Generalizable Crowd Counting via Diverse Context Style Learning
IEEE Transactions on Circuits and Systems for Video Technology(2022)
摘要
Existing crowd counting approaches predominantly perform well on the training-testing protocol. However, due to large style discrepancies not only among images but also within a single image, they suffer from obvious performance degradation when applied to unseen domains. In this paper, we aim to design a generalizable crowd counting framework which is trained on a source domain but can generalize well on the other domains. To reach this, we propose a gated ensemble learning framework. Specifically, we first propose a diverse fine-grained style attention model to help learn discriminative content feature representations, allowing for exploiting diverse features to improve generalization. We then introduce a channel-level binary gating ensemble model, where diverse feature prior, input-dependent guidance and density grade classification constraint are implemented, to optimally select diverse content features to participate in the ensemble, taking advantage of their complementary while avoiding redundancy. Extensive experiments show that our gating ensemble approach achieves superior generalization performance among four public datasets. Codes are publicly available at https://github.com/wdzhao123/DCSL.
更多查看译文
关键词
Training,Logic gates,Redundancy,Lighting,Degradation,Mean square error methods,Visualization,Gated ensemble learning,diverse context styles,generalized crowd counting
AI 理解论文
溯源树
样例
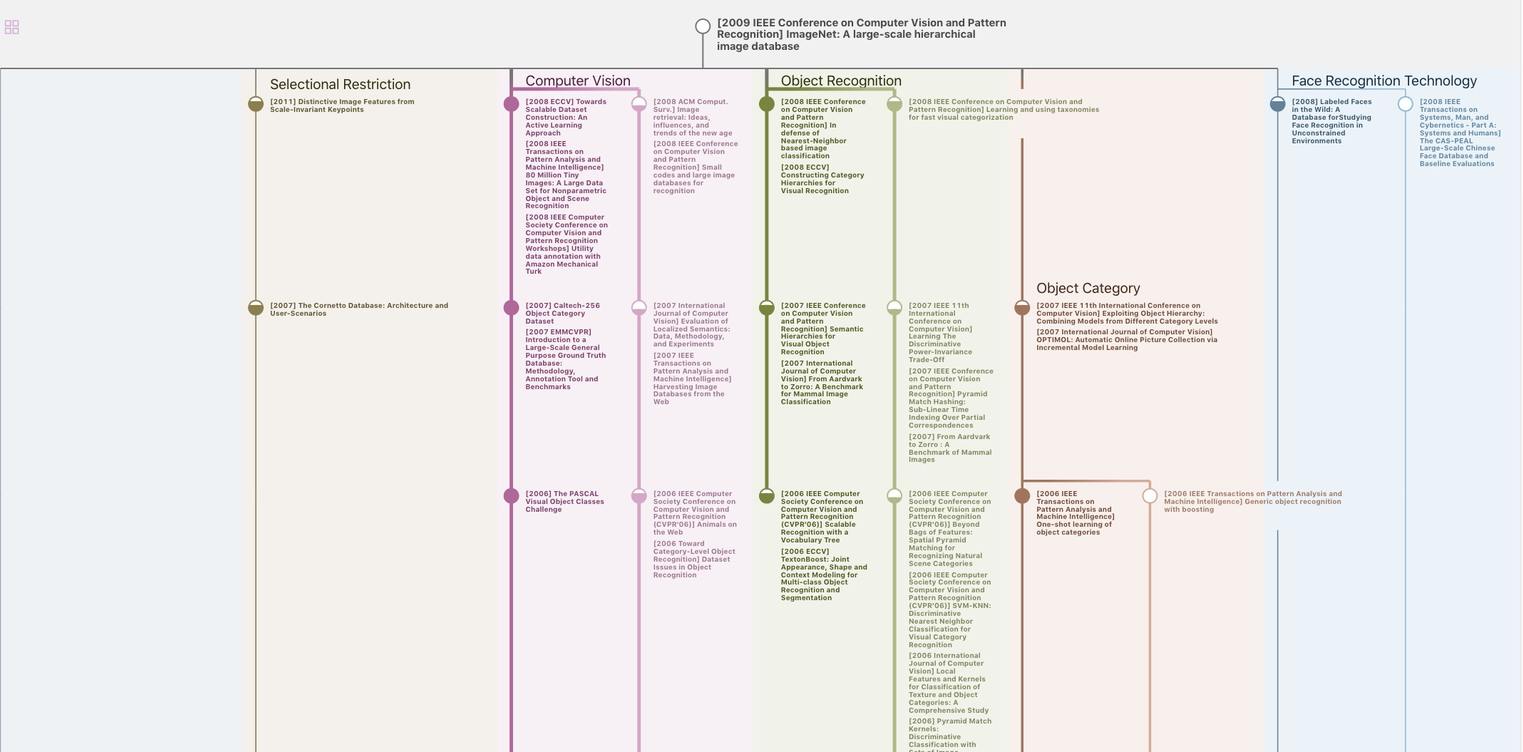
生成溯源树,研究论文发展脉络
Chat Paper
正在生成论文摘要