Systematic Review of Machine Learning Approaches for Detecting Developmental Stuttering
IEEE/ACM Transactions on Audio, Speech, and Language Processing(2022)
摘要
A systematic review of the literature on statistical and machine learning schemes for identifying symptoms of developmental stuttering from audio recordings is reported. Twenty-seven papers met the quality standards that were set. Comparison of results across studies was not possible because training and testing data, model architecture and feature inputs varied across studies. The limitations that were identified for comparison across studies included: no indication of application for the work, data were selected for training and testing models in ways that could lead to biases, studies used different datasets and attempted to locate different symptom types, feature inputs were reported in different ways and there was no standard way of reporting performance statistics. Recommendations were made about how these problems can be addressed in future work on this topic.
更多查看译文
关键词
Developmental stuttering,automatic speech recognition,machine learning,Vapnik–Chervonenkis dimension,language diversity
AI 理解论文
溯源树
样例
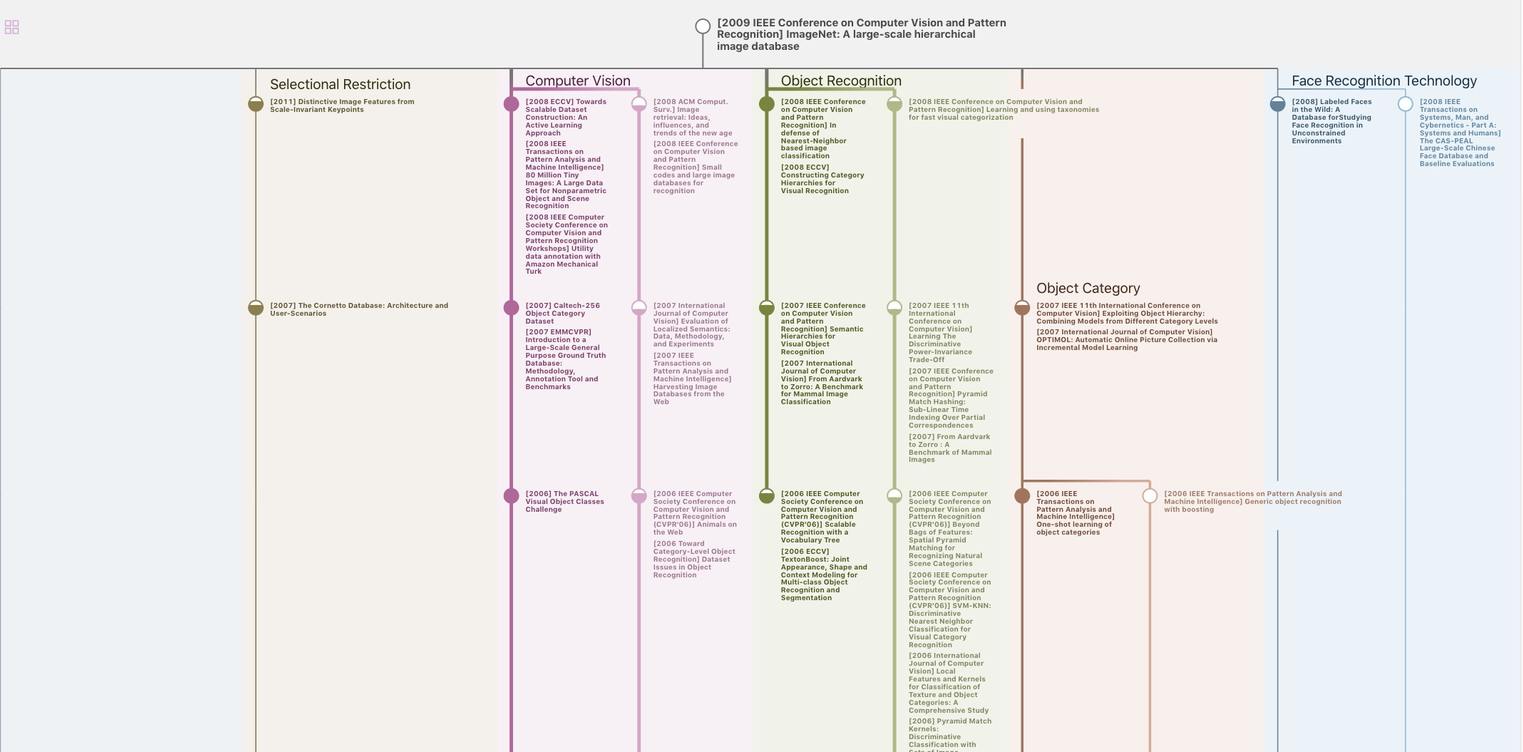
生成溯源树,研究论文发展脉络
Chat Paper
正在生成论文摘要