Probabilistic Selection Approaches in Decomposition-Based Evolutionary Algorithms for Offline Data-Driven Multiobjective Optimization
IEEE Transactions on Evolutionary Computation(2022)
摘要
In offline data-driven multiobjective optimization, no new data are available during the optimization process. Approximation models, also known as surrogates, are built using the provided offline data. A multiobjective evolutionary algorithm can be utilized to find solutions by using these surrogates. The accuracy of the approximated solutions depends on the surrogates and approximations typically involve uncertainties. In this article, we propose probabilistic selection approaches that utilize the uncertainty information of the Kriging models (as surrogates) to improve the solution process in offline data-driven multiobjective optimization. These approaches are designed for decomposition-based multiobjective evolutionary algorithms and can, thus, handle a large number of objectives. The proposed approaches were tested on distance-based visualizable test problems and the DTLZ suite. The proposed approaches produced solutions with a greater hypervolume, and a lower root mean squared error compared to generic approaches and a transfer learning approach that do not use uncertainty information.
更多查看译文
关键词
Gaussian processes,kernel density estimation,Kriging,metamodeling,Pareto optimality,surrogate
AI 理解论文
溯源树
样例
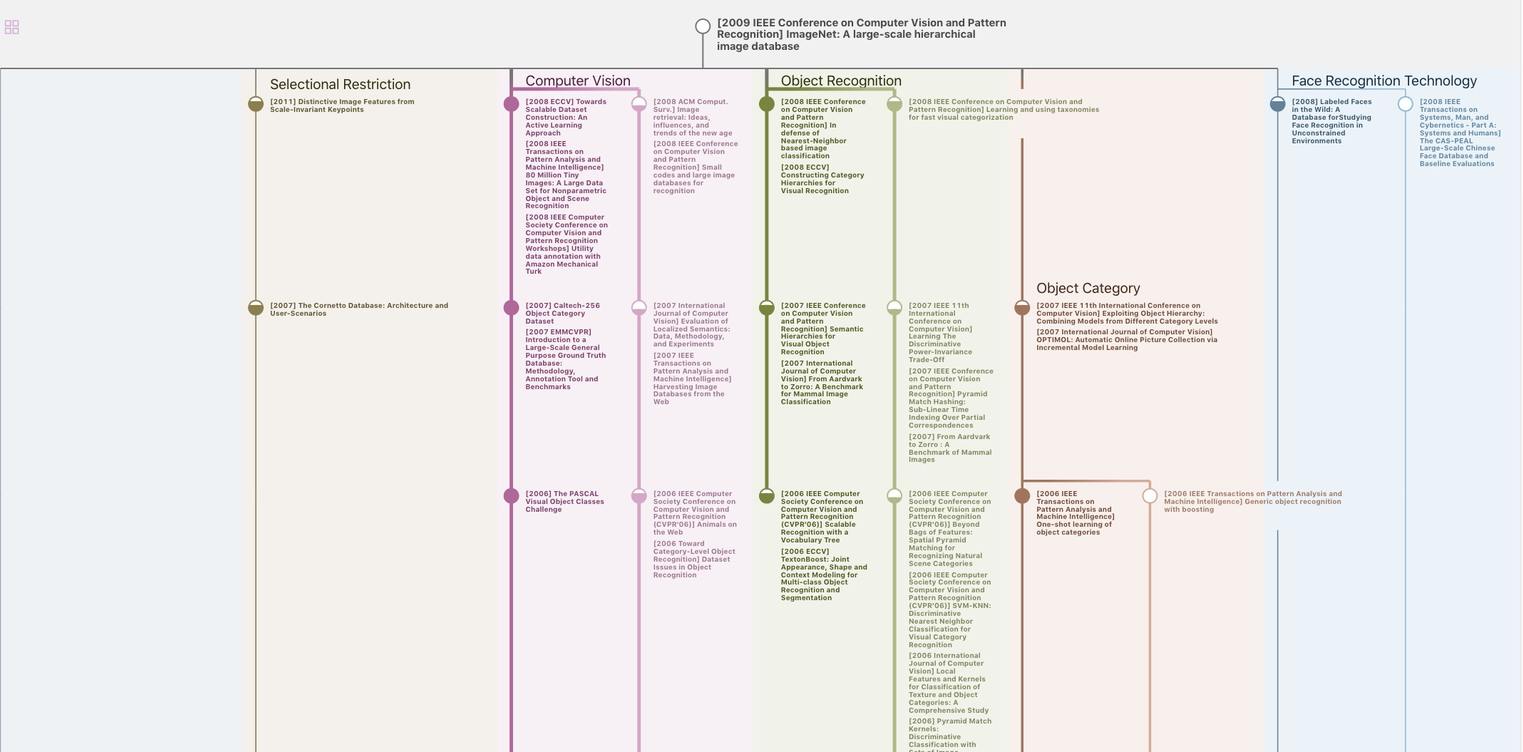
生成溯源树,研究论文发展脉络
Chat Paper
正在生成论文摘要