Attention Augmented Convolutional Neural Network for Fine-Grained Plant Disease Classification and Visualization Using Stochastic Sample Transformations
2021 5th International Conference on Advances in Image Processing (ICAIP)(2021)
摘要
Deep convolutional neural network (DCNN) image analysis improved plant health management. DCNN methods require train samples with diverse feature distribution and discriminative features for the models to generalize well on unseen categories. The limited train sample coined with the locality nature of the convolution operator and commonly shared kernel weights led CNN models to a sub-optimal solution. To address these challenges, we trained a multi-head self-attention augmented CNN (AAC) and explored other attention augmented networks to jointly exploit global salient and local shared features for fine-grained plant disease classification and visualization. We further proposed stochastic train sample transformations to enlarge and improve train sample diversity and feature distribution. The experiment evaluations on the PlantVillage dataset confirmed that the attention augmentation methods demonstrated robust performance in disease classification and visualization. Specifically, the AAC network scored 99.56% with stochastic transformed train samples, 98.01% with simple geometric transformed samples, and 97.34% without transformation. Therefore, attention augmentation in general, AAC in particular, and stochastic sample transformation can address discriminative plant disease classification and visualization.
更多查看译文
AI 理解论文
溯源树
样例
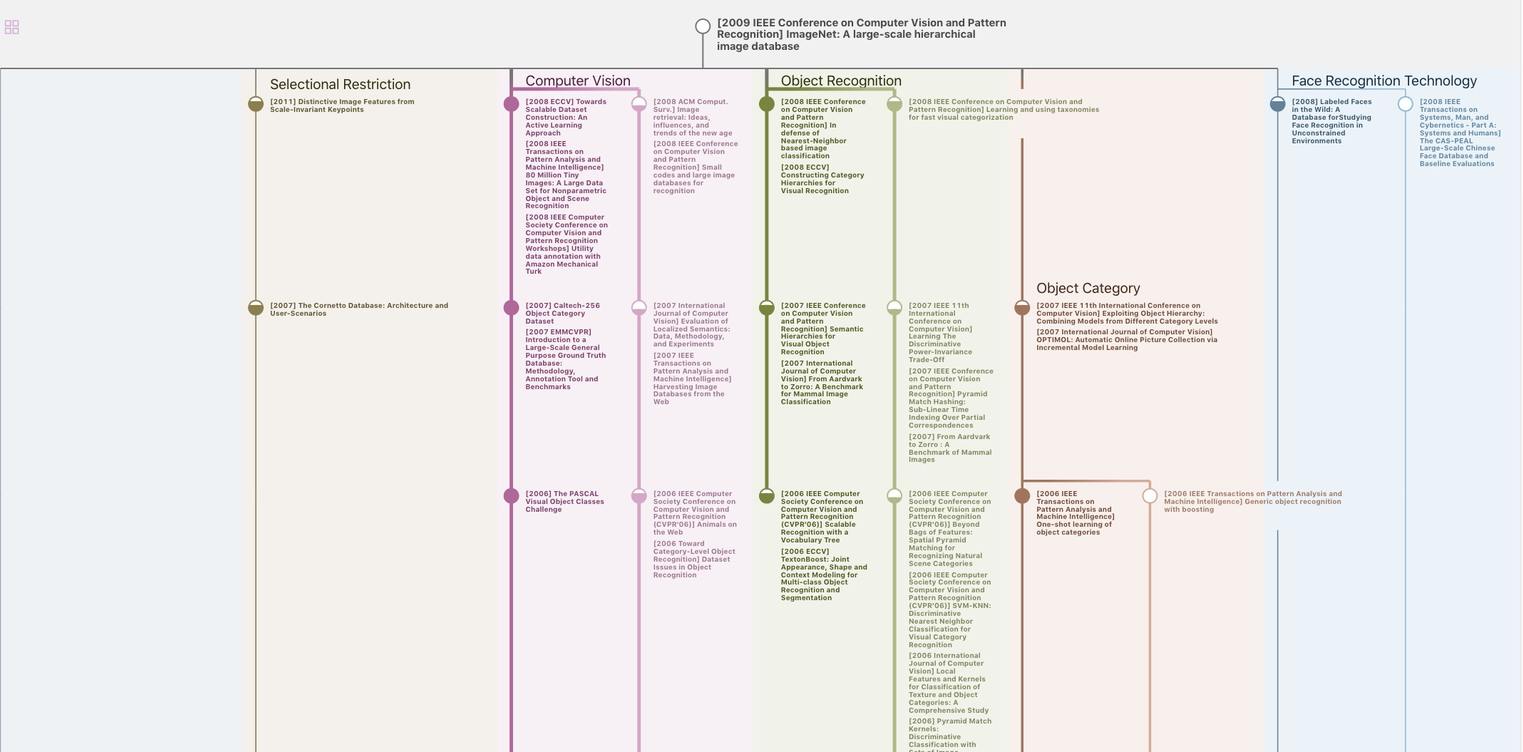
生成溯源树,研究论文发展脉络
Chat Paper
正在生成论文摘要