Mapping Applications Intents to Programmable NDN Data-Planes via Event-B Machines
IEEE ACCESS(2022)
摘要
Location-agnostic content delivery, in-network caching, and native support for multicast, mobility, and security are key features of the novel named data networks (NDN) paradigm. NDNs are ideal for hosting content-centric next-generation applications such as Internet of things (IoT) and virtual reality. Intent-driven management is poised to enhance the performance of the offered NDN services to these applications while reducing its management complexity. This article proposes I2DN, intent-driven NDN, a novel architecture that aims at realizing the first step towards intent modeling and mapping to data-plane configurations for NDNs. In I2DN, network operators and application developers express their abstract and declarative content delivery and network service goals and constraints using uttered or written intents. The intents are classified using built-in intent templates, and a slot filling procedure identifies the semantics of the intent. We then employ Event-B machine (EBM) language modeling to represent these intents and their semantics. The resulting EBMs are then gradually refined to represent configurations at the NDN programmable data-plane. The advantages of the proposed adoption of EBM modeling are twofold. First, EBMs accurately capture the desired behavior of the network in response to the specified intents and automatically refine it into concrete configurations. Second, EBM's formal verification property, referred to as its proof obligation, ensures that the desired properties of the network or its services, as defined by the intent, remain satisfied by the refined EBM representing the final data-plane configurations. Experimental evaluation results demonstrate the feasibility and efficiency of our proposed work.
更多查看译文
关键词
Switches,Metadata,Solid modeling,Licenses,IP networks,Transforms,Semantics,Event-B machines,intent-driven networking,named data networks,programmable data-planes
AI 理解论文
溯源树
样例
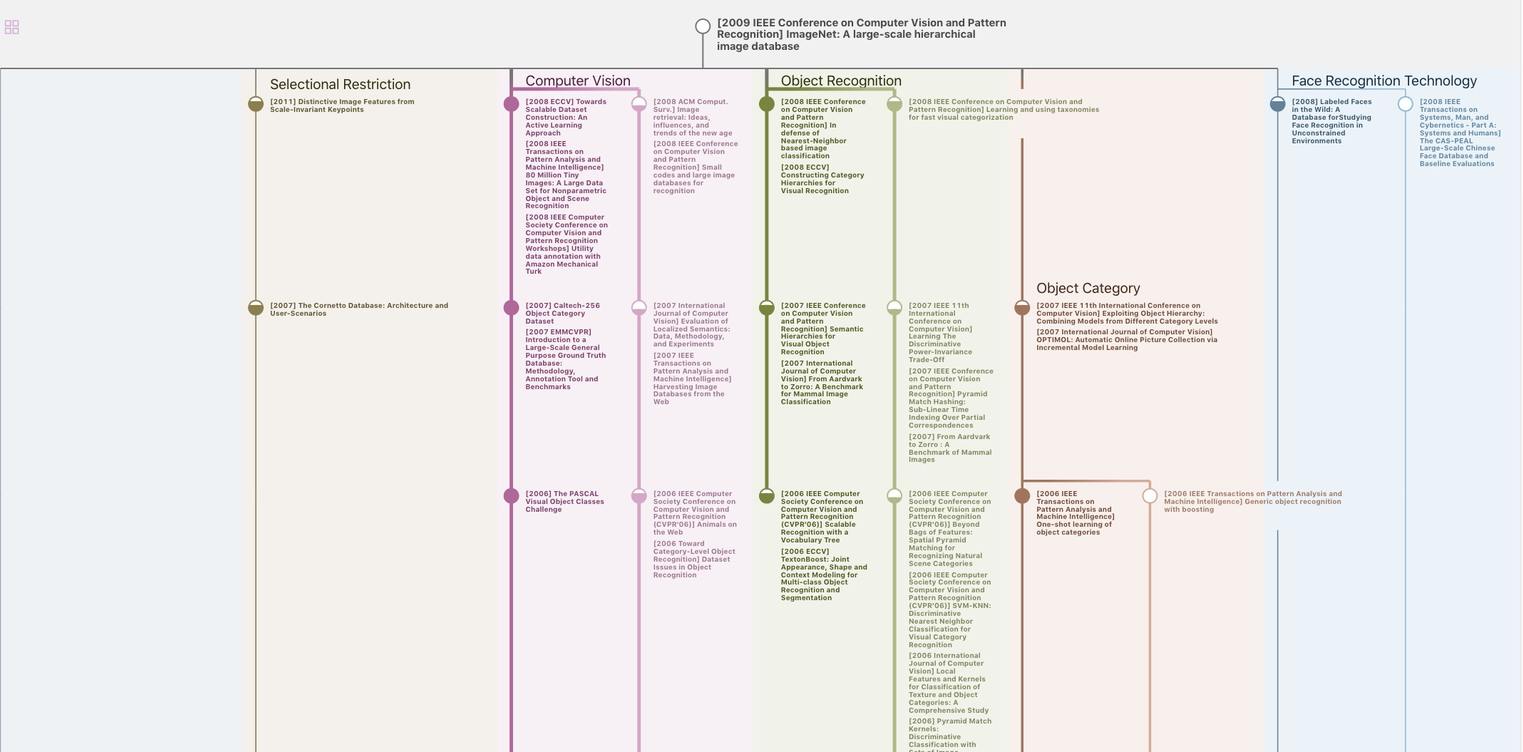
生成溯源树,研究论文发展脉络
Chat Paper
正在生成论文摘要