Noise Reduction of SEM Images using U-net with SSIM Loss Function
Proceedings of the ISCIE International Symposium on Stochastic Systems Theory and its Applications(2021)
摘要
The image-to-image translation networks, such as U-net [1] or Pix2pix [2], are known to be able to convert input images into different images where the image quality is improved or desired semantic information hidden in the input images are extracted. Several types of research based on such image translation networks have been carried out to realize noise removal systems that convert low-quality images taken with a low-performance microscope into high-quality images taken with a high-performance microscope [3,4,5]. In this paper, we develop denoising and deblurring methods to improve the image quality taken by the conventional scanning electron microscope (SEM) as the level of the image quality taken by the field emission (FE) SEM. In order to realize such methods, we utilize Pix2pix and U-net as the image denoiser. We compare the results of each image denoiser qualitatively and quantitatively. We show that the images generated by the conventional U-net [6] are apt to be slightly but entirely blurred, and the generative adversarial networks (GAN) [7] comprising a part of Pix2pix has a risk to inappropriately modify image details. Hence, we propose and evaluate U-net using Structural similarity (SSIM) loss function. We show that SSIM U-net can avoid a slight blur caused by the conventional U-net with fewer falsification than Pix2pix.
更多查看译文
关键词
sem images,u-net
AI 理解论文
溯源树
样例
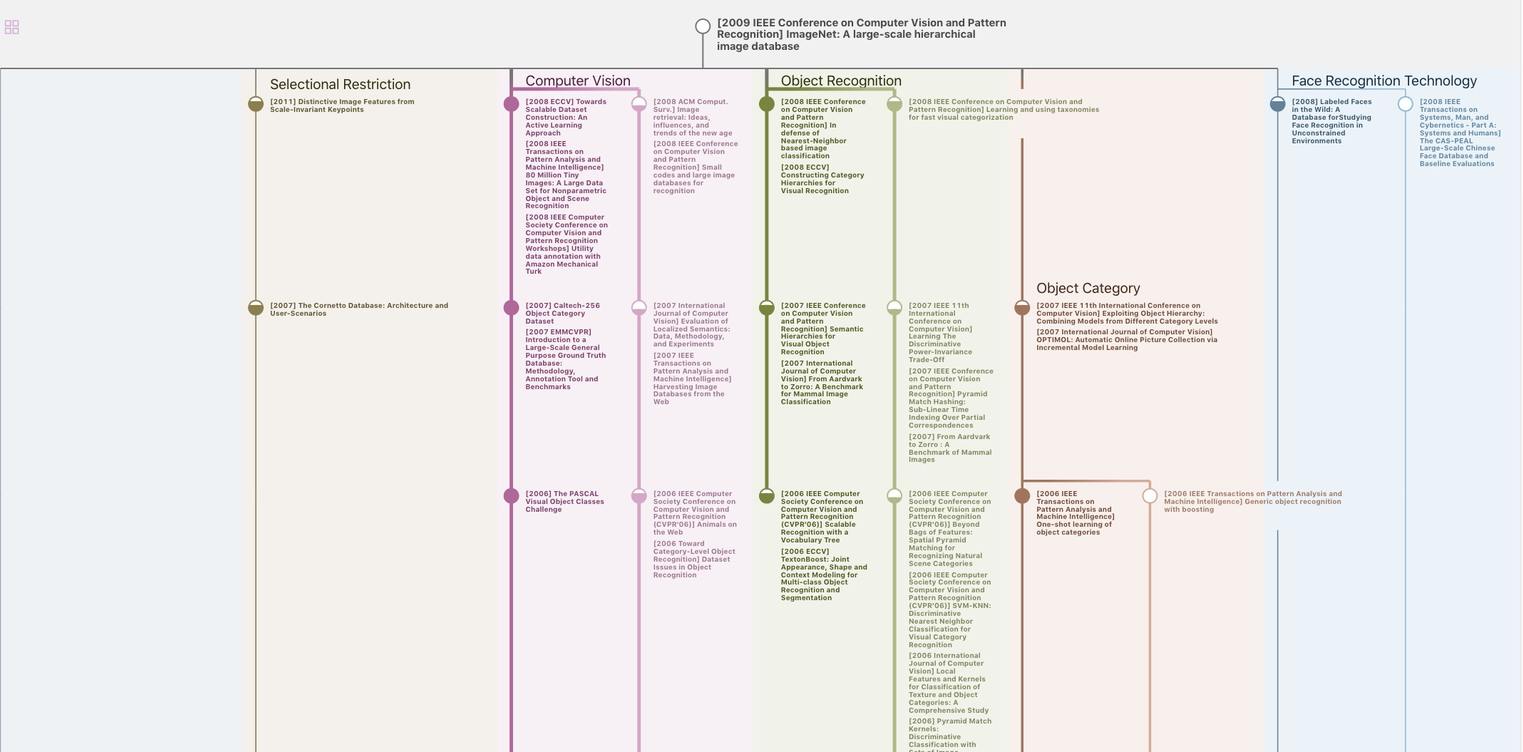
生成溯源树,研究论文发展脉络
Chat Paper
正在生成论文摘要