Efficient Training of the Memristive Deep Belief Net Immune to Non-Idealities of the Synaptic Devices
ADVANCED INTELLIGENT SYSTEMS(2022)
摘要
The tunability of conductance states of various emerging nonvolatile memristive devices emulates the plasticity of biological synapses, making it promising in the hardware realization of large-scale neuromorphic systems. The inference of the neural network can be greatly accelerated by the vector-matrix multiplication (VMM) performed within a crossbar array of memristive devices in one step. Nevertheless, the implementation of the VMM needs complex peripheral circuits, and the complexity further increases as non-idealities of memristive devices prevent precise conductance tuning (especially for the online training) and largely degrade the performance of the deep neural networks (DNNs). Herein, an efficient online training method of the memristive deep belief net (DBN) is presented. The proposed memristive DBN uses stochastically binarized activations, reducing the complexity of peripheral circuits, and uses the contrastive divergence (CD)-based gradient descent learning algorithm. The analog VMM and digital CD are performed separately in a mixed-signal hardware arrangement, making the memristive DBN highly immune to non-idealities of synaptic devices. The number of write operations on memristive devices is reduced by two orders of magnitude. The recognition accuracy of 95-97% can be achieved for the MNIST dataset using pulsed synaptic behaviors of various memristive synaptic devices.
更多查看译文
关键词
contrastive divergence, deep belief net, memristive synapse, non-ideality, restricted Boltzmann machine
AI 理解论文
溯源树
样例
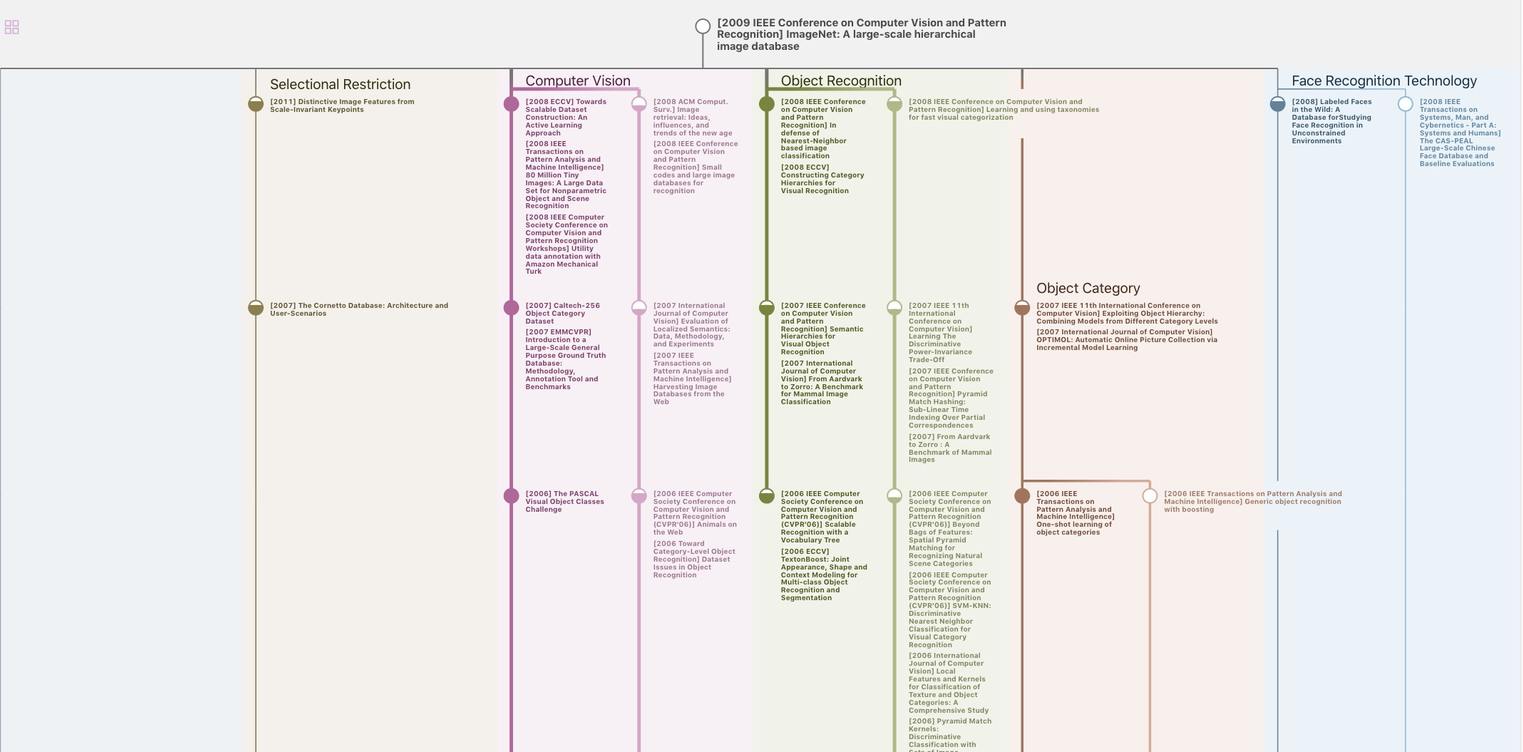
生成溯源树,研究论文发展脉络
Chat Paper
正在生成论文摘要