Differentially expressed genes prediction by multiple self-attention on epigenetics data
BRIEFINGS IN BIOINFORMATICS(2022)
摘要
Predicting differentially expressed genes (DEGs) from epigenetics signal data is the key to understand how epigenetics controls cell functional heterogeneity by gene regulation. This knowledge can help developing 'epigenetics drugs' for complex diseases like cancers. Most of existing machine learning-based methods suffer defects in prediction accuracy, interpretability or training speed. To address these problems, in this paper, we propose a Multiple Self-Attention model for predicting DEGs on Epigenetic data (Epi-MSA). Epi-MSA first uses convolutional neural networks for neighborhood bins information embedding, and then employs multiple self-attention encoders on different input epigenetics factors data to learn which locations of genes are important for predicting DEGs. Next it trains a soft attention module to pick out which epigenetics factors are significant. The attention mechanism makes the model interpretable, and the pure matrix operation of self-attention enables the model to be parallel calculated and speeds up the training. Experiments on datasets from the Roadmap Epigenome Project and BluePrint Data Analysis Portal (BDAP) show that the performance of Epi-MSA is better than existing competitive methods, and Epi-MSA also has a smaller standard deviation, which shows that Epi-MSA is effective and stable. In addition, Epi-MSA has a good interpretability, this is confirmed by referring its attention weight matrix with existing biological knowledge.
更多查看译文
关键词
epigenetics,DNA methylation,histone modification,differentially expressed genes,self-attention
AI 理解论文
溯源树
样例
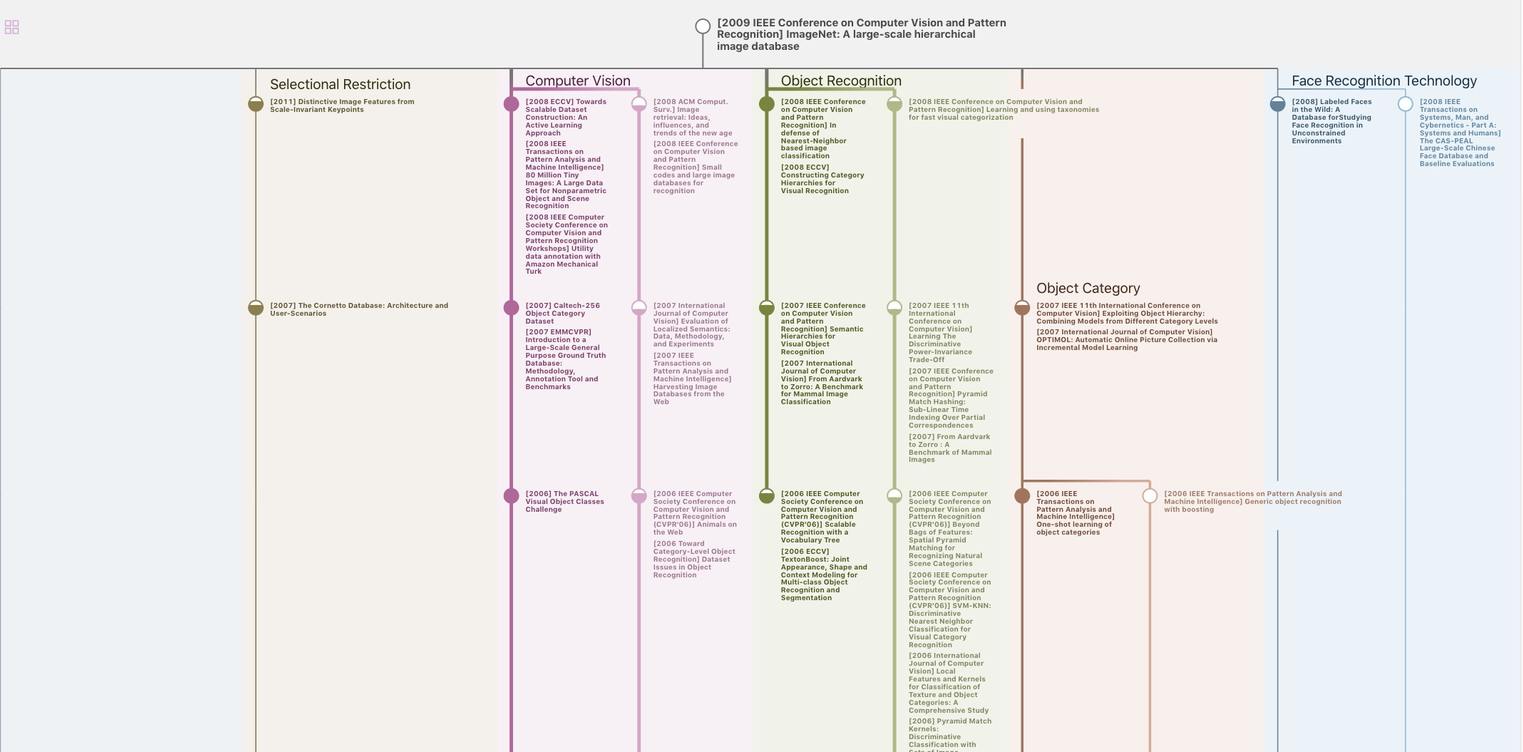
生成溯源树,研究论文发展脉络
Chat Paper
正在生成论文摘要