DIAT-RadHARNet: A Lightweight DCNN for Radar Based Classification of Human Suspicious Activities
IEEE TRANSACTIONS ON INSTRUMENTATION AND MEASUREMENT(2022)
Abstract
Recognizing suspicious human activities is one of the critical requirements for national security considerations. Nowadays, designing the deep convolution neural network (DCNN) models suitable for micro-Doppler (m-D) signature-based human activity classification is rapidly growing. However, high computation cost and a huge number of parameters limit their direct/effective usability in field applications. This article introduces an m-D signatures' dataset DIAT-mu RadHAR covering army crawling, boxing, jumping while holding a gun, army jogging, army marching, and stone-pelting/grenade-throwing, generated using an X-band continuous wave (CW) radar. This article also introduces a lightweight DCNN model, DIAT-RadHARNet, designed for those human suspicious activity classification. To reduce the computation cost and to improve the generalization ability, DIAT-RadHARNet is designed with four design principles: depthwise separable convolutions, channel weighting (CHW) based on the importance, different size filters in the depthwise part, and operating different size kernels on the same input tensor. The network has 213793 parameters with a total of 55 layers. Our extensive experimental analysis demonstrates that the DIAT-RadHARNet model efficiently classifies the activities with 99.22% accuracy, giving minimal false positive and false negative outcomes. The time complexity of the proposed DCNN model observed during the testing phase is 0.35 s. The same accuracy and time complexity are obtained even at adverse weather conditions, low-lighting environments, and long-range operations.
MoreTranslated text
Key words
Convolutional neural network,deep convolution neural network (DCNN)-based classification,human suspicious activity,micro-Doppler (m-Doppler) signatures,X -hand continuous wave (CW) radar
AI Read Science
Must-Reading Tree
Example
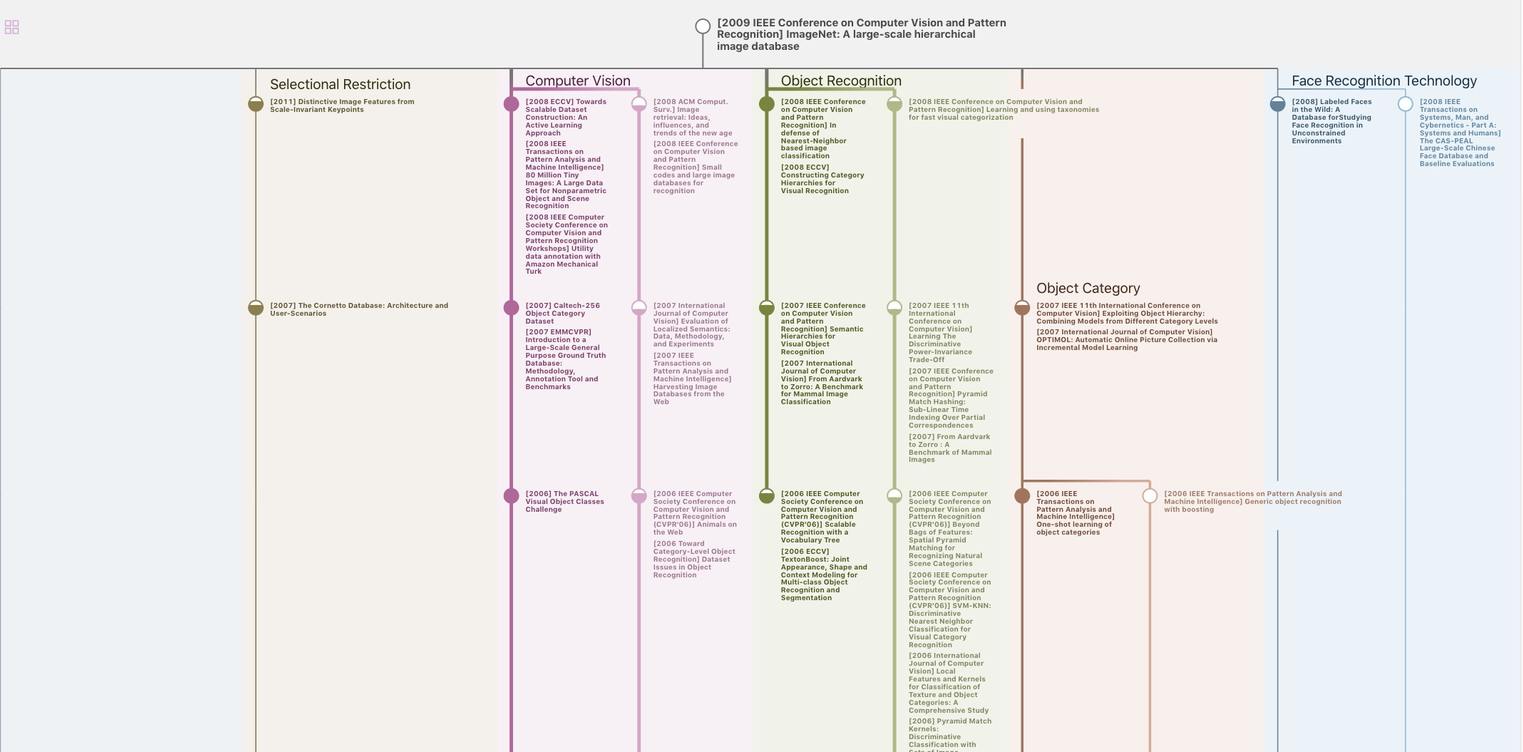
Generate MRT to find the research sequence of this paper
Chat Paper
Summary is being generated by the instructions you defined