Deep learning models for multi-class malware classification using Windows exe API Calls
International Journal of Critical Computer-Based Systems(2022)
Abstract
Metamorphic malware is new and one of the most advanced malwares recently discovered. This malware can bypass anti-viruses and are much harder to detect if present in any computer system or network. This research paper intends to develop a better classification method for this metamorphic malware using the latest malware API calls dataset. The multi-class malware classification used in this study is gated recurrent units (GRU). Another non-conventional multi-class malware classification method used is convolution neural network with long short-term memory (CNN + LSTM). The multi-classification results obtained by GRU are 55% with a 0.56 F1-score, and CNN + LSTM is 60% with a 0.61 F1-score, which is quite good. Moreover, the performance of the proposed deep learning models is compared against different classifiers and existing models to show their effectiveness in categorising malware.
MoreTranslated text
Key words
metamorphic malware,gated recurrent unit,GRU,CNN + LSTM,deep learning models,malware classification,Windows exe API calls,SVM,random forest,XGBoost,decision tree
AI Read Science
Must-Reading Tree
Example
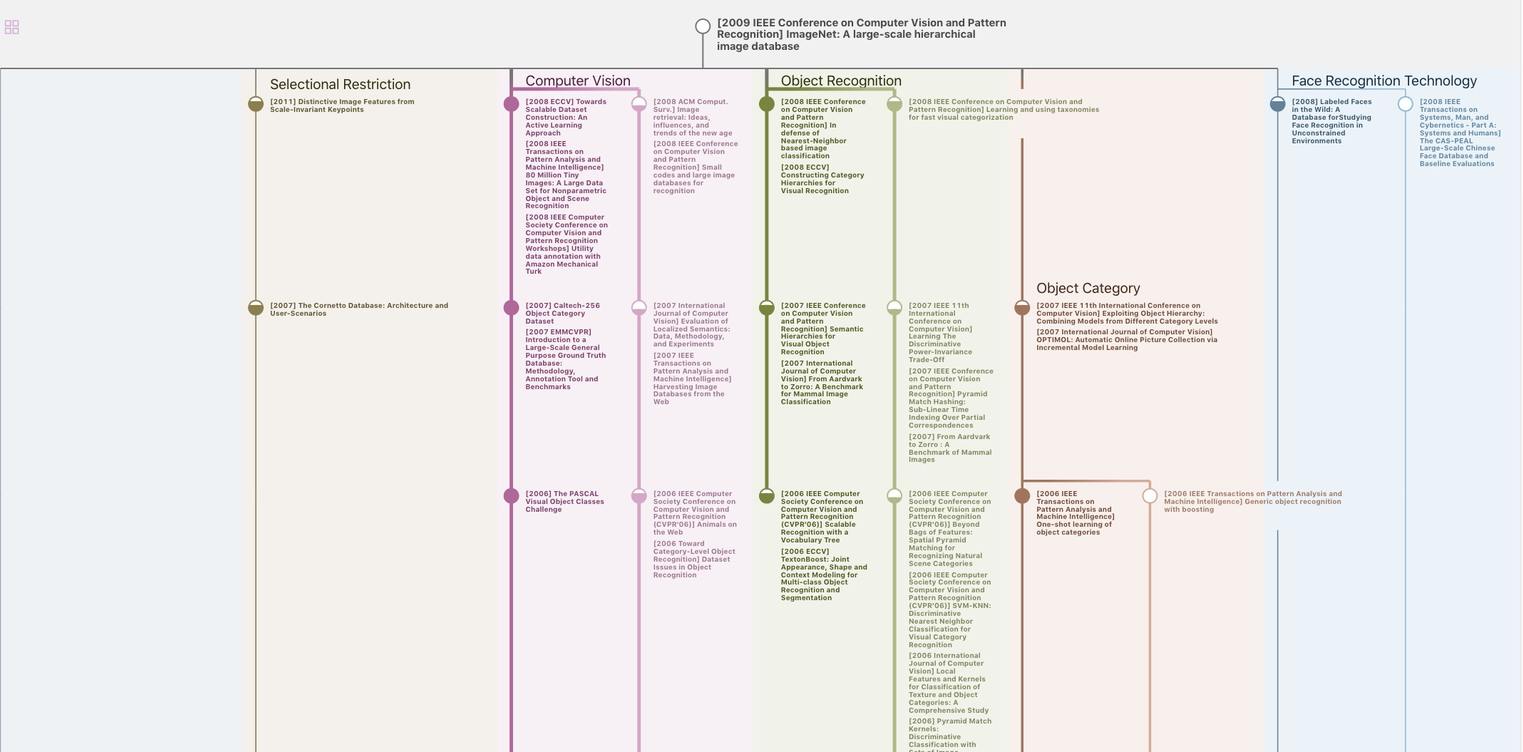
Generate MRT to find the research sequence of this paper
Chat Paper
Summary is being generated by the instructions you defined