Self-Paced Knowledge Distillation for Real-Time Image Guided Depth Completion
IEEE SIGNAL PROCESSING LETTERS(2022)
摘要
Image guided depth completion aims to generate a dense depth map from a sparse one with the guidance of a color image. Previous high-accuracy methods often rely on complex networks that are large in size and expensive in computational cost, making them inapplicable to real-time platforms. In this letter, we propose a self-paced knowledge distillation method, which obtains a lightweight but accurate depth completion model via distilling knowledge from a complex teacher network. Specifically, by taking advantage of the easy-to-hard learning curriculum in deep networks, we first design a groundtruth-free hard-pixel mining module to tell hard and noisy pixels in the teacher's output. Then, we design two self-paced distillation losses, which gradually introduce hard pixels to distill the depth and structure knowledge from the teacher to the compact student network. Experiments on the KITTI benchmark show that the proposed method can improve the original student model by a considerable margin. The distilled compact and real-time student model outperforms all previous lightweight networks, mitigating the performance gap with state-of-the-art high-accuracy but complex models.
更多查看译文
关键词
Knowledge engineering,Predictive models,Training,Task analysis,Real-time systems,Color,Loss measurement,Image guided depth completion,knowledge distillation,self-paced learning
AI 理解论文
溯源树
样例
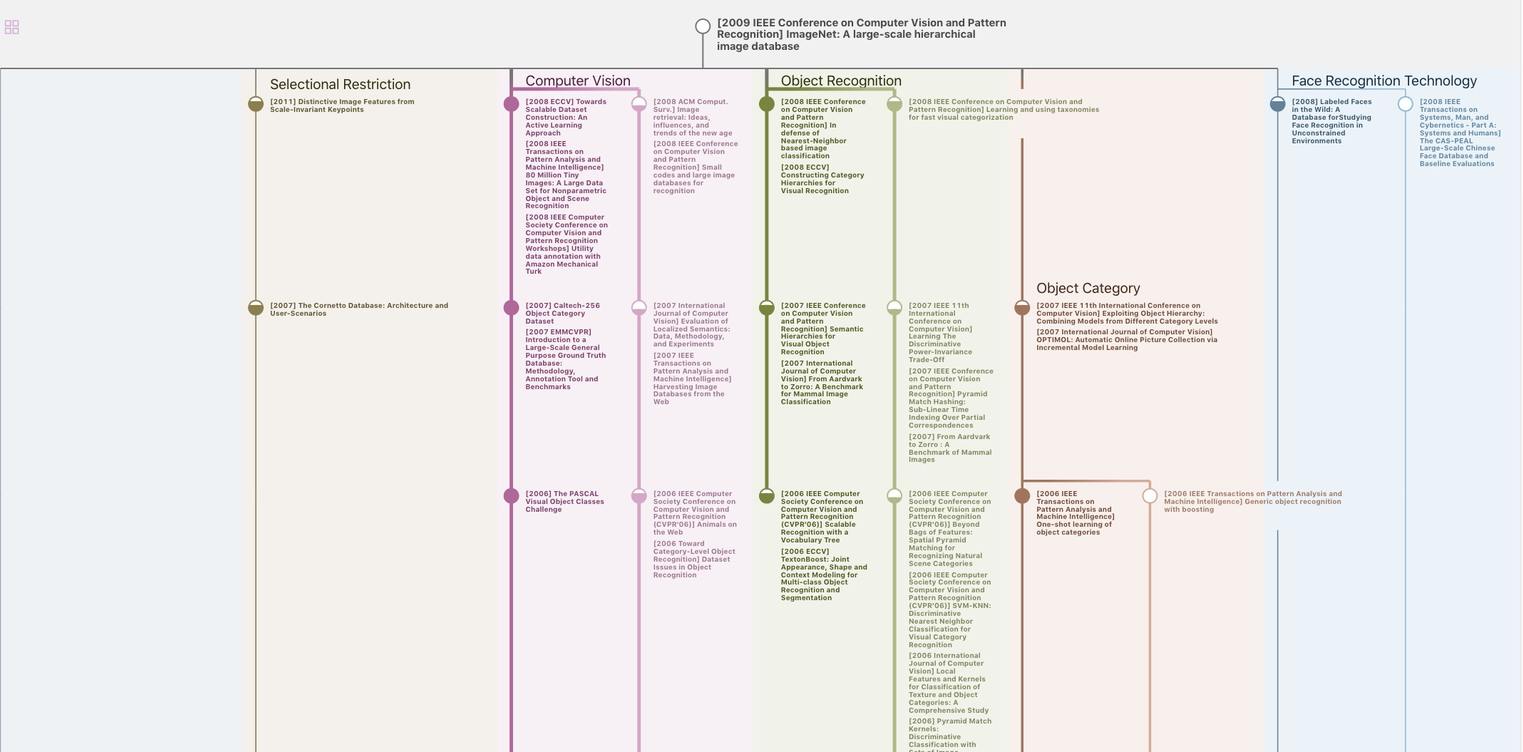
生成溯源树,研究论文发展脉络
Chat Paper
正在生成论文摘要