Abstract P4-11-01: Development of a clinico-bio-behavioral model for cancer-related fatigue (CRF) incorporating inflammatory biomarkers and proteomic data
Cancer Research(2022)
摘要
Abstract Background: We previously developed a clinico-behavioral model of CRF and reported an increased risk of severe CRF among survivors of breast cancer (BC) receiving adjuvant hormonal therapy (HT) (Di Meglio A, ASCO 2021). We now aim to comprehensively explore the contribution of relevant serum proteins in explaining CRF. We adopted a multimodal approach, both (1) hypothesis-driven, based on the rationale that deregulation of systemic inflammatory processes and mediators of immunologic or neuroendocrine activation are associated with vulnerability to CRF, and (2) discovery-driven, based on proteomic analyses. Methods: Women with stage I-III HR+/HER2- tumors receiving HT (N=1153) were included from the multicenter, prospective CANTO cohort (NCT01993498). The primary outcome of interest was severe post-treatment global CRF at year-2 (Y2) after diagnosis (score ≥ 40/100, EORTC QLQ-C30). Secondary outcomes included CRF dimensions (physical, emotional, cognitive; EORTC QLQ-FA12). For the hypothesis-driven analyses, pre-treatment blood samples were profiled (Randox Laboratories Limited, UK) at diagnosis of BC, using a multi-biomarker panel assessing IL6, TNFα, IL1RA, CRP, IL2, IL1β, IFNγ, IL10, IL1A, IL4, and IL8. Pre-specified pre-treatment clinico-behavioral covariates (age, BMI, smoking status, psychological, and pre-treatment symptom burden, based on previously developed models) were forced into a multivariable logistic regression. Biomarkers were retained by Augmented Backwards Elimination (p<0.05) only if significantly associated with CRF. For the discovery approach, we used hyper-reaction monitoring mass spectrometry for the unbiased quantification of all detectable peptides and proteins in human plasma samples at diagnosis (Biognosys, CH), among a discovery subset (N=462). We then aimed to identify a proteomic signature associated with severe CRF at Y2. Log-transformed protein intensities were analyzed in terms of differential expression. The proteins that were identified to be significantly different among the patients reporting and not reporting severe CRF were then used to train a logistic regression model. Results: Prevalence of severe global CRF increased from 21.6% at diagnosis to 34.8% at Y2. In the final model, higher pre-treatment levels of IL6 and lower levels of IFNγ and IL10 were significant predictors of severe global CRF at Y2 (Table). The AUC of this clinico-bio-behavioral model was 0.78 (95%CI 0.75 - 0.82) and was suggestive of an improved performance as compared to clinico-behavioral models. Among CRF dimensions, a significant association emerged only between CRP and severe cognitive CRF (outcome prevalence at Y2 14.2%; adjusted OR per CRP log-unit increase 1.40 [95%CI 1.01-1.93]). In the discovery subset, several proteins were identified as differentially regulated (p<0.05) among patients reporting and not reporting severe CRF at Y2. Most of these were related to coagulation pathways (including C4BPA, C4BPB, HABP2, PLF4, PROS). However, models incorporating proteomic data did not seem to augment the predictive ability compared to clinico-behavioral models. Conclusions: Using clinical and biological pre-treatment measurements, it may be possible to identify a subset of BC patients at high risk for increased post-treatment CRF while on HT. This provides the possibility of testing dedicated preventive interventions. Table. Clinico-bio-behavioral model of pre-treatment predictors of severe global CRF at Y2, incorporating circulating inflammatory biomarkers.Adjusted OR§ (95% CI)Age, per additional 1 year0.98 (0.96-0.99)BMI, per additional unit1.02 (0.99-1.06)Current smoker, vs never2.27 (1.47-3.51)Former smoker, vs never0.97 (0.64-1.46)Anxiety case*, vs normal1.13 (0.75-1.70)Doubtful anxiety*, vs normal1.11 (0.73-1.68)Pre-treatment Insomnia**, per additional 10 points1.09 (1.04-1.15)Pre-treatment Pain**, per additional 10 points1.10 (1.01-1.18)Severe pre-treatment CRF**, vs no4.70 (3.13-7.05)IL6***1.72 (1.25-2.36)IL1RA***1.24 (0.85-1.81)IL2***1.43 (0.99-2.08)IFNγ***0.54 (0.30-0.95)IL10***0.40 (0.18-0.87)IL4***1.47 (0.67-3.20)IL8***1.15 (0.83-1.60)OR= Odds Ratio; CI= Confidence Interval; §by all factors in Table; *HADS; **QLQ-C30; ***per log-unit increase Citation Format: Antonio Di Meglio, Stergios Christodoulidis, Davide Soldato, Antonin Della Noce, Daniele Presti, Julie Havas, Florine Dubuisson, Barbara Pistilli, Valerie Camara-Clayette, Cecile Charles, Patricia A Ganz, Julienne Bower, Ann H Partridge, Alexandra Jacquet, Sibille Everhard, Sandrine Boyault, Fabrice André, Paul-Henry Cournede, Stefan Michiels, Caroline Pradon, Ines Vaz-Luis. Development of a clinico-bio-behavioral model for cancer-related fatigue (CRF) incorporating inflammatory biomarkers and proteomic data [abstract]. In: Proceedings of the 2021 San Antonio Breast Cancer Symposium; 2021 Dec 7-10; San Antonio, TX. Philadelphia (PA): AACR; Cancer Res 2022;82(4 Suppl):Abstract nr P4-11-01.
更多查看译文
AI 理解论文
溯源树
样例
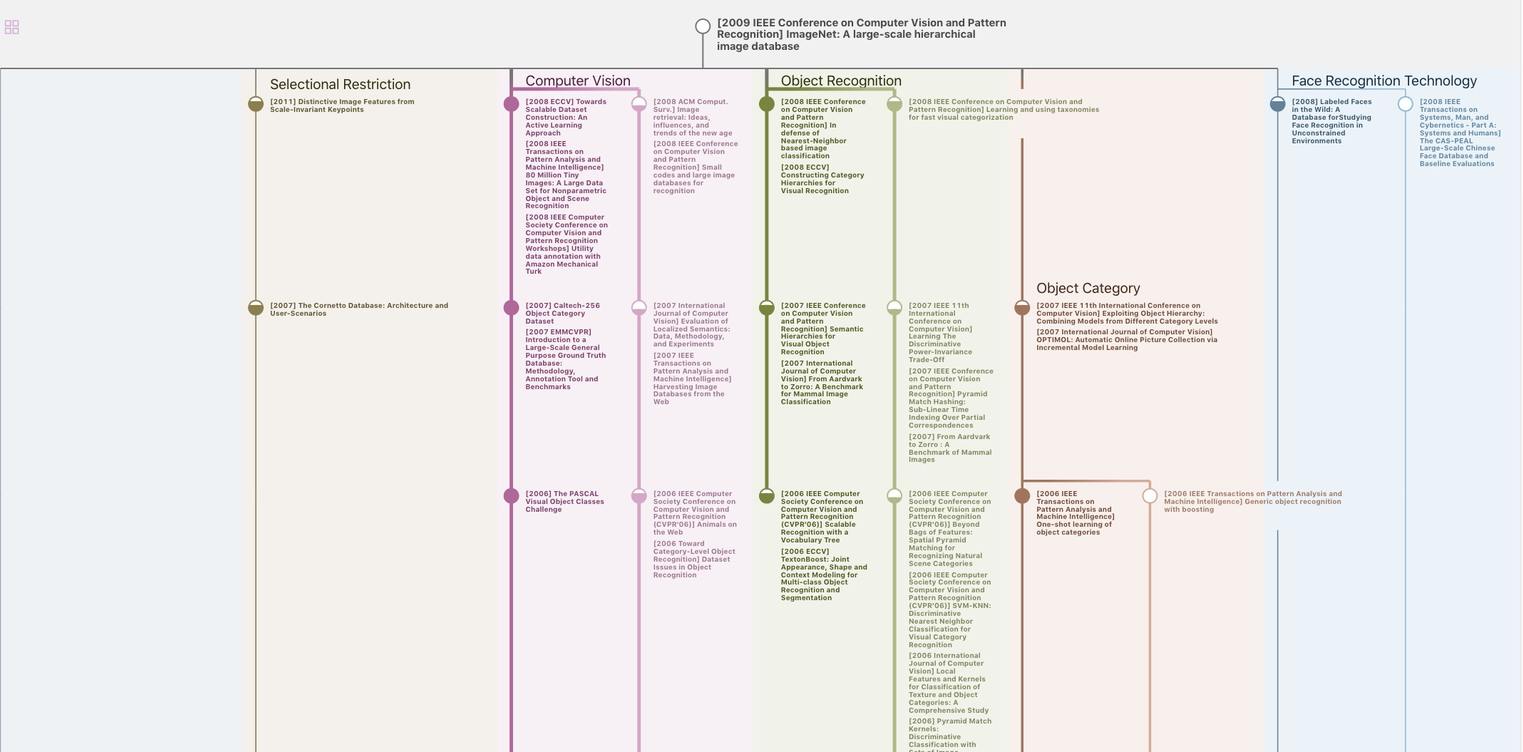
生成溯源树,研究论文发展脉络
Chat Paper
正在生成论文摘要