Model Reduction of Hypersonic Aerodynamics with residual minimization techniques
AIAA SCITECH 2022 Forum(2022)
Abstract
High-fidelity hypersonic aerodynamic simulations require extensive computational resources, hindering their usage in hypersonic vehicle design and uncertainty quantification. Projection-based reduced-order models (ROMs) are a computationally cheaper alternative to full-order simulations that can provide major speedup with marginal loss of accuracy when solving many-query problems such as design optimization and uncertainty propagation. However, ROMs can present robustness and convergence issues, especially when trained over large ranges of input parameters and/or with few training samples. This paper presents the application of several different residual minimization-based ROMs to hypersonic flows around flight vehicles using less training data than in previous work. The ROM demonstrations are accompanied by a comparison to fully data-driven approaches including kriging and radial basis function interpolation. Results are presented for three test cases including one three-dimensional flight vehicle. We show that registration-based ROMs trained on grid-tailored solutions can compute quantities of interest more accurately than data driven approaches for a given sparse training set. We also find that the classic L2 state error metric is not particularly useful when comparing different model reduction techniques on sparse training data sets.
MoreTranslated text
Key words
hypersonic aerodynamics
AI Read Science
Must-Reading Tree
Example
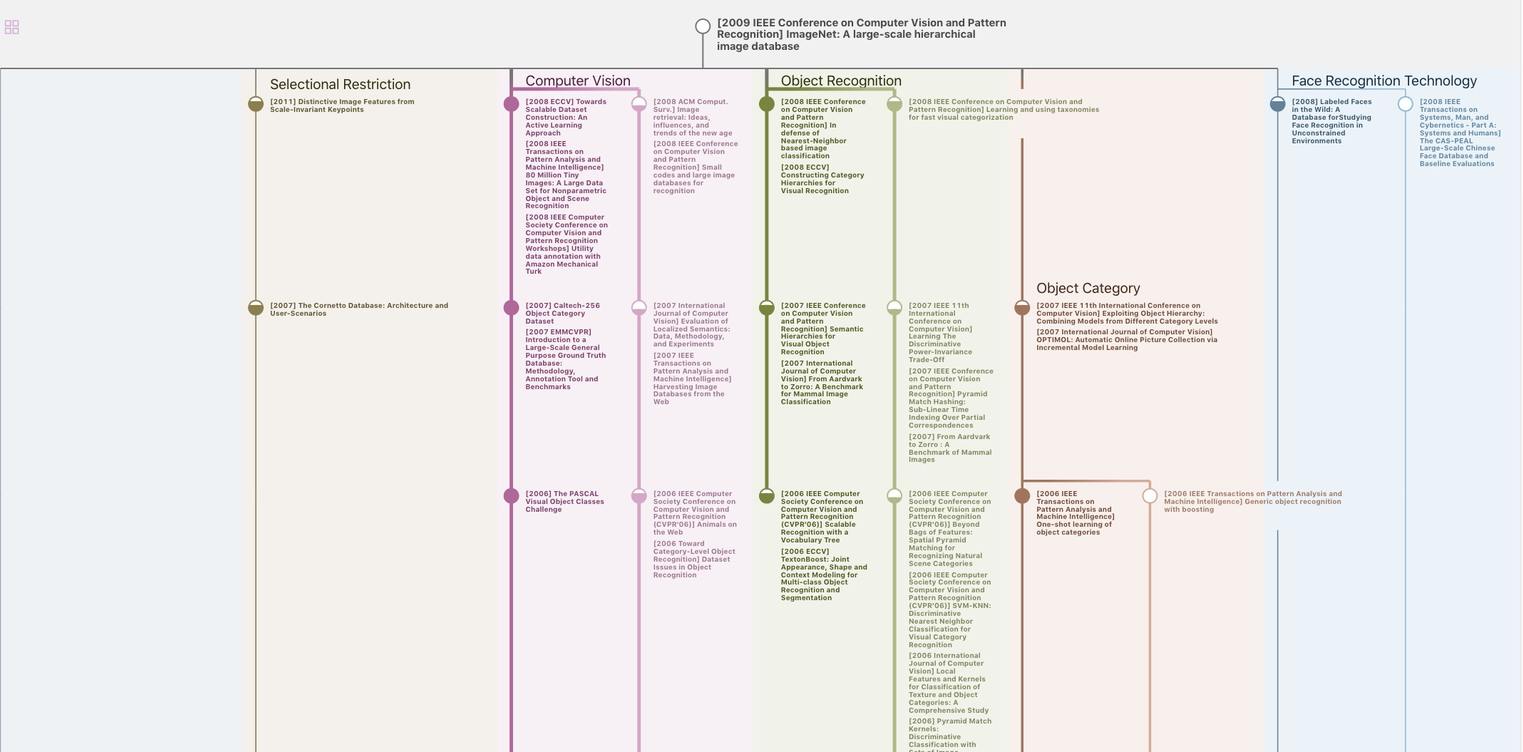
Generate MRT to find the research sequence of this paper
Chat Paper
Summary is being generated by the instructions you defined