Two-stage content based image retrieval using sparse representation and feature fusion
Multimedia Tools and Applications(2022)
摘要
With the advent of large-scale databases in the last two decades, content based image retrieval (CBIR) has been widely investigated. Studies show that the performance of the CBIR system is mainly affected by the image descriptors and the similarity measurement. Therefore, effectively describing the content of an image is a key point in the field of image retrieval. In the present study, a two-stage CBIR algorithm using sparse representation and feature fusion is proposed, in which the global and local features are combined to retrieve the images. The architecture of the CBIR system includes two parts: rough retrieval stage and main retrieval stage. The generalized search tree (GIST) features are initially used to roughly retrieve images with similar scene information by measuring the Canberra distance. Then, sparse coding and feature pooling are used to obtain the sparse representation of the local features extracted from the rough retrieval results. Finally, the Euclidean distance is applied to measure the similarity of the sparse feature vectors to acquire the retrieval results. Compared with the existing single feature-based image retrieval algorithms, experimental results on the Coil20 and Caltech256 image datasets show the best P, R, F1-measure and MAP values. It can be concluded that the proposed method obtains superior retrieval performance.
更多查看译文
关键词
Content based image retrieval,Sparse representation,Feature fusion,Generalized search tree
AI 理解论文
溯源树
样例
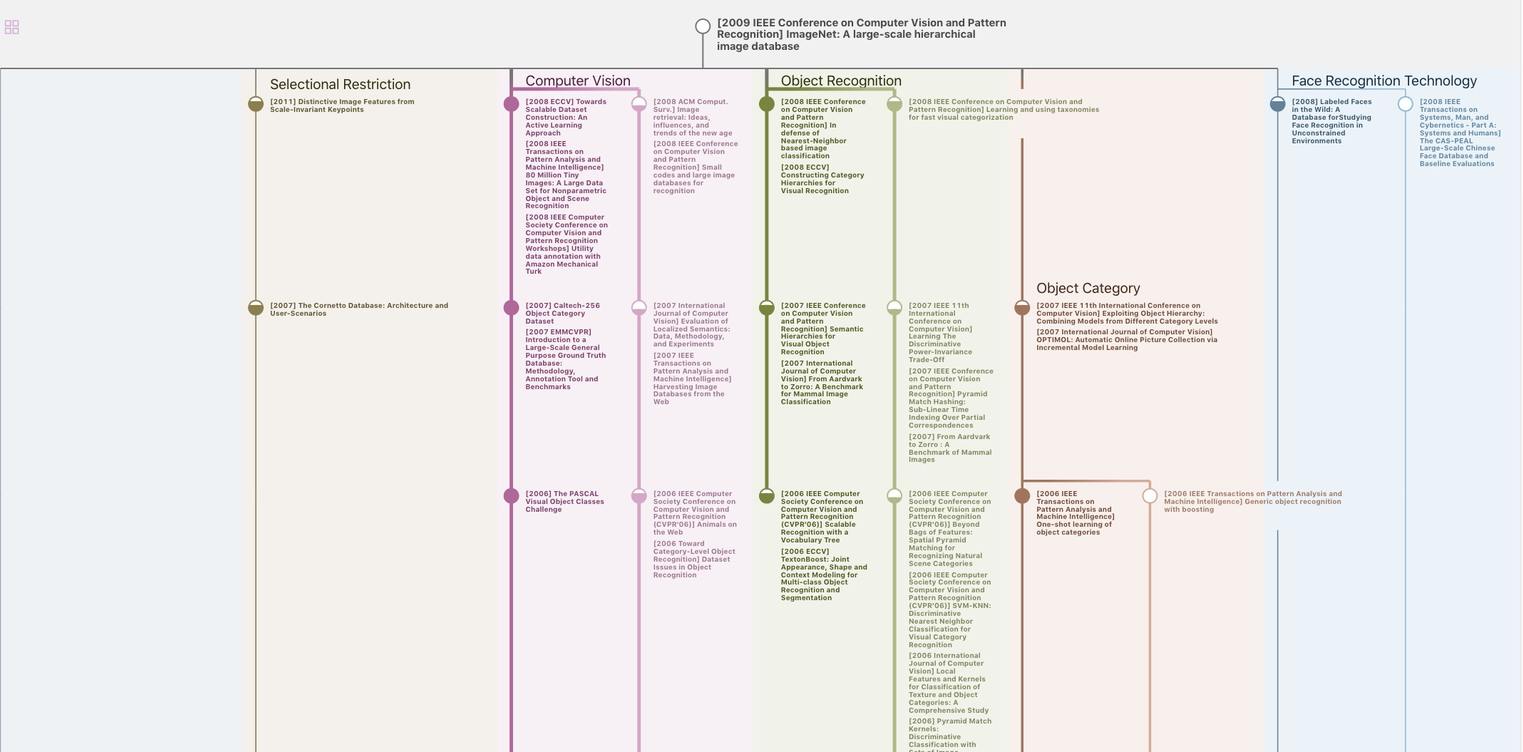
生成溯源树,研究论文发展脉络
Chat Paper
正在生成论文摘要