Predicting steam-gasification output using artificial neural networks
Volume 8A: Energy(2021)
Abstract
Steam gasification has been identified to be one of the most important technologies to produce syngas for biofuel synthesis, due to the high H2 concentration it can reach, which is significantly higher compared with conventional air gasification processes. Double-stage downdraft gasification has proven to be efficient with a secondary steam injection. Various kinds of models, including computational fluid dynamics (CFD), thermo-equilibrium, kinetic rate, and artificial neural network (ANN) have been developed for studying these processes. Equilibrium models assume of chemical reaction equilibrium, in which the transfer phenomenon and the reaction kinetics of primary reactions are considered. In CFD models, the mass, momentum, and energy equations are solved simultaneously along the consideration of the gasifier design to predict parameters such as temperature or species concentrations. To overcome the limitations posed by these models, artificial intelligence tools such as ANNs are reliable for the prediction of nonlinear system data. The application of ANN in gasification processes have been reported by several researchers. ANN based models have been developed for predicting syngas yields and gas composition in air gasification systems. However, to the author's knowledge, no study has focused in the prediction, using ANN, of a double-stage steam gasification system. In the current study, the effect of different temperatures along the gasification process (TO-T7), equivalence ratio, and steam-to-air ratio were studied in an artificial neural network model to predict the gas composition (CO, CH4, and H2) and the lower heating value (LHV) of the syngas. Miscanthus grass is selected as the fuel. The ultimate analysis of the fuel is excluded in the input because the insignificant impact on syngas composition especially H2 production. Feed forward back propagation (FFBP) neural networks were employed for the training of the networks. Then the model with optimal parameters were selected to test against experimental data for validation purposes. Further, the unused data are tested for model accuracy. It was observed that the developed models are able to predict the CO, CH4, H2, and LHV with good accuracy when comparing with experimental data. The FFBP showed excellent performance. A higher coefficient of determination (R2) and lower mean square error (MSE) is achieved by FFBP. The results obtained indicate that ANNs are powerful tools to facilitate system design, operation and control of doublestage downdraft gasifiers with various operating conditions. Utilizing ANN models, one can predict reasonable output parameters without unnecessary failure.
MoreTranslated text
Key words
steam gasification,double-stage gasifier,artificial neural networks (ANNs),feed-forward back propagation (FFBP)
AI Read Science
Must-Reading Tree
Example
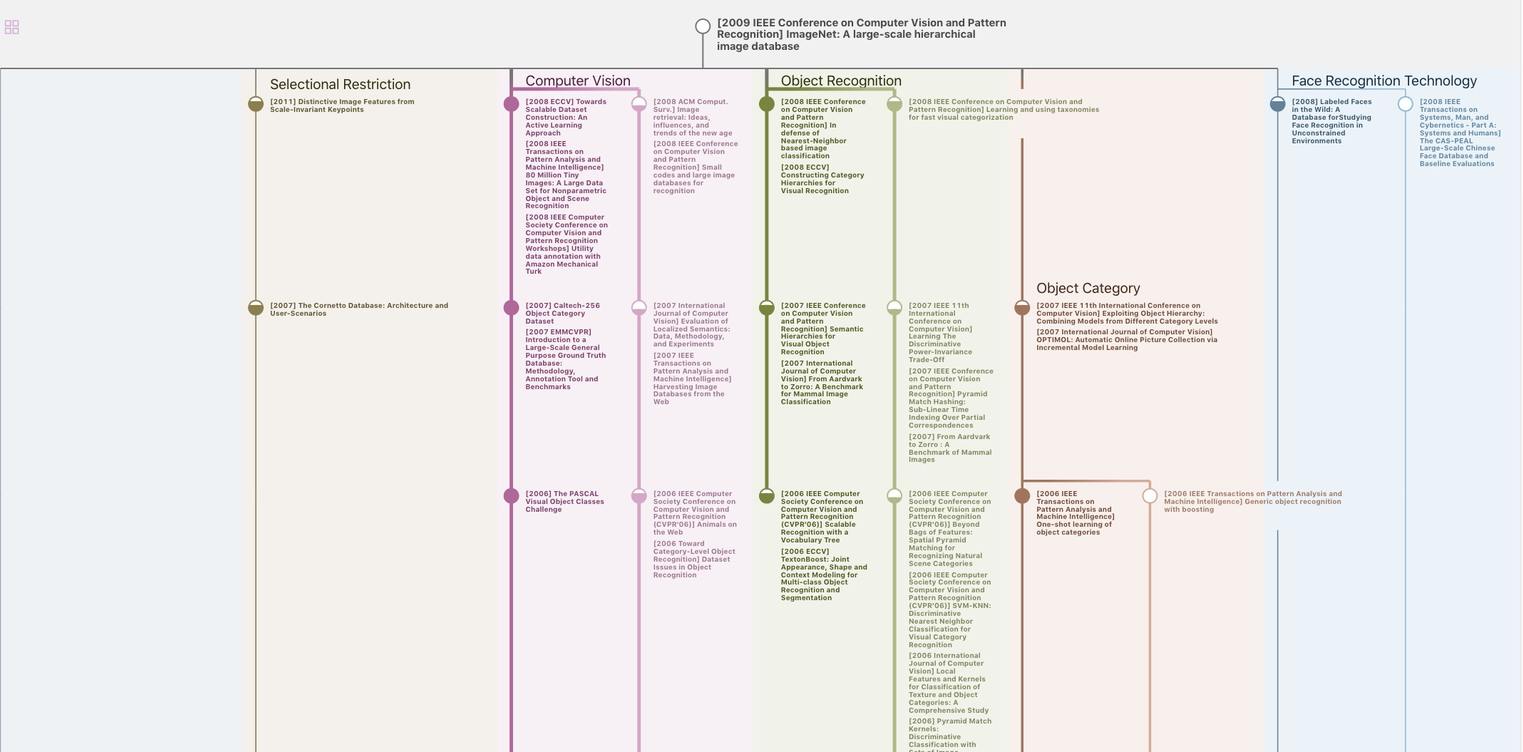
Generate MRT to find the research sequence of this paper
Chat Paper
Summary is being generated by the instructions you defined