Augmented Multi-Component Recurrent Graph Convolutional Network for Traffic Flow Forecasting
ISPRS INTERNATIONAL JOURNAL OF GEO-INFORMATION(2022)
摘要
Due to the periodic and dynamic changes of traffic flow and the spatial-temporal coupling interaction of complex road networks, traffic flow forecasting is highly challenging and rarely yields satisfactory prediction results. In this paper, we propose a novel methodology named the Augmented Multi-component Recurrent Graph Convolutional Network (AM-RGCN) for traffic flow forecasting by addressing the problems above. We first introduce the augmented multi-component module to the traffic forecasting model to tackle the problem of periodic temporal shift emerging in traffic series. Then, we propose an encoder-decoder architecture for spatial-temporal prediction. Specifically, we propose the Temporal Correlation Learner (TCL) which incorporates one-dimensional convolution into LSTM to utilize the intrinsic temporal characteristics of traffic flow. Moreover, we combine TCL with the graph convolutional network to handle the spatial-temporal coupling interaction of the road network. Similarly, the decoder consists of TCL and convolutional neural networks to obtain high-dimensional representations from multi-step predictions based on spatial-temporal sequences. Extensive experiments on two real-world road traffic datasets, PEMSD4 and PEMSD8, demonstrate that our AM-RGCN achieves the best results.
更多查看译文
关键词
traffic flow forecasting,spatial-temporal prediction,graph convolutional networks,augmented multi-component
AI 理解论文
溯源树
样例
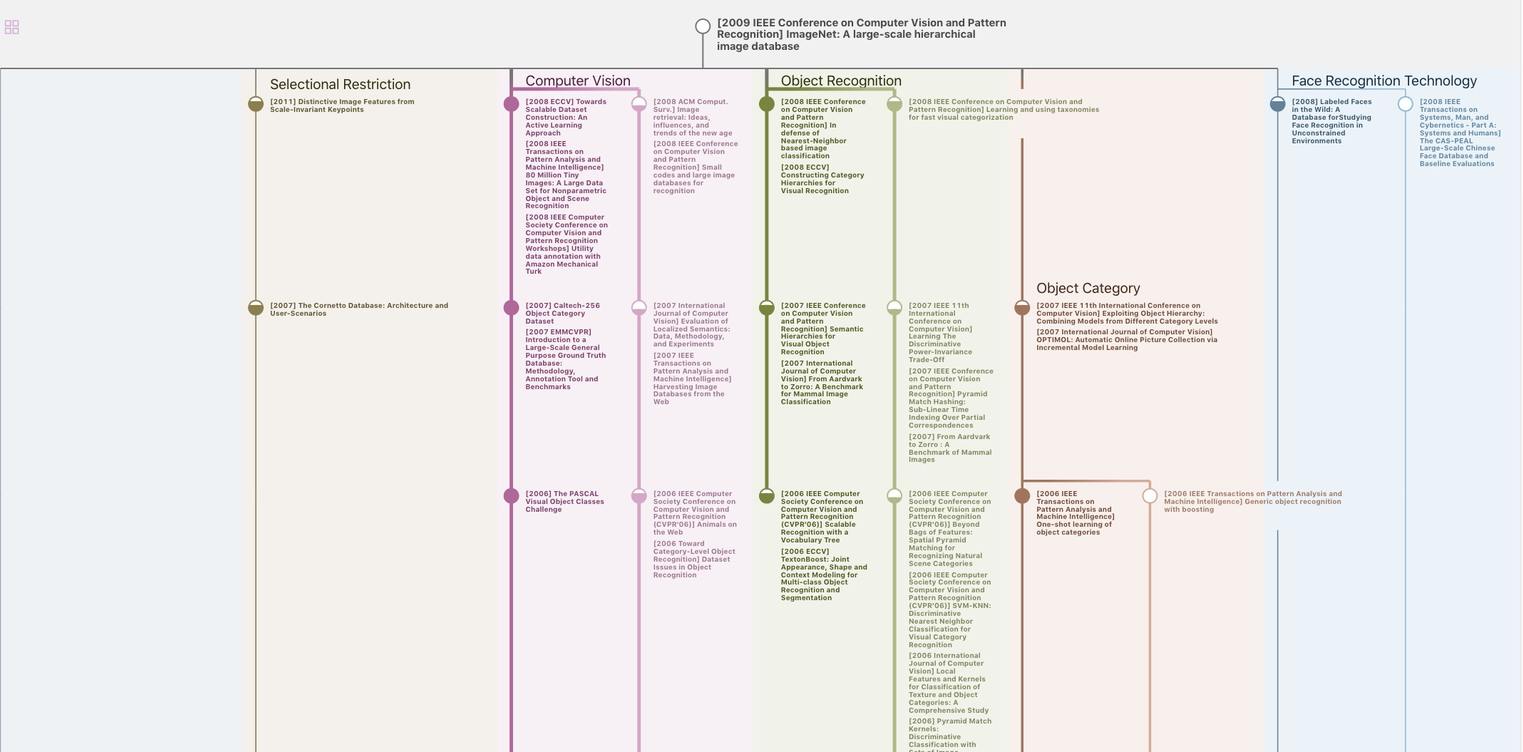
生成溯源树,研究论文发展脉络
Chat Paper
正在生成论文摘要