在生成论文摘要